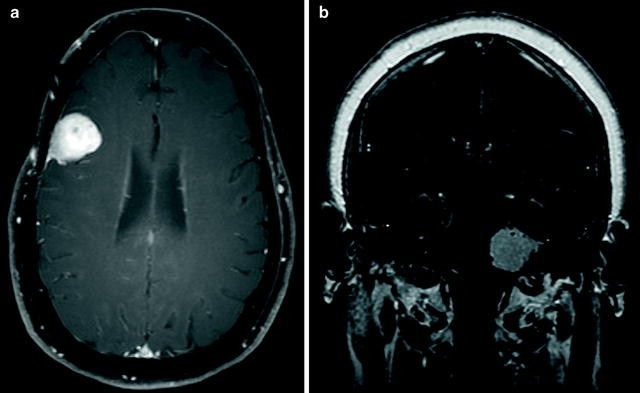
Utilizing machine learning (ML) and mass spectrometry, UF Health researchers achieved 87% accuracy in meningioma tumor grade classification. Published in the Journal of the American Society for Mass Spectrometry, their study showcased the enhanced precision of tumor characterization. This approach analyzed 85 samples, revealing crucial biomarkers for tumor diagnosis. ML integration significantly expedited data assessment, offering a remarkable 17,000 data point analysis in seconds. This innovative method redefines brain tumor evaluation, potentially reclassifying tumors based on genetic insights.
Combining machine learning (ML) with liquid chromatography-high resolution mass spectrometry (LC-HRMS), UF Health researchers aimed to refine meningioma tumor assessments. The ambiguity surrounding grade II tumors prompted the exploration of an approach to guide treatment decisions more accurately. Analyzing 85 meningioma samples, the study aimed to delineate chemical profiles and unearth biomarkers pivotal for diagnostic precision. Initially focusing on metabolomic analysis, the team identified the potential of ML integration for deeper insights. The integration of ML exponentially increased data processing speed, revolutionizing tumor evaluation efficiency and offering new avenues for personalized treatments.
Researchers at the University of Florida (UF) Health have pioneered a groundbreaking approach that combines machine learning (ML) with liquid chromatography-high resolution mass spectrometry (LC-HRMS) to significantly improve the assessment of meningioma brain tumors. Published in the Journal of the American Society for Mass Spectrometry, their study showcases an 87% accuracy rate in classifying tumor grades, offering promising implications for accurate tumor characterization and subsequent treatment decisions.
Meningioma tumors, classified as grade I, grade II, and grade III, pose varying levels of risk and complexity. Grade I tumors are usually slow-growing and less invasive, often necessitating regular monitoring and surgical removal. Conversely, grade III tumors are more aggressive, requiring a combination of surgical intervention and radiation therapy. However, the challenge lies in grade II tumors, where the optimal treatment approach remains uncertain.
Dr. Jesse L. Kresak, a clinical associate professor at the UF College of Medicine, emphasized the ambiguity surrounding grade II tumors, stating, “Grade II tumors are the gray zone.” This uncertainty led the research team to pursue a solution that could provide a more nuanced evaluation to aid clinicians in making informed treatment choices.
The researchers meticulously examined 85 meningioma samples, delving into the chemical compositions of the tumors’ small molecules and fats. This comprehensive analysis allowed for a detailed differentiation between tumor grades, pinpointing potential biomarkers crucial for accurate diagnosis.
Initially focusing on metabolomic analysis within tumor cells to distinguish benign from malignant tumors, the researchers realized the untapped potential of integrating ML into their study. Dr. Timothy J. Garrett, a co-author of the study, highlighted, “Machine learning could be a good opportunity to find things that we wouldn’t be able to find ourselves.”
The inclusion of ML revolutionized the efficiency of tumor evaluation. While traditionally examining around 20 data points in ten minutes, incorporating ML-enabled the analysis of a staggering 17,000 data points in less than a second without compromising accuracy. Initial tests showed one ML model accurately classifying tumor grades with an 87% success rate, a figure projected to improve with a larger dataset for analysis.
The team highlighted the significance of their findings in potentially reclassifying tumors based on new genetic information, enhancing diagnostic precision, and tailoring treatments for individual patients.
This pioneering research exemplifies the healthcare sector’s growing utilization of data analytics and artificial intelligence (AI) in oncology. Coinciding with this trend, the University of Texas MD Anderson Cancer Center recently established its Institute for Data Science in Oncology (IDSO). This institute aims to leverage clinical expertise and data science collaboration to address the pressing needs of cancer patients.
IDSO focuses on fostering partnerships across medicine, academia, science, and industry to advance personalized care and elevate the patient experience through enhanced data generation, collection, and management. This initiative marks a concerted effort to transform cancer care by harnessing the power of data analytics and AI technologies.
Overall, the collaboration of ML and mass spectrometry heralds a transformative era in meningioma tumor characterization. This pioneering study illuminates a path toward more precise grading and personalized treatments. By swiftly analyzing vast data points, the ML-enhanced approach demonstrates its potential to improve diagnostic accuracy. The prospect of reclassifying tumors based on genetic information holds promise for tailored interventions. These advancements underscore the evolving role of technology in healthcare, emphasizing the profound impact of data-driven methodologies on reshaping brain tumor evaluations and, ultimately, patient care.