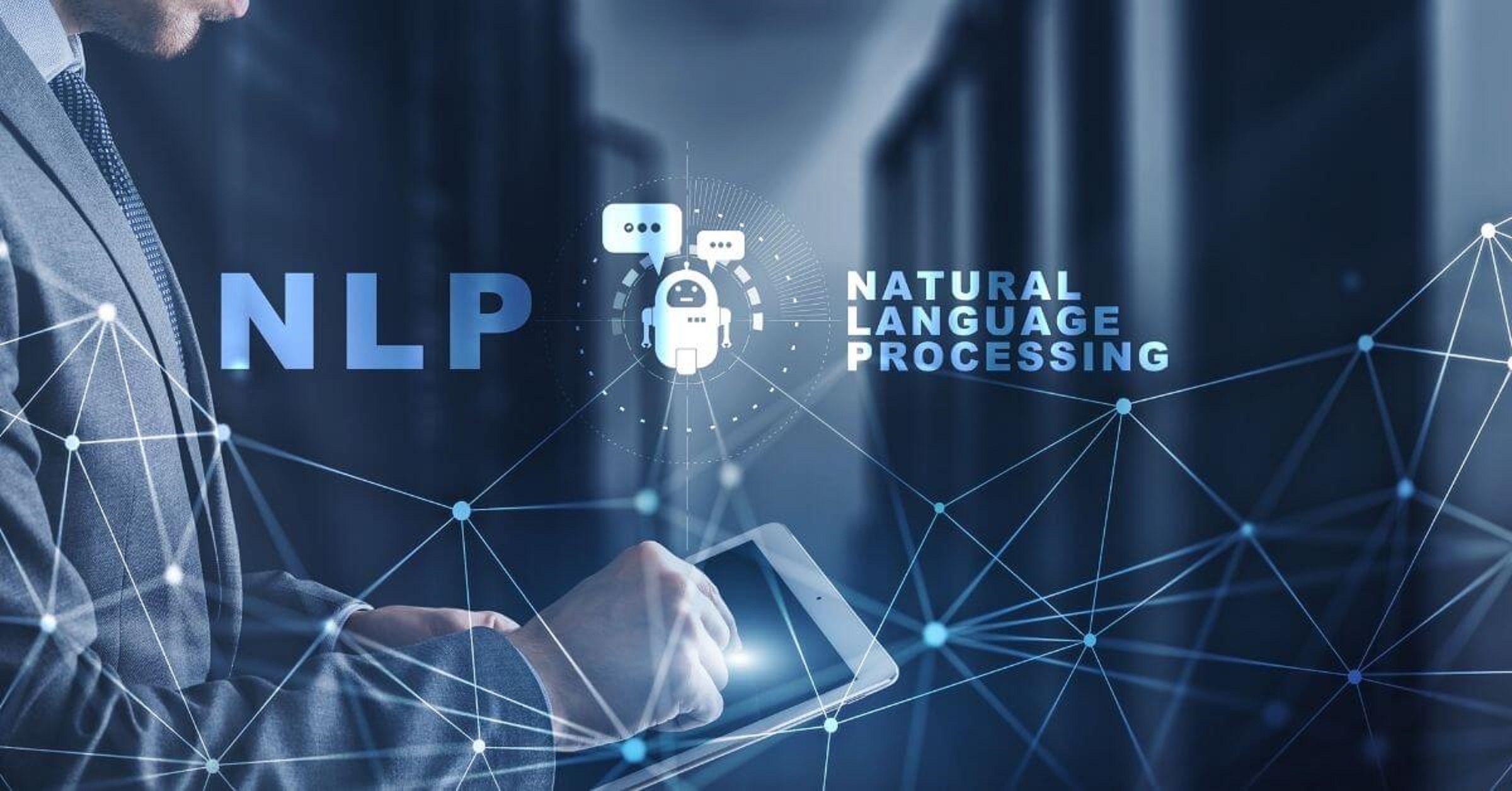
Healthcare generates vast data, often trapped in Electronic Health Records. Natural Language Processing (NLP) can unlock this treasure trove. NLP comprises Natural Language Understanding (NLU) and Natural Language Generation (NLG). NLU grasps meaning from text, while NLG crafts human-like responses. NLP holds promise in healthcare, aiding diagnosis, research, and EHR data analysis. Despite hurdles like data quality and bias, its potential fuels research to harness its power effectively.
Healthcare generates vast volumes of data as patients progress through their care journeys, primarily in the form of clinical notes stored in Electronic Health Records (EHRs). While this data holds immense potential to enhance health outcomes, it often remains challenging to access and analyze effectively.
Enter Natural Language Processing (NLP) technologies, offering a promising solution to the conundrum. These tools empower care teams and researchers to sift through this data mountain, extracting valuable insights. These insights find applications in population health management and clinical decision support.
Although NLP’s potential in healthcare has generated considerable buzz, its two major components, Natural Language Understanding (NLU) and Natural Language Generation (NLG), have received comparatively less attention.
Let’s distinguish between NLP, NLU, and NLG:
– NLP draws from linguistics, artificial intelligence, and computer science to enable computers to comprehend spoken and written human language. Utilizing machine learning and deep learning techniques, NLP transforms unstructured language data into a structured format through a process known as named entity recognition. This technique classifies named entities within text into predefined categories, like individuals, organizations, locations, quantities, percentages, times, and monetary values.
– NLU, as a subset of NLP, focuses on discerning the meaning of a sentence or piece of text. It analyzes syntax (the grammatical structure) and semantics (the intended meaning). NLU also establishes an ontology, specifying relationships between words and phrases. NLU is valuable in sentiment analysis and understanding consumer attitudes.
– NLG, another component of NLP, enables computers to generate human-like text responses based on data inputs. NLG tools use NLP, along with linguistic considerations like syntax, semantics, lexicons, and morphology, to craft appropriate responses. NLG finds use in text-to-speech applications and AI-driven tools like ChatGPT.
Now, let’s delve into healthcare applications:
NLP technologies offer a wide array of benefits in healthcare, including improving care, aiding disease diagnosis, and enhancing clinical research. One of its most promising use cases is deciphering unstructured EHR data. NLP can automate quality measurements for conditions like heart failure, address bias in EHR-based opioid misuse classifiers, assess COVID-19 complications, predict severe maternal morbidity, provide insights into bipolar disorder, aid public health research, and identify factors contributing to patient safety events. NLP is also leveraged to accelerate genetic sequencing and detect HPV-related cancers.
Currently, some healthcare systems and academic institutions have adopted NLP tools. For instance, the University of California, Irvine, employs NLP to bolster medical research, while Mount Sinai incorporates NLP into its web-based symptom checker.
While NLU is less commonly used in healthcare, researchers explore its potential, particularly in healthcare data mining and query understanding. Examples include developing chatbots for patient information retrieval and interpreting drug therapy data from discharge summaries.
NLG has seen limited healthcare use compared to other NLP technologies, but researchers see promise in addressing healthcare’s diverse information needs. It could generate synthetic chief complaints based on EHR variables, enhance information flow in ICUs, provide personalized e-health information, and support postpartum patients.
However, barriers to adoption exist:
1. Data Access and Quality: NLP’s effectiveness depends on access to high-quality data, which can be limited by data type or research area.
2. Bias and Privacy: Biases in NLP models and privacy concerns need to be addressed. Regulations governing data use and privacy protections are still evolving.
3. Frameworks and Guidelines: There’s a need for frameworks and guidelines to ensure NLP tools function as intended, but these are yet to be developed.
4. Evaluation Challenges: NLP tools’ word- or sentence-level annotations may not align with patient or population-level clinical research studies, posing evaluation challenges.
5. Performance Issues: NLP tools must meet stringent healthcare standards, which they sometimes struggle to do. Errors in clinical documentation and lower effectiveness in identifying medical conditions have been noted.
Despite these limitations, NLP’s potential in healthcare continues to drive research aimed at overcoming these challenges and deploying these technologies effectively in clinical settings.