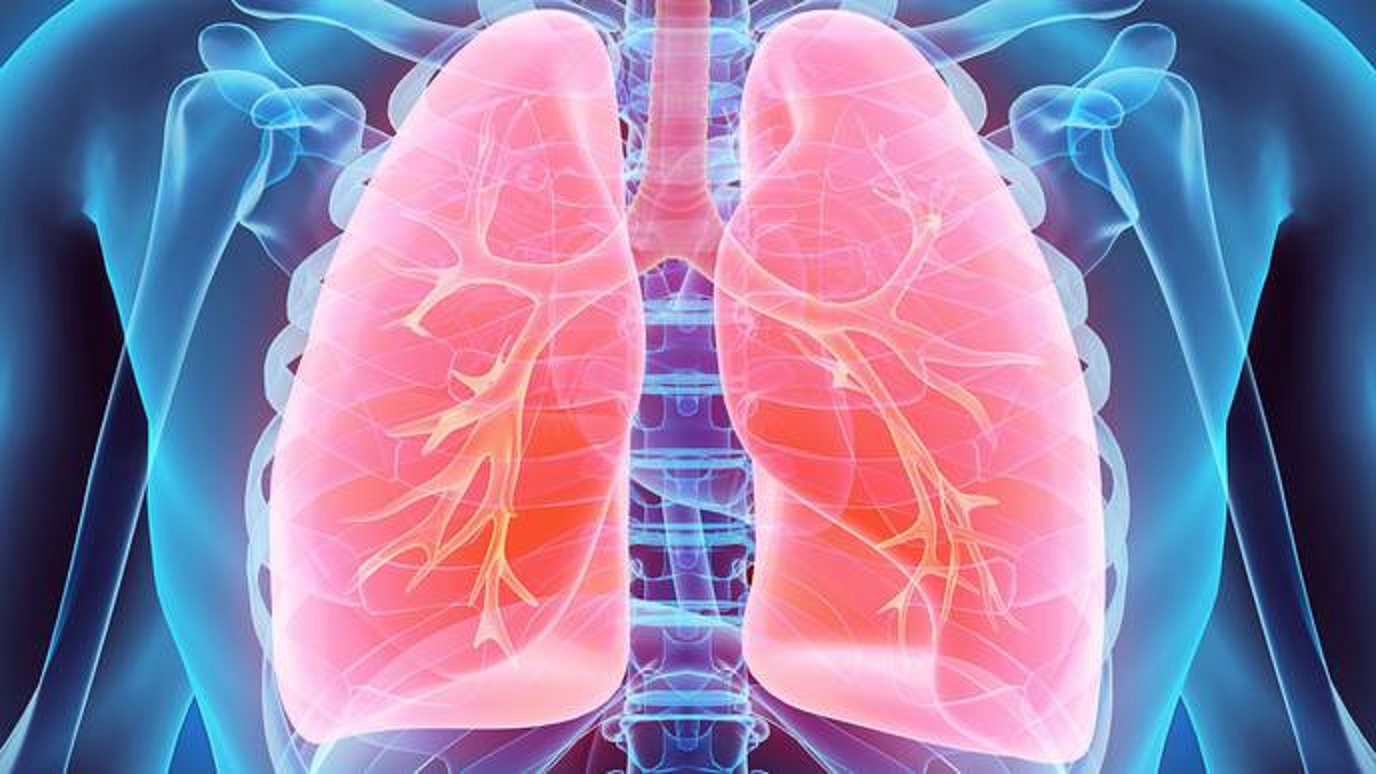
A Machine-Learning tool accurately triages patients with respiratory symptoms before primary care visits, according to a study. By categorizing patients into risk groups, unnecessary interventions like chest X-rays can be reduced for low-risk individuals, improving care and alleviating clinician workloads. The ML model was trained using clinical features and medical records. The research highlights the potential of AI and ML in enhancing triage processes, including the use of deep learning models for acute chest pain diagnosis.
A recent study published in The Annals of Family Medicine has demonstrated the effectiveness of a machine learning (ML)-based clinical decision support tool in accurately categorizing patients with respiratory symptoms before their primary care visits. This innovative approach shows promise for improving patient triage and reducing clinician workloads.
Respiratory symptoms are commonly reported in primary care settings, and while some may resolve on their own, others could indicate more severe illnesses or conditions. Therefore, effective triage of patients with respiratory symptoms is crucial.
However, the challenges of rising healthcare costs and increased clinician workloads make triage a complex task. To address this issue, researchers developed an ML model capable of triaging patients with respiratory symptoms before their primary care appointments.
The ML model was trained using clinical features and relevant questions typically asked before a primary care visit. Additionally, clinical text notes from 1,500 medical records of patients in Reykjavik, Iceland, diagnosed with various respiratory conditions, were extracted. These conditions included the common cold, influenza, bacterial pneumonia, acute bronchitis, chronic obstructive pulmonary disease (COPD), and asthma.
The information extracted from the clinical notes encompassed data on prescription rates, imaging referrals, and the clinician’s assessment of each patient’s symptoms.
Based on these data, patients were categorized into five diagnostic groups and further sorted into ten risk subgroups according to their probability of being diagnosed with a lower respiratory tract infection.
The study findings revealed that patients in risk subgroups one through five were generally younger and had lower rates of antibiotic prescription, re-evaluation in primary and emergency care, chest X-ray referrals, and chest X-rays indicating pneumonia.
Interestingly, none of the patients in these subgroups had chest X-rays showing signs of pneumonia, nor did their clinicians diagnose them with pneumonia.
On the other hand, patients in risk subgroups six through ten were considered high-risk, exhibiting higher rates of symptoms related to lung inflammation.
The researchers concluded that the ML model could effectively reduce unnecessary interventions, such as chest X-rays, for low-risk patients. This would help minimize clinically insignificant findings and alleviate burdens on clinicians without increasing costs for patients.
This research represents a growing area of study that explores how artificial intelligence (AI) and machine learning (ML) can enhance the triage process.
In a related study, researchers recently highlighted the potential of a deep learning (DL) model for improving care for patients presenting with acute chest pain in hospitals. The DL model utilizes chest X-rays to identify individuals with symptoms requiring immediate medical attention, as acute chest pain can be indicative of serious conditions like acute coronary syndrome, pulmonary embolism, or aortic dissection.
Current clinical tests for detecting and diagnosing acute chest pain syndrome often lack specificity, prompting clinicians to resort to pulmonary and cardiovascular diagnostic imaging. However, these tests can introduce inefficiencies in chest pain triage.
Researchers aim to streamline and enhance this process through the application of deep learning and other advanced technologies.