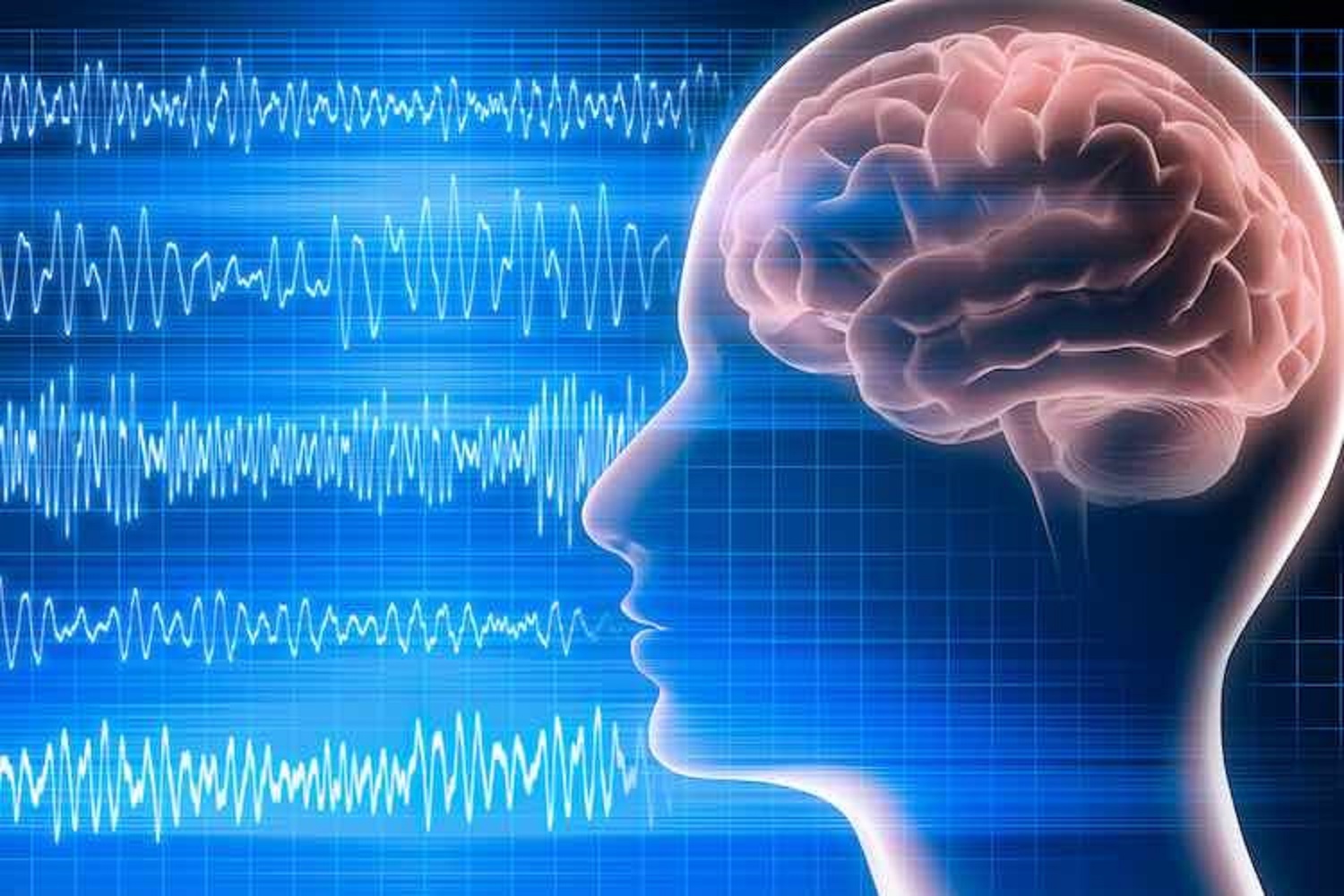
A New Head-Worn device developed by researchers at the University of California, San Diego, uses machine learning and magnetoencephalography (MEG) to distinguish between hand gestures. The device could potentially be used to help people with paralysis or amputated limbs control devices with their minds. The device has an accuracy level of over 85% in distinguishing between hand gestures.
Researchers from the University of California, San Diego, have developed a head-worn device that uses machine learning and magnetoencephalography (MEG) to distinguish between hand gestures. The device could potentially be used to help people with paralysis or amputated limbs control devices with their minds.
MEG is a noninvasive brain imaging technique that uses magnetic fields to measure electrical activity in the brain. A 306-sensor array included in the gadget created by UC San Diego researchers can detect magnetic fields that result from neuronal electric currents between brain neurons.
To evaluate the device’s effectiveness, the researchers ran a trial. This trial, which consisted of 12 willing volunteers, required each subject to wear the device while doing one of the three “rock, paper, scissors” hand gestures.
The data was interpreted through a deep-learning model known as MEG-RPSnet. When reviewing results, researchers found that the device had an accuracy level that exceeded 85 percent in distinguishing between hand gestures.
An area for improvement, however, is related to the number of sensors in the MEG helmets. Given that measurements from half of the sampled regions generated results with a 2 percent to 3 percent loss of accuracy, researchers noted the potential need to eliminate sensors.
The development of this device is a significant step forward in the development of non-invasive brain-computer interfaces. With further research and development, this technology could one day be used to help people with paralysis or amputated limbs regain a greater degree of independence.
Other Applications of Wearable Devices in Clinical Cases
As the use of wearable devices continues to grow, researchers are discovering new ways to apply them to clinical cases. For instance, in a study published in April 2022, study results indicated that wearable sensors used to monitor heart rate, skin temperature, and respiratory rate showed potential for detecting and managing COVID-19.
In this study, researchers analyzed various databases related to the use of wearable devices. The Fitbit was the most frequently used gadget, followed by the WHOOP strap, the Apple watch, and the Empatica E4, according to a final sample evaluation of 12 trials.
Of the 12 studies, nine used machine-learning algorithms to explore physiological data, eight of which indicated an existing correlation between COVID-19 and an elevated heart rate. Further, 75 percent of studies that reviewed respiratory rates indicated that they increased with COVID-19 and that 76 percent of participants experienced elevated temperatures just before the onset of symptoms.
These findings suggest that wearable devices could be used to develop early warning systems for COVID-19. Further research is needed to confirm these findings and develop practical applications for wearable devices in the clinical setting.