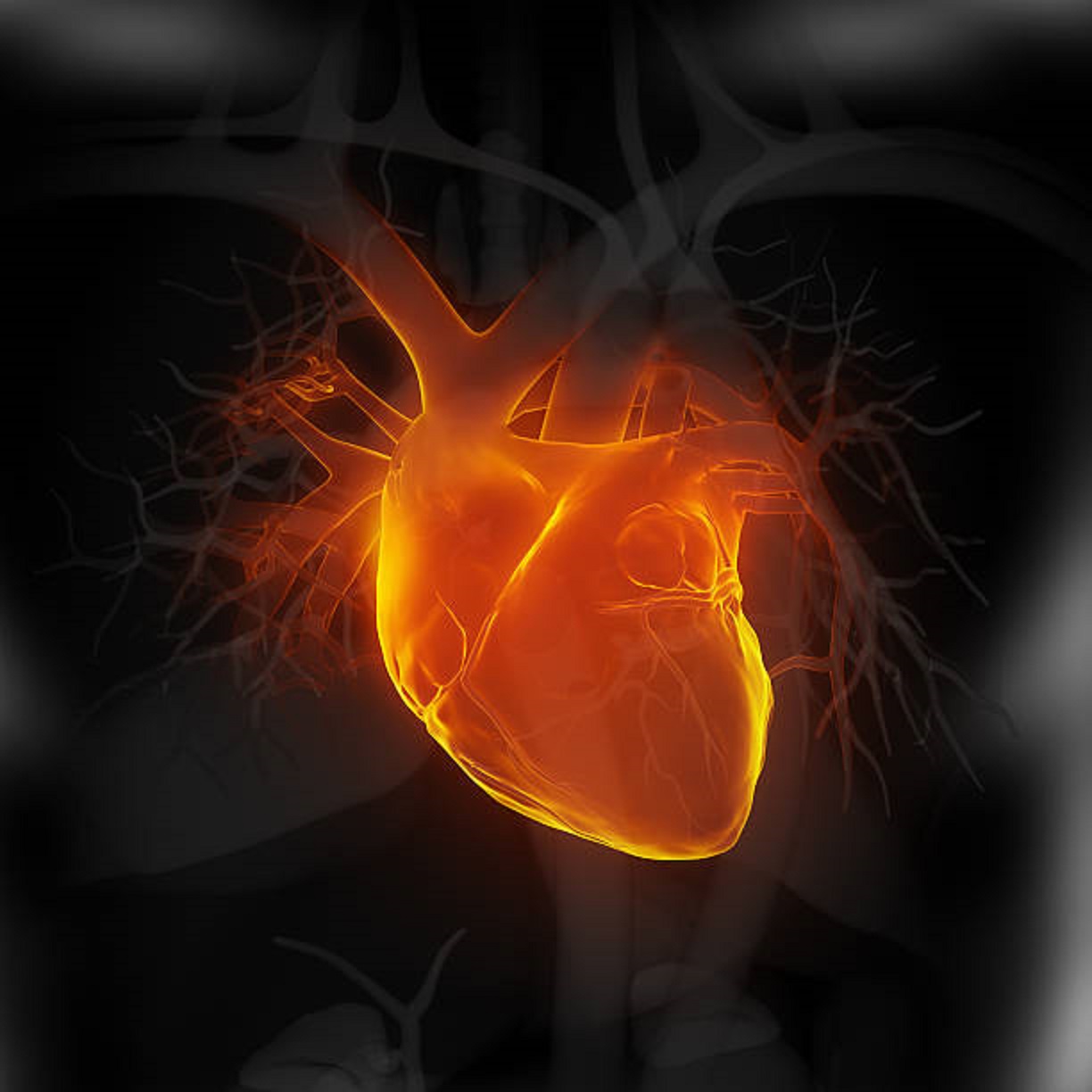
New research shows that clinical decision support (CDS) tools have not effectively reduced racial and gender disparities among cardiovascular disease (CVD) patients. Despite efforts using CDS tools to enhance care quality metrics, disparities in aspirin use, blood pressure control, and more persist. This study underscores the need for alternative interventions. Additionally, the University of Houston has developed an AI-based CDS tool for diabetes complications prediction, reflecting healthcare’s evolving landscape.
Recent research has indicated that the implementation of clinical decision support (CDS) tools has not led to significant reductions in disparities based on race and sex among patients with cardiovascular disease (CVD).
A novel study examined the effectiveness of clinical decision support (CDS) tools in various quality metrics for cardiovascular care, including the utilization of aspirin and blood pressure management. Surprisingly, the utilization of CDS tools did not result in notable reductions in healthcare disparities within the population of CVD patients. This discovery has sparked the necessity for further exploration of alternative interventions.
The study defines clinical decision support (CDS) tools as encompassing electronic health record (EHR) prompts, standing medical orders, and clinical registries. These tools are designed to enhance patient outcomes.
Although clinical decision support (CDS) tools are often associated with resourcefulness, their impact on healthcare disparities remains ambiguous. Researchers sought to investigate the effectiveness of population-based CDS tools in mitigating racial and gender differences in cardiovascular care.
To this end, a cross-sectional study was conducted, utilizing practice-level data from the Agency for Healthcare Research and Quality-supported EvidenceNOW initiative. This initiative spanned from May 1, 2015, to April 30, 2021, across 12 states. It aimed to improve preventive cardiovascular care by providing quality improvement assistance to smaller primary care practices.
The researchers drew their conclusions by considering practice-level estimates of disparities among different races and genders.
In total, the EvidenceNOW initiative encompassed 576 primary care practices that supplied survey data and EHR-derived ABCS (Aspirin use, Blood pressure control, Cholesterol management, Smoking cessation) data categorized by race and gender. Out of these practices, 219 included patient panels predominantly consisting of White patients, while 327 contained panels dominated by female patients.
Discrepancies were evident among patients meeting various metrics analyzed in the study. White patients held a larger proportion concerning blood pressure and cholesterol management, whereas men exhibited a higher share in terms of aspirin use and cholesterol management in comparison to women.
However, the opposite trend was observed in blood pressure control and smoking cessation counseling, where women surpassed men.
Except for the smoking cessation metric, the utilization of CDS tools did not demonstrate a correlation with disparities based on race and gender. Nonetheless, practices that employed CDS tools showed a higher proportion of male patients meeting the smoking counseling metric relative to female patients.
Despite the limited disparities observed within practices implementing CDS tools, these resources failed to significantly alleviate inequities. This conclusion underscores the imperative of seeking out alternative interventions.
The integration of CDS tools to enhance healthcare is becoming an increasingly prominent practice.
In November 2022, researchers from the University of Houston developed an artificial intelligence (AI)-based CDS tool. This tool harnessed the power of deep learning (DL) to assess the risk of diabetes-related complications in patients.
Dubbed “Primary Care Forecast,” this tool relied on DL techniques and patient historical data to create the Diabetes Complication Severity Index (DCSI) Progression Tool.
Dr. Winston Liaw, the lead investigator of the project and chair of the Department of Health Systems and Population Health Sciences at the UH Tilman J. Fertitta Family College of Medicine, expressed his vision for the tool: “Our ultimate aim is to empower clinicians to be more proactive rather than reactive when managing diabetes. By leveraging the capabilities of artificial intelligence and machine learning, we can effectively connect individuals at risk with interventions before their conditions worsen.”
Both of these endeavors exemplify the evolution of healthcare. As the capabilities of CDS tools continue to expand, researchers strive to harness them to their fullest potential.