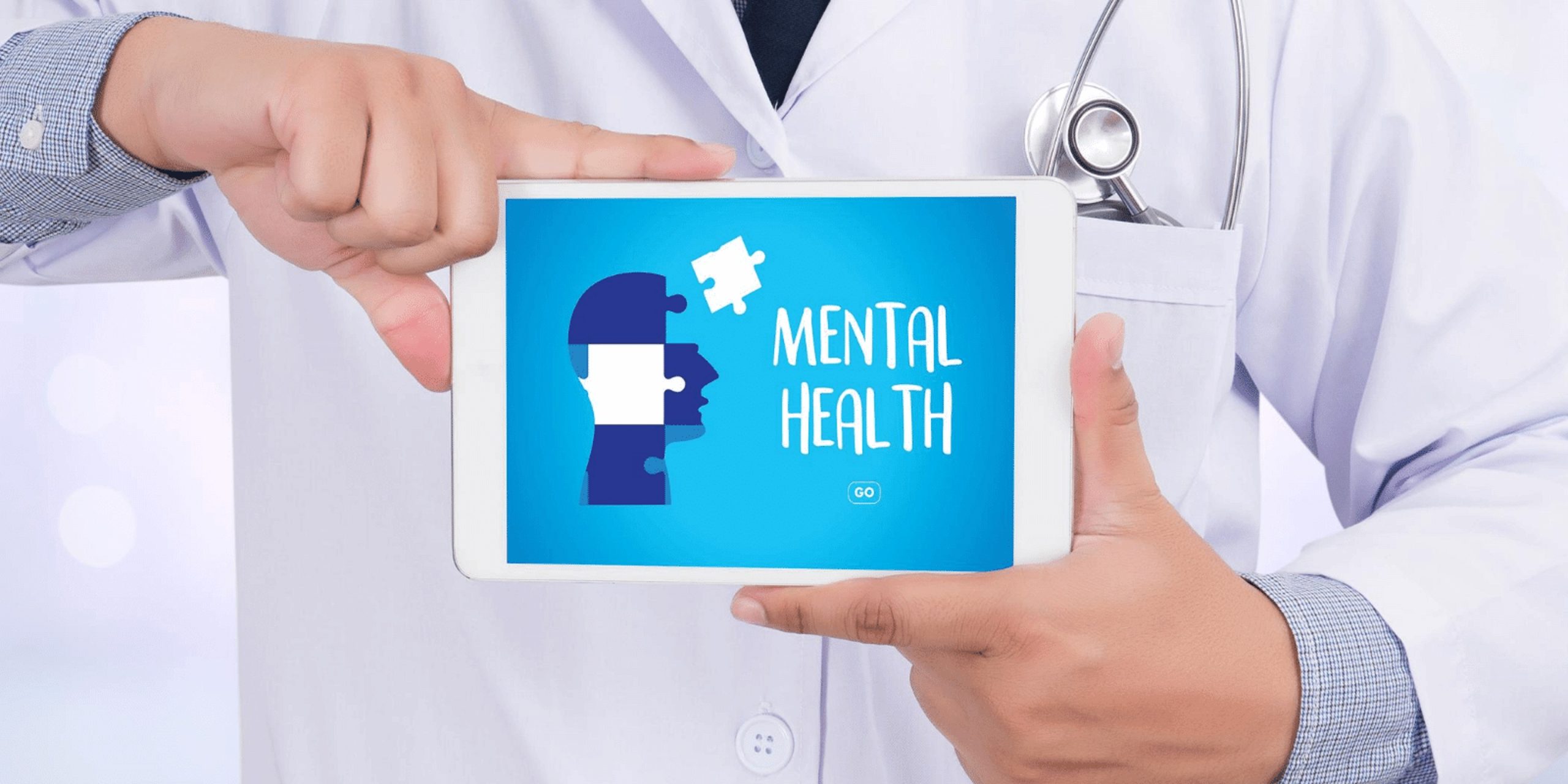
Artificial intelligence (AI) is increasingly recognized for its potential in mental healthcare, aiding in diagnosis, personalized treatment, and patient compliance. AI chatbots provide therapy and support, wearables interpret bodily signals, and machine learning analyzes data for early detection and prediction of mental health conditions. Challenges include addressing bias and the subjective nature of mental health diagnosis. Careful implementation and collaboration between AI researchers and healthcare professionals can lead to improved outcomes and relief for those affected by mental health issues.
Artificial intelligence (AI) is increasingly recognized as a valuable tool in the field of mental healthcare, benefiting clinicians, therapists, and researchers. In this article, we explore how AI can aid in diagnosing conditions, developing therapies, and facilitating personalized approaches and treatments.
Over the past three years, the Covid-19 pandemic has led to a surge in individuals seeking help for mental health issues like depression and anxiety. Tragically, suicide has become the fourth leading cause of death among 15 to 29-year-olds worldwide. Consequently, healthcare and therapeutic services face mounting pressure, making access to them more challenging. In this context, could smart technology powered by machine learning offer a solution, reducing the reliance on medication or confinement in mental health hospitals?
Let’s delve into some of the ways this revolutionary technology is already transforming lives and improving patient outcomes for various mental health conditions.
AI Therapists
Imagine sharing your deepest feelings and thoughts with a robot rather than a human. Chatbots are increasingly used to provide advice and communication channels for mental health patients during their treatment. These chatbots assist with symptom management, identify trigger keywords, and connect patients with human mental healthcare professionals when necessary.
For example, Woebot is a therapeutic chatbot that adapts to users’ personalities, offering guidance through various therapies and talking exercises commonly used to help patients cope with diverse conditions. Another chatbot, Tess, provides free, on-demand emotional support 24/7 and helps individuals deal with anxiety and panic attacks whenever they arise.
Wearables
Some AI mental health solutions go beyond traditional apps by functioning as wearables that interpret bodily signals through sensors. These wearables intervene and offer assistance when needed, instead of waiting for user interaction.
One such wearable is Biobeat, which collects data on sleeping patterns, physical activity, heart rate, and rhythm variations. This information is used to assess the user’s mood and cognitive state. By comparing the data with anonymized and aggregated information from other users, predictive warnings can be generated when intervention may be necessary. Users can then adjust their behavior or seek assistance from healthcare services proactively.
Diagnosing and Predicting Outcomes
AI can analyze a range of data sources, such as medical records, behavioral data, voice recordings, and social media data, using machine learning to identify early warning signs of mental health problems before they escalate.
For instance, an IBM and University of California review revealed that machine learning achieved high accuracy in predicting and classifying mental health problems like suicidal thoughts, depression, and schizophrenia. The reviewed studies utilized data from electronic health records, brain imaging, smartphone and video monitoring systems, and social media.
Moreover, researchers at Vanderbilt University Medical Center demonstrated that machine learning could predict with 80% accuracy whether a person was at risk of suicide using hospital admission, demographic, and clinical data.
The Alan Turing Institute is undertaking a project to predict mental health issues by leveraging large-scale datasets from individuals without apparent symptoms. This effort aims to identify those likely to develop symptoms in their lifetimes.
AI has also been applied to predict patients’ likelihood of responding positively to cognitive behavioral therapy (CBT), potentially reducing the need for medication. Deep learning techniques have been used to validate the effectiveness of CBT as a treatment method, leading to improved patient outcomes, as highlighted in research published in JAMA Psychology.
Improving Patient Compliance
Ensuring patient compliance with prescribed treatments, including medication adherence and therapy sessions, is a significant challenge in mental health care. AI can help predict when a patient might become non-compliant and issue reminders or notify healthcare providers for manual intervention. This can be achieved through chatbots, SMS, automated calls, and emails. Algorithms can also identify behavioral patterns or triggering events that may lead to non-compliance, enabling healthcare workers to develop strategies to overcome these obstacles.
Personalized Treat
One exciting area of research involves using AI to develop personalized treatments for various mental health conditions. AI can monitor symptoms and treatment responses to provide insights for adjusting individualized treatment plans. For example, a research project at the University of California, Davis focused on creating personalized treatment plans for children with schizophrenia using computer vision analysis of brain images. These AI algorithms must be understandable by doctors who may not be AI professionals, ensuring transparency and collaboration.
Challenges in Using AI for Mental Health Treatment
While AI holds great promise for predicting mental health issues, creating personalized treatment plans, and ensuring compliance, it also presents challenges that require collaboration between AI researchers and healthcare professionals.
One challenge is AI bias, where inaccuracies or imbalances in training data can lead to unreliable predictions or perpetuate social prejudice. For example, if mental health issues are more likely to go undiagnosed in certain ethnic groups due to limited healthcare access, algorithms trained on biased data may also struggle to accurately diagnose those issues. AI engineers and mental healthcare professionals must work together to implement checks and balances that counteract biases or eliminate biased data to ensure fair and accurate outcomes.
Additionally, diagnosing mental health conditions often relies on subjective judgment from clinicians, and the same subjectivity applies to machine diagnoses. Decisions are based on patients’ self-reported feelings and experiences rather than objective medical tests, which introduces uncertainty. Careful monitoring and follow-up are necessary to achieve the best outcomes for patients.
A recent World Health Organization report highlighted significant gaps in our understanding of AI’s application in mental healthcare, flaws in processing data in existing AI healthcare applications, and inadequate evaluation of bias risks.
AI has the potential to make a positive impact on mental healthcare by predicting issues, personalizing treatment plans, and ensuring compliance. However, progress must be made cautiously, thoroughly assessing models and methodologies for bias risks before deploying them in situations that affect human lives. As our understanding and implementation of AI solutions improve, we can build a stronger case for wider adoption of these groundbreaking technologies. Ultimately, this can lead to improved outcomes for conditions that are currently challenging to treat, alleviating the devastating impact of mental health problems on patients’ lives.