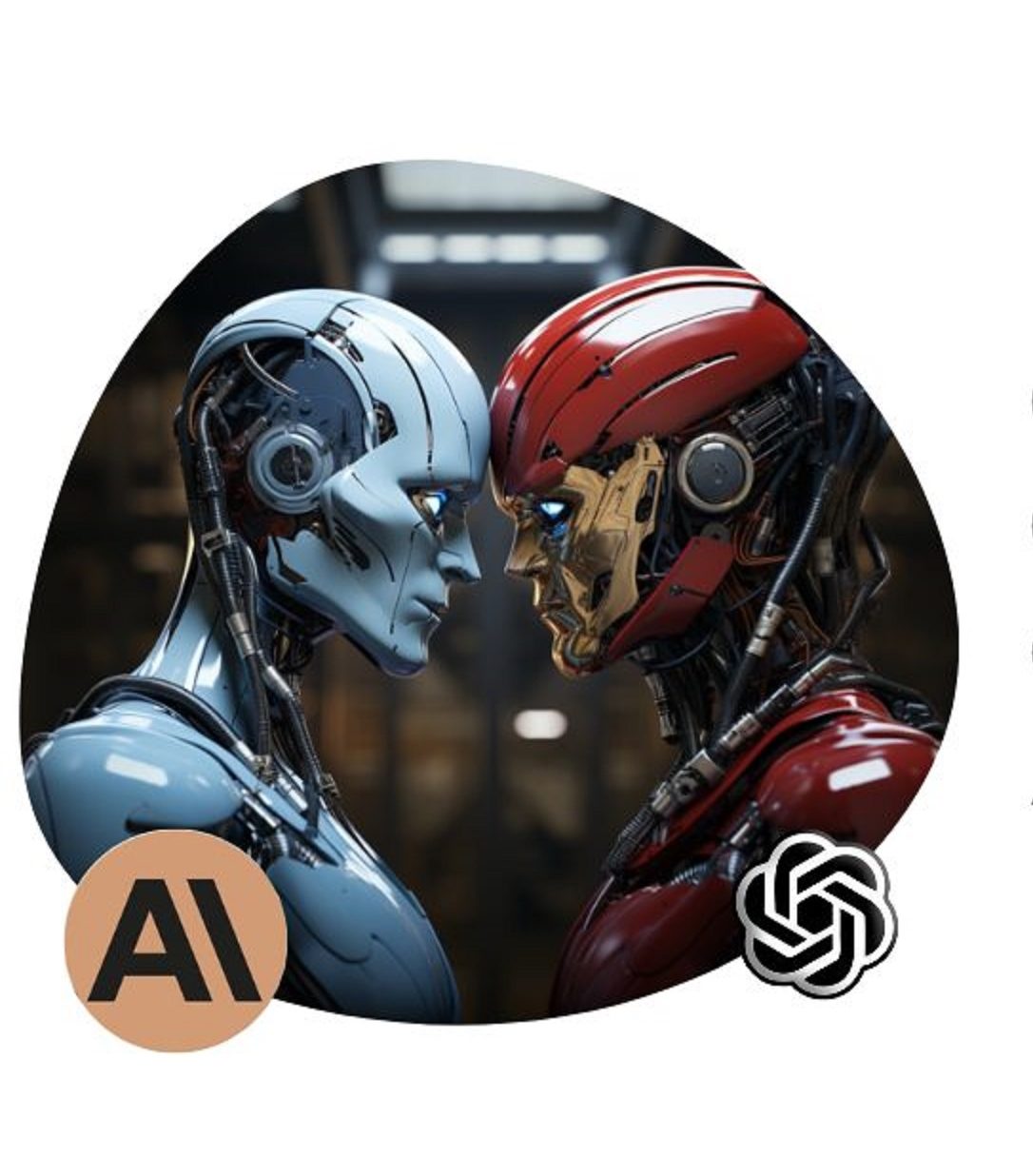
Leading AI models, including Meta, OpenAI, Anthropic, and Cohere A.I., exhibit varying creativity in generating information. A recent Arthur AI report assesses their tendencies. GPT-4 excels in math, Meta’s Llama 2 is moderate, Anthropic’s Claude 2 understands its limits, and Cohere AI generates imaginative content with inaccuracies. Amid AI misinformation concerns, the study explores hallucination rates, with GPT-4 showing improvement over GPT-3.5. Cohere contests findings. Understanding models’ real-world performance is crucial, per Arthur’s CEO, Adam Wenchel.
The leading AI models in the tech industry, namely Meta, OpenAI, Anthropic, and Cohere A.I., exhibit varying degrees of creativity in generating information. A recent report from Arthur AI, a machine learning monitoring platform, delves into their performance and tendencies.
In terms of distinct characteristics, if these AI models were awarded superlatives, GPT-4 by OpenAI, supported by Microsoft, would excel in mathematical tasks. Meanwhile, Meta’s Llama 2 would be positioned as moderately capable, Anthropic’s Claude 2 would demonstrate an astute understanding of its limitations, and Cohere AI would stand out for its propensity to generate imaginative content, often accompanied by confidently inaccurate answers.
The Arthur AI research arrives at a critical juncture when the proliferation of misleading information originating from AI systems is more contentious than ever, particularly in light of the upcoming 2024 U.S. presidential election and the surge in generative AI technologies.
This report is unique in its comprehensive evaluation of hallucination rates within AI models, going beyond a mere placement on an LLM leaderboard. According to Adam Wenchel, Co-founder and CEO of Arthur, this research marks the first attempt to thoroughly analyze the frequency of hallucinations. These hallucinations occur when large language models (LLMs) fabricate entirely fictional information, mimicking factual statements. For instance, ChatGPT was found to have cited inaccurate cases in a New York federal court filing, leading to potential sanctions for the involved New York attorneys.
The Arthur AI researchers conducted experiments involving these AI models across various categories such as combinatorial mathematics, U.S. presidents, and Moroccan political leaders. These inquiries were structured to necessitate multifaceted reasoning, thereby provoking the LLMs to make errors. The findings revealed that GPT-4 outperformed all other models, demonstrating fewer instances of hallucination compared to its predecessor, GPT-3.5. Notably, GPT-4 exhibited a 33% to 50% reduction in hallucination rates in mathematical queries, contingent on the category.
In contrast, Meta’s Llama 2 displayed a higher overall rate of hallucination than both GPT-4 and Anthropic’s Claude 2. GPT-4 secured the top position in mathematical questions, closely followed by Claude 2. However, Claude 2 emerged as the most accurate in U.S. presidents’ knowledge, pushing GPT-4 to second place. GPT-4 retained its supremacy in Moroccan political knowledge, while Claude 2 and Llama 2 tended to refrain from responding to such queries.
The researchers conducted a secondary experiment to gauge how much these AI models hedged their responses with cautious phrases to mitigate potential risks. GPT-4 exhibited a 50% relative increase in hedging compared to GPT-3.5. This increase was perceived as contributing to higher frustration levels among users. In contrast, Cohere’s AI model did not engage in hedging in any of its responses. Claude 2 excelled in terms of “self-awareness,” displaying an accurate understanding of its knowledge boundaries and answering solely within the scope of its training data.
Cohere, through a spokesperson, contested the results, asserting the efficacy of its retrieval automated generation technology in providing verifiable citations to authenticate information sources.
Overall, Adam Wenchel emphasized that users and businesses should test these AI models on their specific requirements. He underscored the importance of understanding how these models perform within the context of their actual applications rather than relying solely on benchmarks. He stressed that a comprehensive grasp of the models’ real-world performance is essential given their multifaceted utilization.