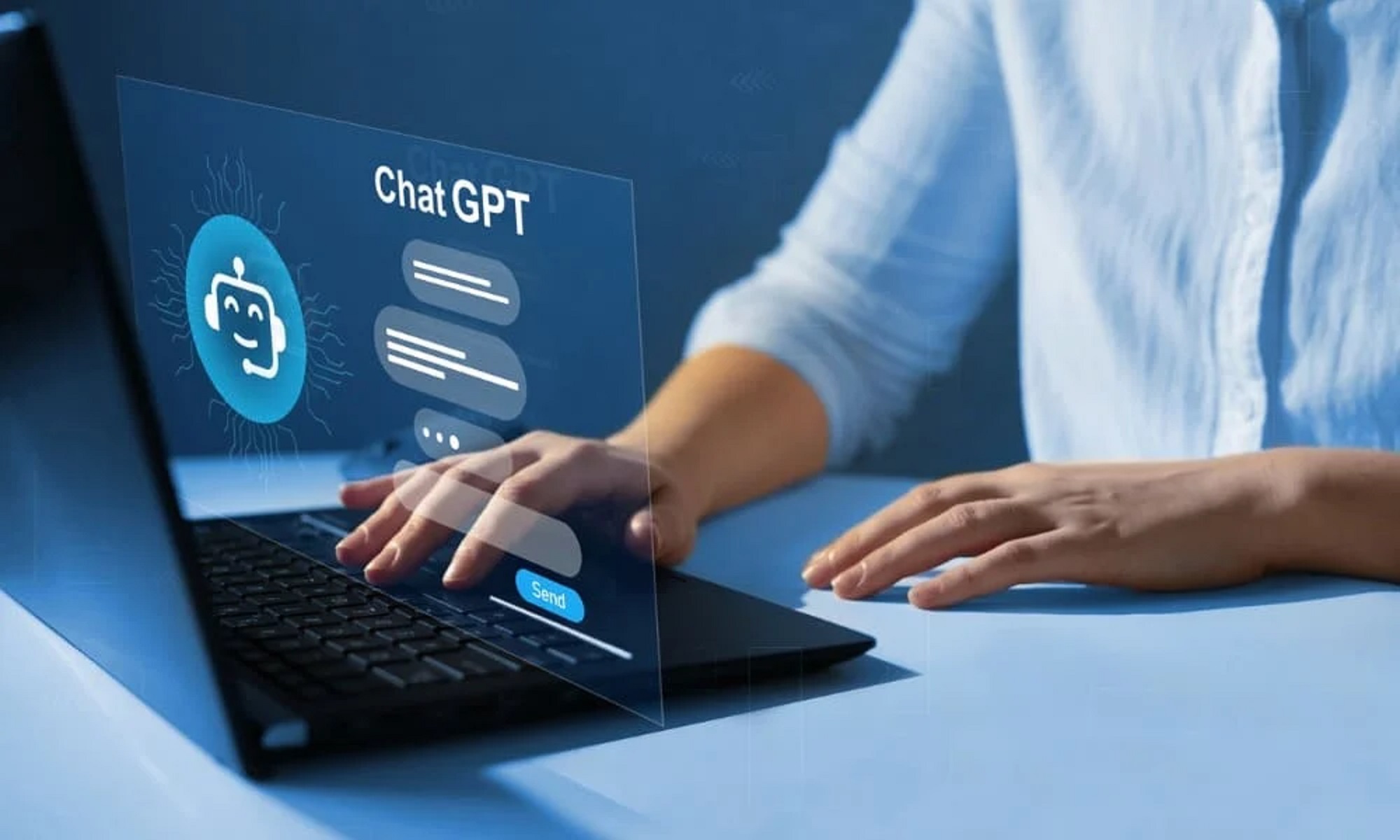
ChatGPT achieved a 72% accuracy in clinical decision-making, per Mass General Brigham’s study. It excelled in final diagnoses (76.9%) but struggled with initial differential diagnoses (60.3%). Researchers view it as a valuable medical tool for decision support, comparable to a recent medical graduate’s level. However, challenges like ambiguous training data and model hallucinations need to be addressed before clinical implementation. The study underscores AI’s role in aiding, not replacing, clinicians, particularly in resource-limited settings.
Researchers at Mass General Brigham recently completed a study in which they found that ChatGPT performed a variety of clinical decision-making tasks across diverse care contexts with an overall accuracy rate of 72%.
The researchers found that ChatGPT exhibited remarkable accuracy in clinical decision-making, particularly when it was provided with more clinical information. This conclusion was drawn from a study published in the Journal of Medical Internet Research (JMIR), where Mass General Brigham researchers examined the capabilities of large language models (LLMs) and AI-driven chatbots in healthcare. While the potential of LLMs and AI in healthcare is growing, their specific role in clinical reasoning and decision-making has not been thoroughly investigated.
The research aimed to assess ChatGPT’s effectiveness in offering clinical decision support across medical specialties within primary care and emergency department contexts. The approach involved inputting 36 published clinical vignettes into the model and tasking it with generating recommendations for differential diagnoses, diagnostic testing, final diagnosis, and management for each case. The recommendations were tailored to the patient’s gender, age, and case severity provided in the vignettes.
Dr. Marc Succi, the corresponding author of the study and an associate chair of innovation and commercialization, emphasized that the research extensively evaluated ChatGPT’s decision-support capabilities throughout the patient care process, encompassing everything from initial differential diagnosis to testing, diagnosis, and management.
The model’s accuracy was measured by comparing its responses to human-scored answers for the questions in each vignette. According to these evaluations, ChatGPT achieved an overall accuracy of 71.7 percent across all 36 clinical scenarios.
The model’s highest accuracy, at 76.9 percent, was in generating final diagnoses. However, its performance was slightly lower at 60.3 percent for initial differential diagnoses. The model achieved 68 percent accuracy in clinical management decisions, and this performance remained consistent across primary care and emergency care contexts.
Comparatively, ChatGPT’s performance was less impressive when tasked with differential diagnoses and clinical management compared to answering general medical knowledge questions. Notably, the model’s responses were unbiased in terms of gender.
Dr. Succi noted that the performance level achieved by ChatGPT is comparable to that of a freshly graduated medical professional, such as an intern or resident. This suggests that large language models could significantly contribute to medical practice by providing accurate clinical decision support.
The researchers highlighted that ChatGPT’s performance, while impressive, still faces two significant limitations that must be addressed before its implementation in clinical care: the lack of clear information about the model’s training data and the potential for model hallucinations.
This study underscores the potential of advanced technologies like ChatGPT to assist medical professionals rather than replace them. Dr. Succi explained that while ChatGPT struggled with initial differential diagnoses, it excelled in areas where physicians bring the most value: generating possible diagnoses based on limited initial information.
Moving forward, the research team plans to explore how AI tools can enhance patient care and outcomes, especially in resource-constrained healthcare environments.
This research contributes to the growing body of work examining the potential of large language models in healthcare. For instance, researchers from New York University’s Grossman School of Medicine recently deployed their LLM, NYUTron, to predict clinical outcomes and demonstrated improvements in areas such as readmission forecasting and length of stay prediction.