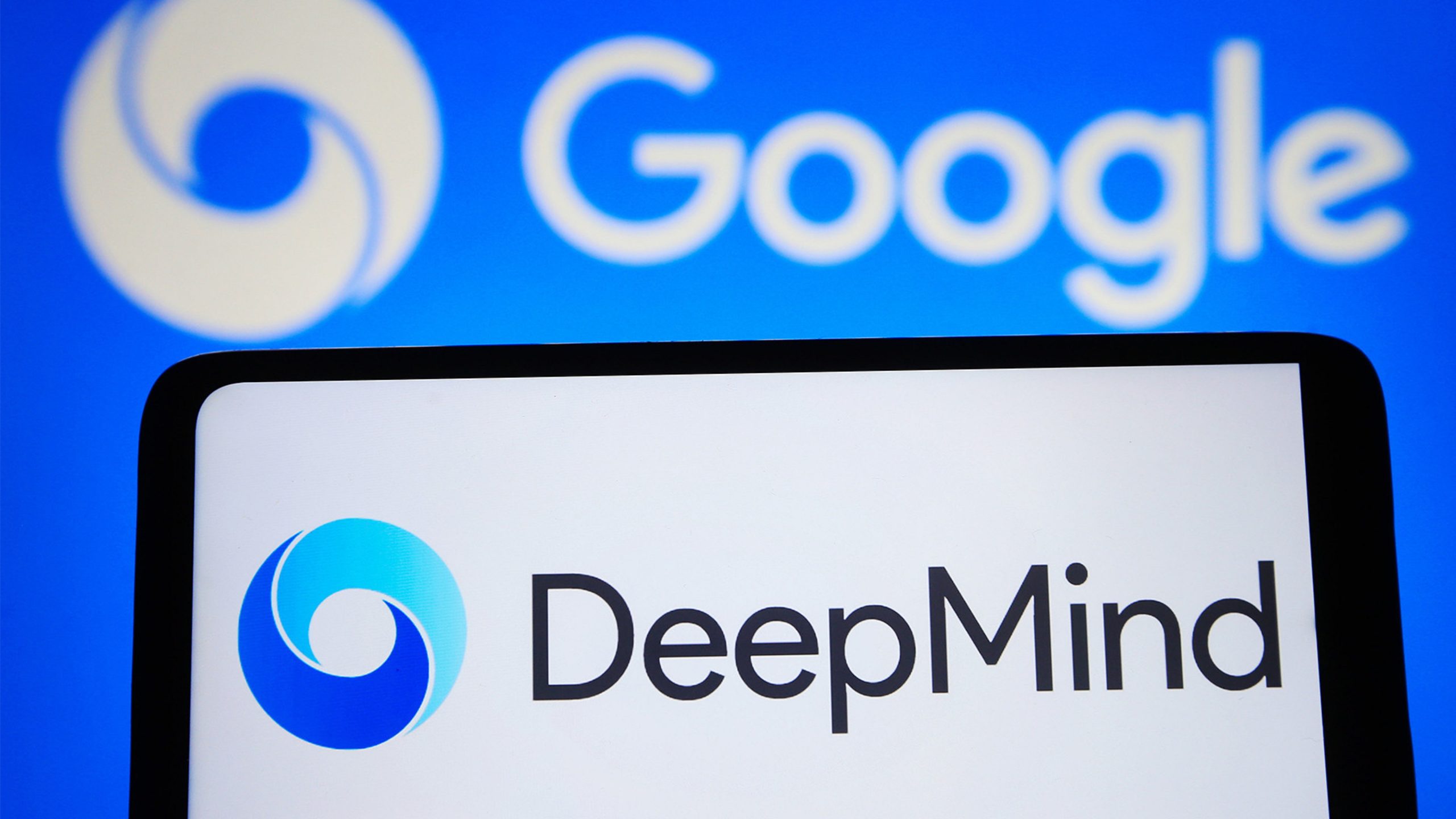
Google DeepMind is developing an algorithm called Gemini that aims to surpass OpenAI’s ChatGPT. Inspired by the success of AlphaGo, Gemini combines the strengths of AlphaGo with the language capabilities of large models. It aims to enhance problem-solving and planning abilities. Google’s response to the competitive landscape includes the deployment of the Bard chatbot and generative AI in various products. DeepMind’s expertise in reinforcement learning and potential collaborations with external researchers aim to address the risks associated with AI development.
The CEO of Google DeepMind, Demis Hassabis, has revealed that the company is developing a new algorithm called Gemini, which he believes will surpass OpenAI’s ChatGPT in terms of capabilities. The inspiration for Gemini comes from DeepMind’s groundbreaking AI program, AlphaGo, which famously defeated a world champion Go player in 2016. While Gemini shares similarities with OpenAI’s GPT-4, it incorporates techniques used in AlphaGo to enhance its abilities, such as problem-solving and planning.
Hassabis describes Gemini as a combination of AlphaGo’s strengths and the advanced language capabilities of large models like GPT-4. The development of Gemini is still ongoing and is expected to take several months and involve significant financial investment. Its completion could play a crucial role in Google’s response to the competitive landscape, especially against ChatGPT and other generative AI technologies.
Google has been cautious about deploying AI products but has recently accelerated its efforts following the success of ChatGPT. They have introduced a chatbot called Bard and integrated generative AI into various products, including their search engine. In April, DeepMind merged with Google’s primary AI lab, Brain, forming Google DeepMind, to leverage the strengths and innovations of both organizations.
Hassabis, who has experience navigating the fast-paced world of AI development, believes that the combination of reinforcement learning techniques and large language models holds great potential. DeepMind’s expertise in reinforcement learning, coupled with ideas from other areas of AI such as robotics and neuroscience, could contribute to enhancing the capabilities of large language models like Gemini.
However, Hassabis acknowledges the need to address the risks associated with advanced AI development. He emphasizes the importance of researching evaluation tests to assess the capabilities and controllability of new AI models. DeepMind may also make its systems more accessible to outside scientists to encourage collaboration and ensure that concerns about exclusive access to AI research are addressed.
Hassabis acknowledges the uncertainties surrounding the potential dangers of AI but emphasizes the urgency of developing safeguards as progress in the field continues. He remains optimistic about the potential benefits of AI for humanity but emphasizes the need for responsible and thoughtful development to mitigate risks.