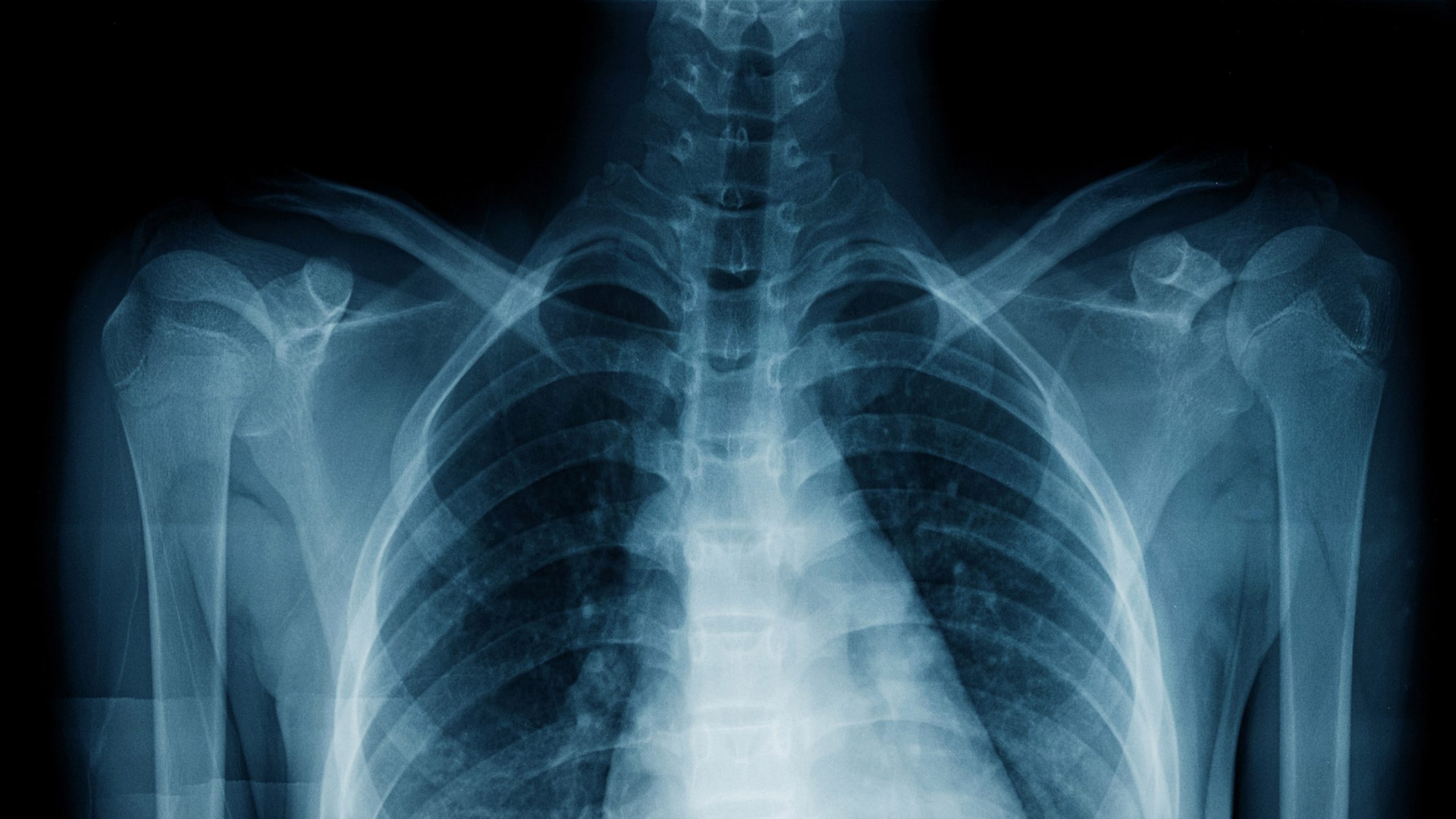
Northwestern Medicine researchers have pioneered an AI model that outperforms radiologists in chest X-ray interpretation. This groundbreaking technology, featured in JAMA Network Open, enhances emergency department diagnostics and clinical workflows. Trained on 900,000 X-rays and radiologist reports, the AI generates human-like text reports. Tests demonstrated comparable accuracy with radiologists, even detecting previously missed anomalies. The AI offers the potential to address radiologist shortages and could revolutionize various medical imaging modalities. This breakthrough highlights the potential of generative AI in healthcare.
In a groundbreaking development, Northwestern Medicine researchers have harnessed the power of generative artificial intelligence (AI) to significantly enhance the interpretation of chest X-rays. This innovative AI model has demonstrated its ability to outperform radiologists in certain cases, marking a significant step forward in medical imaging.
The brainchild of a dedicated team of researchers from Northwestern Medicine, this generative AI tool has proven its capacity to interpret chest radiographs with remarkable precision. In a study published in JAMA Network Open, this extraordinary tool has shown its potential to not only match but even surpass the diagnostic capabilities of radiologists for specific medical conditions.
The primary aim of this AI tool, as elucidated by Jonathan Huang, the first author of the study, was to bridge the gap in healthcare, particularly in settings where emergency department radiologists might be scarce. In these critical scenarios, the AI model acts as an invaluable assistant, generating text reports from medical images that help streamline clinical workflows and boost overall efficiency.
To create this pioneering AI model, the research team employed a dataset of 900,000 chest X-rays along with corresponding radiologist reports. Through a rigorous training process, the AI model learned to generate reports for each image. These reports were constructed in a way that closely emulated the language and style of a human radiologist, accurately describing the clinical findings and their relevance.
The AI model’s mettle was then put to the test by evaluating its performance on 500 chest X-rays from an emergency department at Northwestern Medicine. The results of the AI-generated interpretations were compared to those produced by radiologists and teleradiologists who initially assessed each image in a clinical setting.
The significance of this research lies in its real-world applicability, specifically in emergency department scenarios where onsite radiologists might not be readily available. The AI model, as demonstrated, can act as a valuable complement to human decision-making, ensuring a higher level of care and accuracy.
Furthermore, the researchers engaged five board-certified emergency medicine physicians to assess the AI-generated reports. These experts rated each report on a scale from one to five, with a rating of five indicating concurrence with the AI’s interpretation and the absence of any necessary revisions.
Through this meticulous process, the researchers uncovered the AI’s remarkable ability to identify chest X-rays with significant clinical findings and produce high-quality imaging reports. Notably, the study revealed that there was no significant difference in accuracy between radiologist- and AI-generated reports, even for life-threatening conditions like pneumonia.
For the detection of abnormalities, the AI model exhibited a sensitivity of 84 percent and a specificity of 98 percent, compared to the on-site radiologists. In contrast, the original reports from teleradiologists had a sensitivity of 91 percent and a specificity of 97 percent for the same task.
In some instances, the AI tool even identified findings that had been overlooked by radiologists, such as a pulmonary infiltrate in one of the X-rays. This showcases the AI model’s potential to not only match but surpass human diagnostic capabilities.
The researchers emphasized that this generative AI model represents a significant breakthrough in the field of medical imaging. Unlike many existing AI tools in radiology, which tend to serve a single purpose, this AI model provides a comprehensive analysis of medical images and diagnoses, even outperforming some medical professionals.
Looking ahead, the research team has ambitious plans to expand the model’s capabilities to encompass other imaging modalities such as MRIs, ultrasounds, and CAT scans. Their vision is to make this tool a valuable resource for clinics facing workforce shortages, significantly improving patient care.
As senior author Mozziyar Etemadi, MD, PhD, pointed out, “We want this to be the radiologist’s best assistant, and hope it takes the drudgery out of their work.” The team has initiated a pilot study where radiologists are actively involved in evaluating the tool’s real-time application.
This breakthrough in generative AI is not an isolated incident. Medical institutions worldwide are exploring the potential of AI in various healthcare applications. For instance, a team of researchers from Johns Hopkins Medicine recently unveiled a machine-learning model that estimates the percent necrosis (PN) in patients with intramedullary osteosarcoma. This model streamlines the assessment of tumor necrosis, which is vital for treatment evaluation and prognosis estimation.
The possibilities presented by generative AI in healthcare are limitless. With ongoing research and development, these AI tools hold the promise of revolutionizing medical diagnostics and improving patient outcomes.