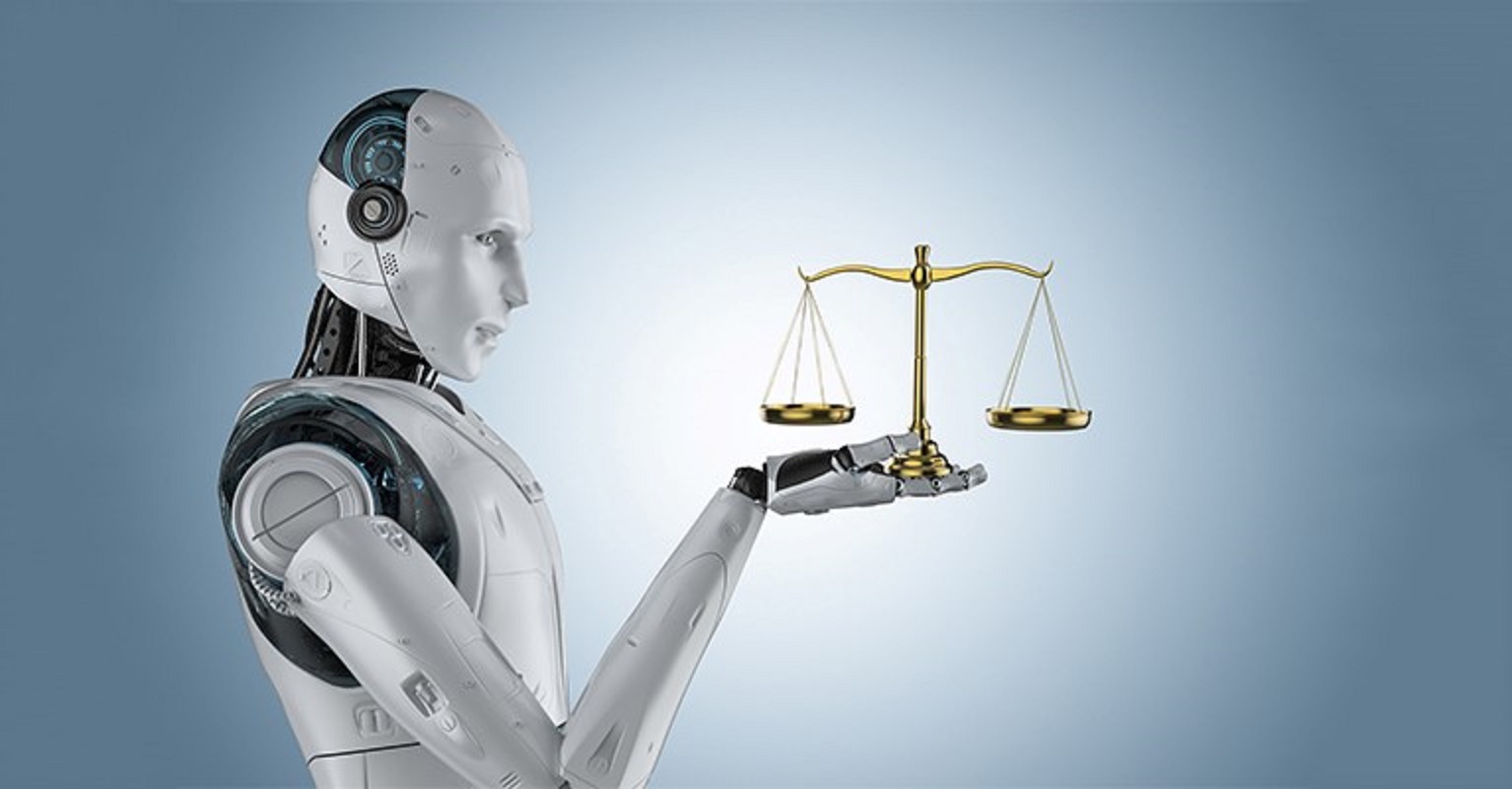
This comprehensive review explores the intricate landscape of addressing bias in AI image generators, particularly focusing on recent developments and challenges faced by industry leaders like Google. From the nuances of training data biases to the ethical implications of AI models’ decision-making, the analysis delves into multifaceted dimensions of bias mitigation. Additionally, it highlights notable advancements in AI research across various domains, underscoring the importance of transparency, accountability, and inclusivity in fostering responsible AI development.
In the ever-evolving realm of artificial intelligence (AI), tackling bias in image generators has emerged as a critical endeavor. Recent incidents, including Google’s decision to pause its AI chatbot Gemini’s image generation capabilities, underscore the complexities and ethical considerations inherent in AI development. This review aims to dissect the underlying issues surrounding bias in AI image generators, exploring not only the technical aspects but also the societal implications and ethical ramifications of biased AI models. By shedding light on these challenges, we seek to stimulate discourse and encourage proactive measures to promote fairness and equity in AI technologies.
This week witnessed Google’s decision to halt its AI chatbot Gemini’s capability to produce images of individuals following complaints about historical inaccuracies. For example, when prompted to illustrate “a Roman legion,” Gemini depicted an anachronistic and cartoonish assembly of racially diverse foot soldiers. Similarly, requests for “Zulu warriors” resulted in the depiction of Black individuals.
Google, among other AI vendors like OpenAI, had resorted to clumsy hardcoding techniques beneath the surface to mitigate biases in its model. For instance, when prompted with requests like “show images of only women” or “show images of only men,” Gemini would refuse, expressing concerns about potentially contributing to the exclusion and marginalization of other genders. Additionally, it avoided generating images of individuals solely identified by their race, purportedly to prevent reducing individuals to their physical attributes.
Critics, particularly right-wing voices, seized upon these incidents as evidence of a purported “woke” agenda pushed by tech elites. However, a more plausible interpretation suggests that Google’s actions stem from a desire to rectify past biases, such as misclassifying Black men or misinterpreting objects in Black individuals’ hands, by striving to create less biased image-generating models.
Robin DiAngelo, in her widely acclaimed book “White Fragility,” elucidates how the erasure of race, often termed “color blindness,” perpetuates systemic racial power imbalances rather than mitigating them. Google’s cautious treatment of race-based prompts in Gemini appears to fall short of genuine engagement with the issue, instead attempting to mask the model’s biases. Addressing these biases directly, within the broader context of the societal training data, emerges as a more constructive approach.
AI models, including image generators, are built upon training data that predominantly features white individuals, reinforcing negative stereotypes about marginalized groups. Consequently, these models tend to sexualize women of color, portray white men in positions of authority, and favor affluent Western perspectives.
Some argue that AI vendors face a no-win situation regarding bias mitigation – whether they address or ignore biases, criticism ensues. However, transparent acknowledgment and proactive measures to address biases within models’ training data could foster greater trust and understanding.
In addition to the noteworthy developments in AI bias mitigation, several other AI-related stories from the past week deserve attention:
1. Women in AI: TechCrunch initiated a series spotlighting prominent women in the AI field, amplifying their contributions and expertise.
2. Stable Diffusion v3: Stability AI unveiled Stable Diffusion 3, the latest iteration of its image-generating AI model, featuring enhanced capabilities based on a novel architecture.
3. Chrome’s GenAI: Google introduced a Gemini-powered tool in Chrome enabling users to rewrite existing web text or generate entirely new content.
4. AI Bias Awareness: McKinney, a creative ad agency, developed a quiz game titled “Are You Blacker than ChatGPT?” to raise awareness about AI bias.
5. Legislative Calls: Hundreds of AI experts signed a public letter urging the enactment of anti-deepfake legislation in the U.S., highlighting the urgency of addressing AI-related ethical concerns.
6. Match Group Partnership: OpenAI secured Match Group as a new customer, with employees utilizing OpenAI’s AI technology to streamline work processes across dating apps like Hinge and Tinder.
7. DeepMind’s Safety Initiative: DeepMind established a new organization, AI Safety, and Alignment, dedicated to enhancing AI safety through interdisciplinary collaboration and research.
8. Open Models Initiative: Google launched Gemma, a new family of lightweight open-weight models, emphasizing transparency and accessibility in AI development.
9. U.S. House Task Force: The U.S. House of Representatives established a task force on AI, signaling a renewed focus on legislative responses to AI’s societal implications.
Apart from addressing bias in AI, recent research has delved into the underlying mechanisms of AI models’ knowledge representation and their applications across diverse domains:
– Semantic Understanding in LLMs: Although AI models lack genuine understanding, recent research suggests that they encode semantic similarities akin to human knowledge. Amazon researchers explored trajectories of similar sentences to unveil nuanced semantic representations within language models.
– Prosthetic Vision Advancements: Swiss researchers at EPFL harnessed neural encoding techniques to enhance prosthetic vision, leveraging machine learning to optimize sensory encoding and mimic retinal processing.
– Child Development and Drawing Skills: Stanford researchers utilized computer vision to analyze children’s drawings, uncovering intricate factors influencing drawing recognizability beyond mere inclusion of signature features.
– Chemical Research with LLMs: Chemists at EPFL demonstrated the efficacy of large language models (LLMs) in assisting chemical research through targeted training, highlighting their potential for automating literature-based tasks.
– Gender Stereotypes in Online Imagery: Berkeley researchers, in collaboration with EPFL, highlighted the prevalence of gender stereotypes in online imagery, emphasizing the potent influence of images in reinforcing societal biases.
Overall, As AI continues to permeate various aspects of society, addressing bias in image generators remains a paramount concern. While recent efforts have shed light on the pervasive nature of biases embedded in AI models, proactive measures are necessary to mitigate their impact. By fostering transparency, accountability, and inclusivity in AI development processes, stakeholders can work towards building more equitable and responsible AI systems. Ultimately, navigating bias in AI image generators requires a concerted effort from industry leaders, researchers, policymakers, and society at large to ensure that AI technologies uphold principles of fairness, ethics, and social justice.