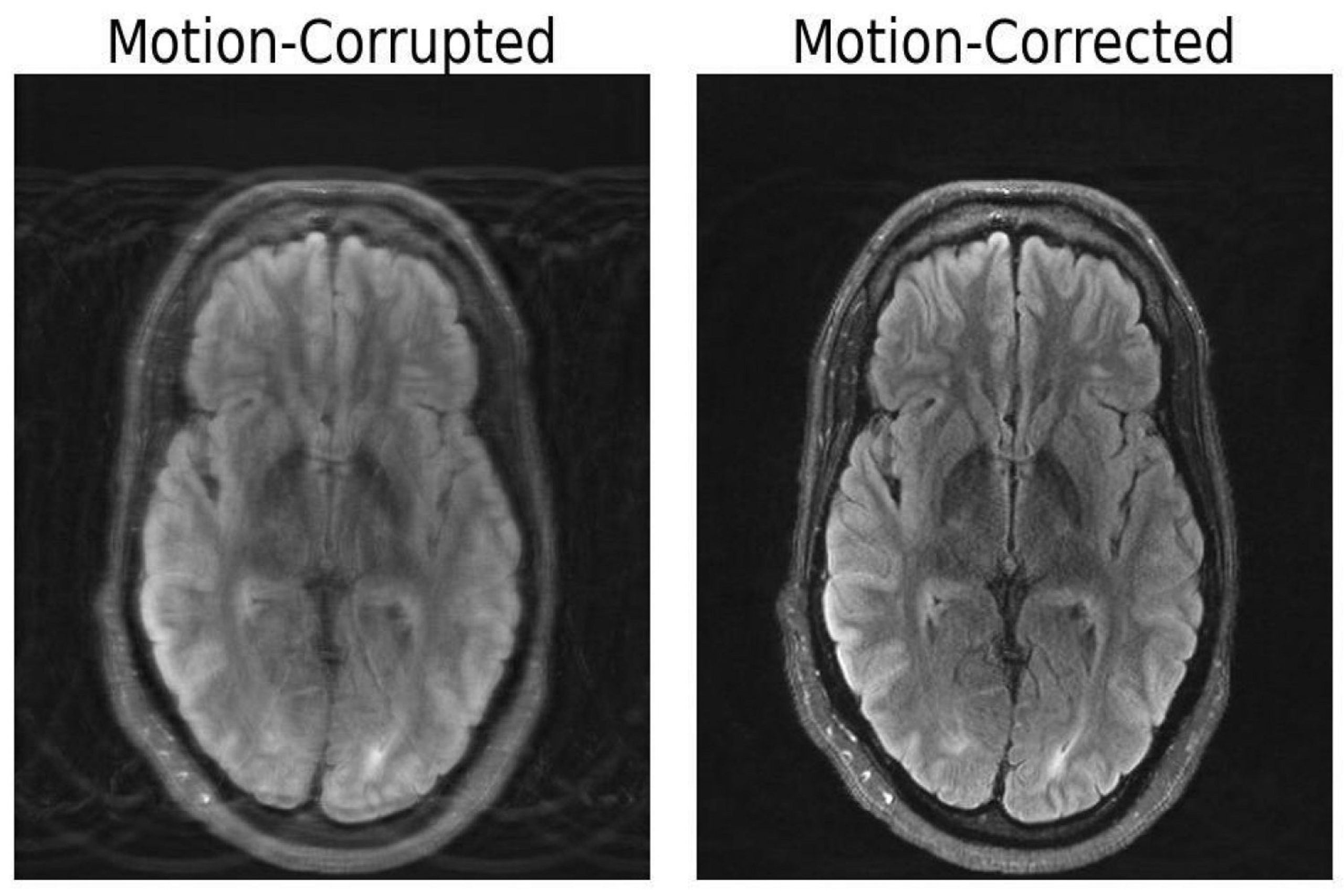
MIT researchers have developed a deep learning model that rectifies motion-distorted brain MRI scans. Combining physics principles and AI, the approach addresses artifacts caused by patient movements during slow MRI imaging. This innovation aims to reduce misdiagnosis risks, particularly in sensitive patient groups. The method’s success lies in maintaining image accuracy, which has the potential to trim excessive hospital costs linked to repeat scans. Future applications could extend to other body parts and complex motion scenarios, revolutionizing MRI practices.
MIT scientists have effectively employed the fusion of deep learning and physics principles to address the challenge of correcting brain MRI scans that have been compromised by motion artifacts. This breakthrough has the potential to significantly reduce the likelihood of misdiagnoses.
A recent preprint paper on arXiv details how researchers from the Massachusetts Institute of Technology (MIT) have devised a deep learning (DL) model specifically engineered to rectify magnetic resonance imaging (MRI) scans that have been distorted due to motion artifacts.
While MRI scans outshine computed tomography (CT) scans and X-rays in capturing intricate soft tissue contrasts, they come at the cost of being slower. Moreover, even slight movements, like a patient taking a deep breath can greatly disrupt MRI scans.
These motion-induced disturbances can obscure vital information for medical professionals interpreting the scans, leading to image artifacts. These artifacts can potentially compromise an entire image, posing the risk of misdiagnosis or inappropriate treatment decisions.
Nalini Singh, a lead author of the paper and a Ph.D. student affiliated with the Abdul Latif Jameel Clinic for Machine Learning in Health (Jameel Clinic) in the Harvard-MIT Program in Health Sciences and Technology (HST), emphasized, “Motion is a frequent concern in MRI, given its relatively slow imaging process.”
The duration of an MRI session varies based on the type of imaging needed, typically ranging from several minutes to an hour. As the scan duration extends, the chances of small movements occurring that can lead to artifacts in the final scan also increase.
To mitigate motion-related issues, medical practitioners may opt to anesthetize patients or request controlled breathing. However, these strategies aren’t always feasible for patient groups that are prone to motion, such as children and individuals with psychiatric disorders.
To tackle this challenge, the researchers endeavored to construct a model capable of rectifying distorted MRIs. Their innovative approach involves computationally generating motion-free images from the data affected by motion artifacts, without necessitating any alterations to the scanning procedure.
Singh elucidated, “Our objective was to amalgamate physics-based modeling and deep learning to harness the advantages of both methodologies.”
The effectiveness of their approach hinges on maintaining the fidelity of the image output with the actual measurements of the depicted subject. This ensures that the model doesn’t inadvertently generate seemingly realistic images that exhibit inaccuracies upon closer examination.
The research team underscored that these inaccuracies could detrimentally impact diagnoses and patient outcomes, highlighting the critical need for the model to avoid such discrepancies.
Additionally, the authors emphasized that their model, along with similar technologies, could not only enhance patient outcomes but also contribute to reducing unnecessary healthcare expenses associated with repeated MRI scans due to motion artifacts.
Looking ahead, the researchers are considering the possibility of applying this approach to MRIs of other body parts or for cases involving more complex head motions, such as fetal MRIs.
According to Daniel Moyer, an assistant professor of computer science at Vanderbilt University who specializes in machine learning (ML) in medical imaging, this work represents a significant advancement in MRI motion correction. He believes that these methods will likely become commonplace in a wide array of clinical scenarios, benefiting various patient demographics and clinical contexts.
Moreover, other researchers are also exploring ways to enhance MRI capabilities through cutting-edge technologies. In January, a collaboration between New York University (NYU) Grossman School of Medicine and Meta AI Research unveiled how artificial intelligence (AI) could expedite and enhance the accuracy of MRI reconstruction, outperforming conventional techniques. This initiative, known as fast MRI, aims to leverage AI to accelerate MRI scans, potentially addressing the issue of prolonged patient wait times and expanding MRI accessibility.