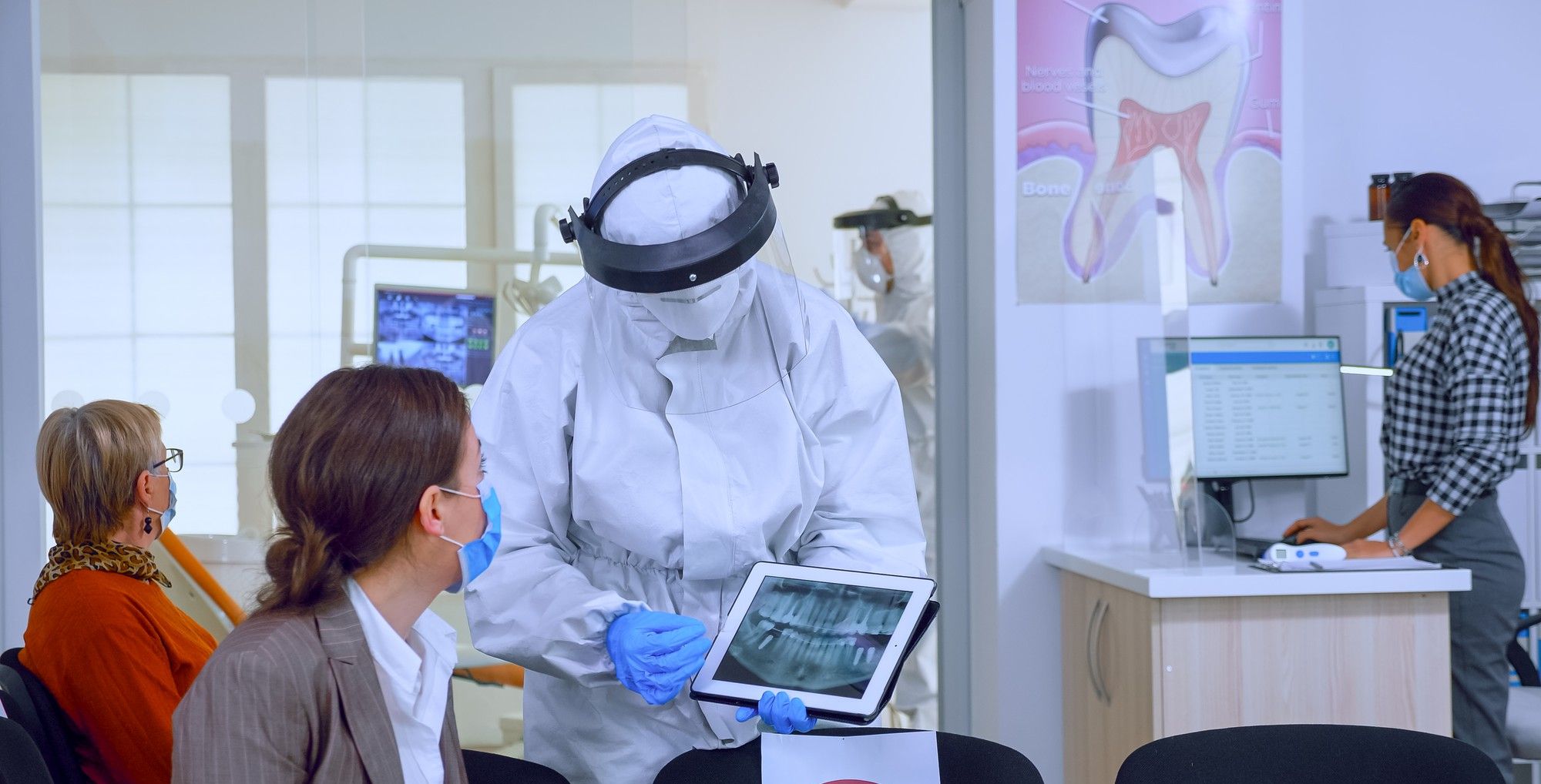
A new study published in Nature Communications used machine learning to analyze electronic health records from two patient populations in the United States. The study found that long COVID risk and symptoms can vary significantly across different populations. The researchers believe that these differences may be due to several factors, including the demographics of the two populations, the severity of the COVID-19 pandemic in each area, and the different healthcare systems in place. The study’s findings highlight the need for further research into long COVID and the potential use of machine learning to better understand the disease.
A recent study demonstrated that the risk and signs of protracted COVID can range dramatically among various populations. This study was published in Nature Communications. In the study, electronic health records (EHRs) from two sizable patient groups in the United States were analyzed using machine learning by researchers at Weill Cornell Medical.
The researchers found that patients in New York City were more likely to experience long COVID than patients in Florida. They also found that patients in New York City were more likely to experience a wider range of symptoms, including hair loss, fatigue, abnormal heartbeat, blood clots in the lung, chest pain, sores in the small intestine and stomach, and dementia.
The researchers believe that these differences may be due to several factors, including the demographics of the two populations, the severity of the COVID-19 pandemic in each area, and the different healthcare systems in place.
The study’s findings highlight the need for further research into long COVID. The researchers believe that machine learning can be a valuable tool for understanding the disease and developing new treatments.
Other research using machine learning to study long COVID
Other institutions are also using machine learning (ML) to investigate long COVID. In January 2023, researchers from the University of California, Berkeley (UC Berkeley) used ML to identify six subtypes of long COVID.
The UC Berkeley researchers used ML software and electronic health record (EHR) data to find common symptoms and identify condition subtypes of long COVID. They computationally modeled PASC phenotype data found in EHRs and assessed phenotypic similarities among patients. Using these, the research team then clustered patients into groups based on patient-patient similarity scores.
These efforts yielded six clusters of PASC patients with distinct profiles of phenotypic abnormalities. The six subtypes of long COVID identified by the UC Berkeley researchers are:
- Subtype 1: This subtype is characterized by fatigue, shortness of breath, and cognitive impairment.
- Subtype 2: This subtype is characterized by chest pain, heart palpitations, and abnormal heart rhythms.
- Subtype 3: This subtype is characterized by muscle pain, joint pain, and headaches.
- Subtype 4: This subtype is characterized by gastrointestinal problems, such as diarrhea, nausea, and vomiting.
- Subtype 5: This subtype is characterized by neurological problems, such as dizziness, numbness, and tingling.
- Subtype 6: This subtype is characterized by skin problems, such as hair loss and rashes.
The identification of these six subtypes of long COVID is a significant step forward in our understanding of the disease. It is now possible to better characterize the symptoms and severity of long COVID, which can help to improve diagnosis and treatment. Additionally, the identification of these subtypes may lead to the development of more targeted treatments for long COVID.
The research conducted by the UC Berkeley researchers is just one example of the many ways that ML is being used to investigate long COVID. As ML technology continues to develop, we will likely learn even more about this complex disease. This knowledge will help us to develop better treatments and improve the lives of people affected by long COVID.