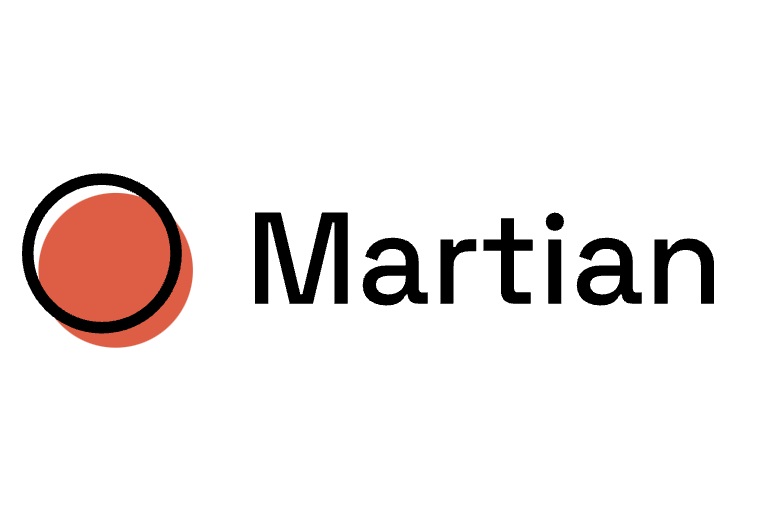
Martian, founded by UPenn AI researchers, pioneers interpretability-aligned AI products amidst an industry shift favoring competitive models over fundamental research. Securing $9 million in funding, Martian develops a groundbreaking “model router.” This router intelligently directs prompts to optimal Large Language Models (LLMs) based on performance and cost metrics, potentially reducing expenses associated with high-end models. Martian’s innovation lies in estimating model performance sans execution, facilitating seamless model switches for diverse applications. Early adoption among major enterprises indicates promising effectiveness, underlining Martian’s potential to revolutionize AI efficiency strategies.
In a climate prioritizing competitive AI models over foundational research, UPenn AI researchers Upadhyay and Ginsberg unveil Martian—a company focused on interpretability-driven AI products. Their $9 million funding propels Martian’s innovation: a pioneering “model router.” This router strategically allocates prompts to suitable Large Language Models (LLMs), envisioning significant cost reductions by circumventing exclusive reliance on high-end models. Martian’s approach relies on estimating model performance without execution, enabling dynamic model switches tailored to specific tasks. Despite competitors like Credal, early uptake by major enterprises highlights Martian’s potential in enhancing AI efficiency strategies.
Shriyash Upadhyay and Etan Ginsberg, AI researchers from the University of Pennsylvania, observe a trend in AI companies emphasizing competitive AI models over fundamental research. Their insight reveals a prioritization of staying ahead in the market rather than exploring the basics.
Identifying this concern during their LLM research at UPenn, Upadhyay and Ginsberg stress the challenge of making AI research profitable. Their solution? Founding Martian, a company focused on interpretability-aligned products, aiming to advance interpretability research over capability research. This approach aims to bolster profound research outcomes.
Emerging from stealth with $9 million in funding from prominent investors like NEA, Prosus Ventures, Carya Venture Partners, and General Catalyst, Martian channels resources into product development, internal model operations research, and team expansion.
Martian’s flagship offering, a “model router,” automatically directs prompts to the optimal Large Language Model (LLM), such as GPT-4, based on criteria like uptime, skillset, and cost-to-performance ratio. Unlike the current practice of funneling all requests to a single LLM, Martian’s router dynamically selects the most suitable model for specific tasks within an application.
This approach demonstrates potential cost savings, a critical aspect given the considerable expense of high-end models. Permutable.ai’s CEO disclosed a yearly cost of over $1 million to process 2 million articles daily using premium OpenAI models.
Martian’s innovation lies in its ability to estimate model performance without execution, efficiently switching between models to ensure optimal cost and performance balance. It intelligently selects cost-effective models unless higher-end models are essential, indexing new models seamlessly into applications.
While Martian isn’t the only player in automatic model-switching tech (Credal also offers a similar tool), its success hinges on competitive pricing and efficacy in high-stakes commercial contexts. Despite this, early adoption among “multibillion-dollar” enterprises hints at its potential effectiveness.
Upadhyay and Ginsberg emphasize the complexity of building an effective model router, attributing their breakthrough to a deep understanding of these models’ fundamental workings—a milestone they’ve pioneered.
Martian’s innovation in model routing, driven by a profound comprehension of AI models, heralds a paradigm shift in AI optimization strategies, promising cost efficiency and performance enhancements for diverse commercial applications.
Martian, initiated by UPenn researchers, leads the charge in interpretability-focused AI products amid a landscape favoring competitive AI models. Bolstered by $9 million funding, Martian’s revolutionary “model router” promises efficiency gains. By selectively directing prompts to optimal Large Language Models (LLMs), it anticipates substantial cost savings by circumventing costly high-end model dependency. Martian’s innovative approach of estimating model performance facilitates seamless model switches, a feat appreciated by early adopters among major enterprises. This underscores Martian’s potential to transform AI efficiency strategies, heralding a shift towards cost-effective and performance-driven AI applications.