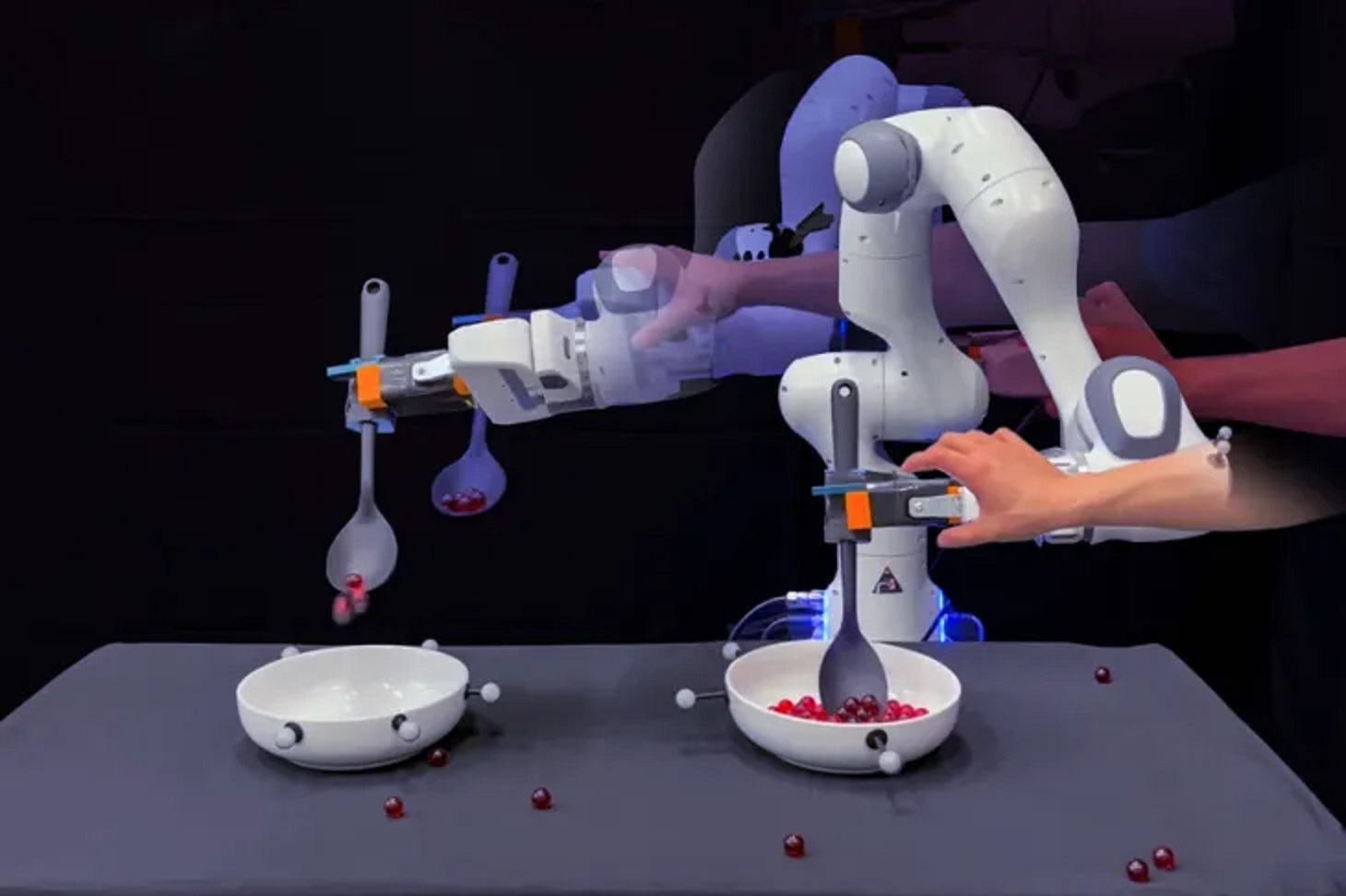
Innovative research from MIT presented at the International Conference on Learning Representations (ICLR) unveils a groundbreaking approach to error recovery in home robotics. By harnessing the power of Large Language Models (LLMs), robots gain the ability to interpret natural language instructions and autonomously address errors in real-time. Through meticulous demonstrations and deliberate sabotage, researchers demonstrate the transformative potential of LLMs in enabling robots to navigate through unforeseen challenges with agility and resilience. This paradigm shift marks a significant milestone in the evolution of home robotics, paving the way for intelligent, autonomous companions that seamlessly integrate into household environments.
Unlocking the true potential of home robotics hinges upon the ability to navigate and recover from errors autonomously. Despite advancements in various aspects, such as pricing and practicality, the challenge of error recovery persists. However, a beacon of hope emerges through the integration of Large Language Models (LLMs). These sophisticated models offer a pathway to infuse a sense of “common sense” into robotic systems, enabling them to adapt and recover from unforeseen challenges without human intervention. As we delve into the realm of LLM-powered robotics, we embark on a journey toward a future where household companions seamlessly navigate through dynamic environments with agility and resilience.
Understanding the Challenges in Home Robotics
In the realm of home robotics, success stories akin to the Roomba vacuum cleaner are few and far between. Despite advancements in pricing, practicality, form factor, and mapping technologies, these robotic companions often struggle to meet consumer expectations. One persistent challenge lies in the realm of error recovery. When inevitable mistakes occur, how can these machines autonomously rectify errors without human intervention?
Bridging the Gap: From Industrial Solutions to Consumer-Friendly Approaches
While error recovery has long been a focal point in industrial robotictowdtowardessing such issues at the consumer level present unique challenges. Unlike big corporations with ample resources to tackle problems as they arise, expecting consumers to possess programming skills or hire technical assistance for every glitch is impractical. However, emerging research from MIT offers a promising solution, leveraging the power of Large Language Models (LLMs) to empower home robots.
Unveiling the Power of Large Language Models in Robotics
Presenting their findings at the International Conference on Learning Representations (ICLR), MIT researchers shed light on a groundbreaking approach to error recovery in robotics. Their study introduces a novel method aimed at infusing a sense of “common sense” into the error correction process.
In essence, the research emphasizes that robots possess a remarkable ability to mimic actions. However, without explicit programming to adapt to unforeseen circumstances, robots often falter when faced with unexpected challenges, necessitating a restart of the entire task. This is particularly problematic in dynamic environments like households, where the slightest changes can disrupt a robot’s functionality.
Overcoming Limitations through Innovative Techniques
While imitation learning has gained traction in the realm of home robotics, it struggles to account for the myriad environmental variations that can impede seamless operation. Traditional approaches treat demonstrations as continuous actions, overlooking the need to address smaller subsets of actions within the larger task. Herein lies the crux of the problem that the new research endeavors to solve.
Redefining Error Recovery: A Paradigm Shift in Robotics
The innovative approach proposed by MIT researchers involves leveraging LLMs to streamline the error recovery process. By breaking down demonstrations into smaller, more manageable subsets, robots equipped with LLMs gain the ability to autonomously identify and address errors without requiring manual intervention.
Empowering Robots with Natural Language Understanding
Central to this approach is the ability of LLMs to interpret natural language instructions, effectively bridging the gap between human demonstrations and robotic actions. Rather than relying on manual labeling and assignment of subactions, LLMs empower robots to understand the sequential steps of a task in a human-like manner.
A Case Study: Marbles and Bowls
To illustrate the efficacy of their method, researchers conducted experiments involving a robot tasked with scooping marbles and pouring them into an empty bowl. While seemingly straightforward for humans, this task presents a series of intricate subtasks for robots. Through meticulous demonstrations and deliberate sabotage, researchers showcased the robot’s ability to self-correct errors in real time, thereby eliminating the need for human intervention.
Transforming Failure into Opportunity
In essence, this novel approach represents a paradigm shift in the field of home robotics, transforming failures into opportunities for autonomous learning and improvement. By seamlessly integrating LLMs into the error recovery process, robots can navigate through unforeseen challenges with agility and resilience, ultimately enhancing their adaptability in dynamic environments.
As we reflect on the transformative impact of Large Language Models on home robotics, it becomes evident that we stand at the threshold of a new era in human-robot interaction. The integration of LLMs represents more than just a technological advancement; it signifies a paradigm shift towards autonomy and adaptability in robotic systems. By empowering robots with the ability to understand and interpret natural language instructions, we pave the way for a future where errors are not setbacks but opportunities for learning and growth. As we continue to explore the vast potential of LLM-powered robotics, we move closer to realizing a vision where household companions seamlessly navigate through dynamic environments, enriching the lives of individuals and families alike.