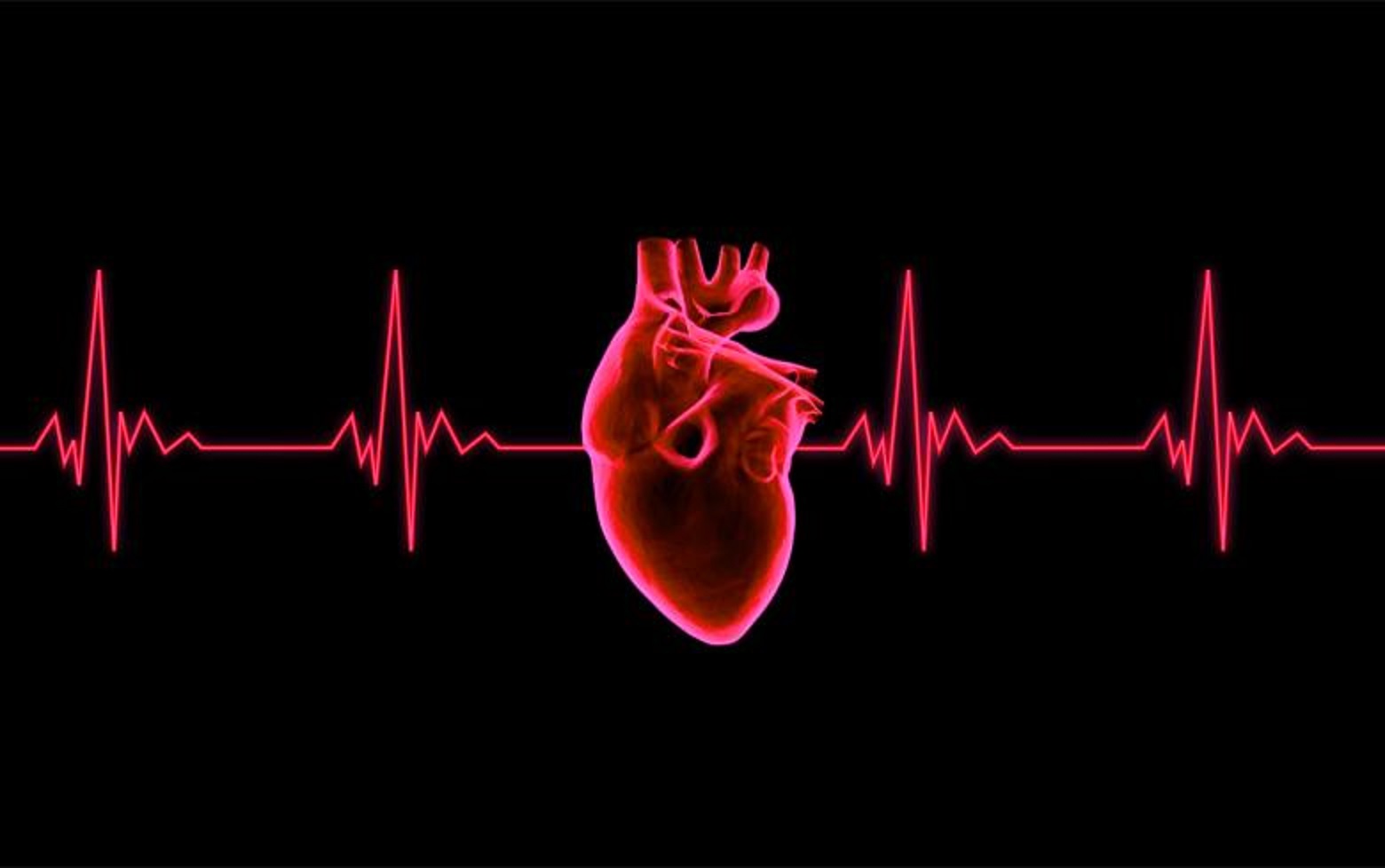
A deep learning model has been created and validated to precisely estimate cardiac function and find valvular diseases using chest radiographs. Various conditions, including left ventricular ejection fraction and tricuspid regurgitant velocity, were classified by the model with high accuracy rates. It was also emphasised that it could be useful in places where there is little access to echocardiography experts. Additionally, another study addressed the problem of unclear ECGs in chest pain triage by introducing a machine-learning solution that uses electrocardiogram readings to detect and classify heart attacks.
Researchers have successfully developed and validated a deep learning (DL) model capable of estimating cardiac function and identifying valvular diseases using chest radiographs. The study, recently published in The Lancet Digital Health, highlights the potential of DL models in leveraging chest radiographs for cardiovascular examination, particularly in the classification of valvular diseases and cardiac function.
Traditionally, chest radiographs have been widely accessible and commonly used for cardiovascular assessment, but their effectiveness in classifying valvular diseases and cardiac function has been uncertain. To address this knowledge gap, the researchers embarked on developing and validating a DL model that could simultaneously detect various conditions, including left ventricular ejection fraction, tricuspid regurgitant velocity, mitral regurgitation, aortic stenosis, aortic regurgitation, mitral stenosis, tricuspid regurgitation, pulmonary regurgitation, and inferior vena cava dilation using chest radiographs.
The research team collected chest radiographs and associated echocardiogram data from four medical institutions in Japan. Three of these sites were utilized for training, validation, and internal testing of the DL model, while the fourth site’s data was used for external testing.
The training dataset consisted of 22,551 chest radiographs paired with 22,551 echocardiograms from 16,946 patients. The external testing dataset included 3,311 chest radiographs from 2,617 patients. The DL model’s performance was assessed based on several metrics, including the area under the receiver operating characteristic curve (AUC), sensitivity, specificity, and accuracy.
The model demonstrated favorable results with AUCs of 0.92 for classifying left ventricular ejection fraction, 0.85 for classifying tricuspid regurgitant velocity, 0.89 for classifying mitral regurgitation, 0.83 for classifying aortic stenosis, 0.83 for classifying aortic regurgitation, 0.86 for classifying mitral stenosis, 0.92 for classifying tricuspid regurgitation, 0.86 for classifying pulmonary regurgitation, and 0.85 for classifying inferior vena cava dilation. The model achieved corresponding accuracy rates of 86 percent, 75 percent, 85 percent, 73 percent, 68 percent, 90 percent, 83 percent, 69 percent, and 86 percent, respectively.
These findings support the conclusion that the DL model accurately estimates valvular heart diseases and cardiac functions using digital chest radiographs. Furthermore, the model’s ability to classify echocardiography values swiftly and with minimal system requirements suggests its potential applicability in regions with limited access to echocardiography specialists.
This research aligns with the growing interest in utilizing artificial intelligence-based tools to enhance cardiovascular care. In a separate study from the University of Pittsburgh Medical Center (UPMC), researchers recently introduced a machine learning (ML) solution that employs electrocardiogram (ECG) readings to detect and categorize heart attacks. By identifying subtle patterns in ECGs that may elude clinicians, this model effectively classifies patients according to their risk of heart attack. The research team reported a reclassification rate of one in three chest pain patients using this method, addressing the issue of ambiguous ECGs in chest pain triage, which can lead to delayed critical medical intervention.