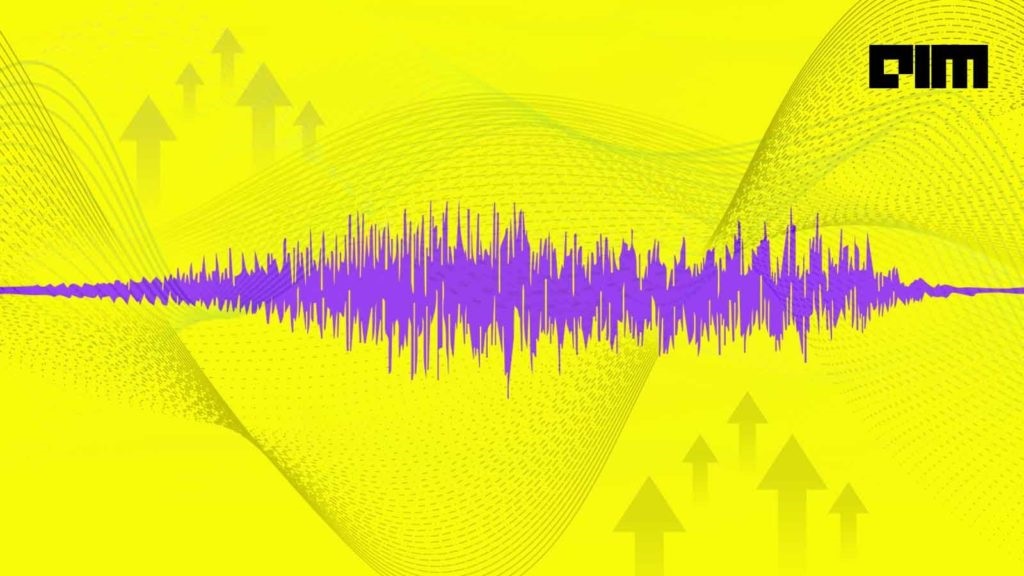
Amazon researchers unveil groundbreaking text-to-speech (TTS) model, BASE TTS, exhibiting emergent abilities in handling linguistic complexities. Through extensive training and experimentation, the model demonstrates proficiency in articulating challenging text excerpts, surpassing its predecessors. This breakthrough paves the way for enhanced naturalness and versatility in TTS applications, offering promising prospects for accessibility and beyond.
In a landmark achievement, researchers at Amazon have unveiled the most significant text-to-speech (TTS) model to date, boasting “emergent” qualities that greatly enhance its capacity to naturally articulate even the most complex sentences. This breakthrough holds promise for steering the technology out of the uncanny valley, marking a significant stride forward in the field.
While the evolution and refinement of these models were anticipated, the researchers set out with the specific goal of witnessing a leap in capabilities akin to the advancements observed in language models upon reaching a critical size threshold. Interestingly, beyond a certain point, Large Language Models (LLMs) exhibit heightened robustness and versatility, demonstrating proficiency in tasks beyond their training.
It’s crucial to note that this surge in capability does not imply the attainment of sentience by these models. Rather, their performance in certain conversational AI tasks experiences an exponential improvement past a certain scale. The team at Amazon AGI, transparent in their aim to achieve Artificial General Intelligence (AGI), speculated that similar advancements might occur as text-to-speech models scaled up, and their research validates this hypothesis.
Dubbed “Big Adaptive Streamable TTS with Emergent Abilities” or BASE TTS, the new model represents a significant leap forward. Utilizing 100,000 hours of public domain speech data, predominantly in English (with additional samples in German, Dutch, and Spanish), the largest iteration of the model incorporates a staggering 980 million parameters, making it the largest model of its kind. Additionally, the researchers trained models with 400 million and 150 million parameters, providing a comparative spectrum to pinpoint the emergence of these remarkable capabilities.
Surprisingly, it was the medium-sized model that demonstrated the desired leap in capability, primarily evident in its emergent abilities rather than a substantial improvement in ordinary speech quality. Examples of challenging text excerpts highlighted in the research paper include compound nouns, emotional expressions, foreign words, paralinguistics, punctuations, questions, and syntactic complexities, all of which the BASE TTS model tackled with notable proficiency.
While text-to-speech engines traditionally struggle with such linguistic complexities, the BASE TTS model exhibited remarkable competence, surpassing its contemporaries such as Tortoise and VALL-E. Demonstrations on the model’s dedicated website showcased its natural rendition of challenging texts, although these examples were curated by the researchers.
The success of the BASE TTS models underscores the pivotal role played by model size and training data in enabling the model to handle linguistic intricacies effectively. However, it’s essential to acknowledge that this remains an experimental endeavor, not yet ready for commercial deployment. Future research endeavors will focus on identifying the inflection point for emergent ability and optimizing the training and deployment processes accordingly.
An additional noteworthy feature of the BASE TTS model is its “streamable” nature, allowing it to generate speech incrementally at a relatively low bitrate. Furthermore, efforts have been made to encapsulate speech metadata such as emotionality and prosody in a separate, low-bandwidth stream, offering enhanced versatility.