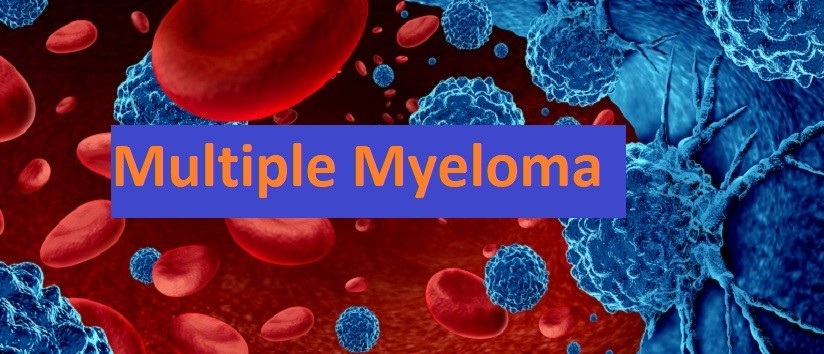
In a groundbreaking stride, the study introduces IRMMa, a state-of-the-art computational model poised to reshape the prognosis landscape for multiple myeloma. Unlike conventional models, IRMMa, developed through meticulous analysis of tumor genomics, offers a nuanced, individualized risk prediction. The model’s flexibility and capacity to adapt to evolving patient prognoses set it apart, providing a dynamic tool for clinicians. As healthcare embraces AI and machine learning, IRMMa emerges as a beacon of personalized medicine, holding the potential to refine treatment strategies and significantly improve outcomes for those grappling with this complex and variable disease.
The quest for precision medicine in multiple myeloma reaches a milestone with the introduction of IRMMa. Published in the Journal of Clinical Oncology, this groundbreaking model promises to transcend the limitations of traditional prognostic tools by intricately incorporating tumor genomics. Unlike its predecessors, which relied on population-level averages, IRMMa focuses on predicting individual patient risks. Led by Dr. C. Ola Landgren, the research team identified 12 distinct subtypes of multiple myeloma during IRMMa’s development, underscoring the importance of tailoring treatments to the unique genetic makeup of each patient. The evolving landscape of cancer care demands a shift toward personalized risk assessment, and IRMMa emerges as a pivotal player in this transformative journey.
Evolution of Multiple Myeloma Assessment:
Historically, multiple myeloma assessment relied on staging solid tumors, a method developed in the 1970s. At that time, the approach was accurate based on available treatments and the quantifiable presence of cancer. However, the oncology landscape has evolved significantly, introducing diverse treatment options that necessitate a more nuanced evaluation of therapeutic choices in the context of a patient’s prognosis.
Challenges in Prognosis Tools:
Despite advancements in cancer treatment, predicting patient outcomes remains a challenge due to limited tools. Existing prediction models often rely on population-level averages, categorizing patients into low, standard, and high-risk groups. However, this approach fails to consider individualized risk and its susceptibility to treatment impacts. The research team emphasizes the need for a paradigm shift towards precision medicine.
IRMMa Development and Methodology:
The researchers, led by senior author Dr. C. Ola Landgren, leveraged clinical, treatment, and genetic data from 2,000 newly diagnosed multiple myeloma patients across prominent institutions. Utilizing patient DNA sequences, the team identified 90 “driver genes” with mutations driving tumor growth. By matching treatment outcomes to individual genetic sequences, the researchers employed machine learning to discern patterns and create a flexible risk prediction model.
Unique Features of IRMMa:
IRMMa stands out by predicting the risk for individual patients rather than relying on group-level predictions. The model’s flexibility allows adaptation to changes in prognosis post-treatment. As new therapies emerge, additional patient data can be integrated to continually refine and enhance the model’s predictive accuracy.
Identifying Distinct Subtypes:
During IRMMa’s development, the research team identified and classified 12 distinct subtypes of multiple myeloma. This granularity provides unprecedented insights into the disease, emphasizing the importance of tailoring treatments to individual patient profiles.
Future Implications and Community Collaboration:
While currently intended for research purposes, the research team envisions expanding IRMMa’s utility by incorporating more patient data. The model’s growth relies on collaborative efforts within the research community, with an emphasis on feeding it diverse datasets to enhance its clinical applicability.
Advancements in Healthcare Predictive Models:
The use of advanced technologies, such as artificial intelligence (AI) and machine learning, is increasingly prevalent in healthcare. This extends beyond cancer research, as demonstrated by a recent development where researchers successfully created a risk score to predict intensive care unit (ICU) admission and survival among older adults living in the community. The population-level risk model achieved high performance in distinguishing between patients based on survival outcomes and ICU admission.