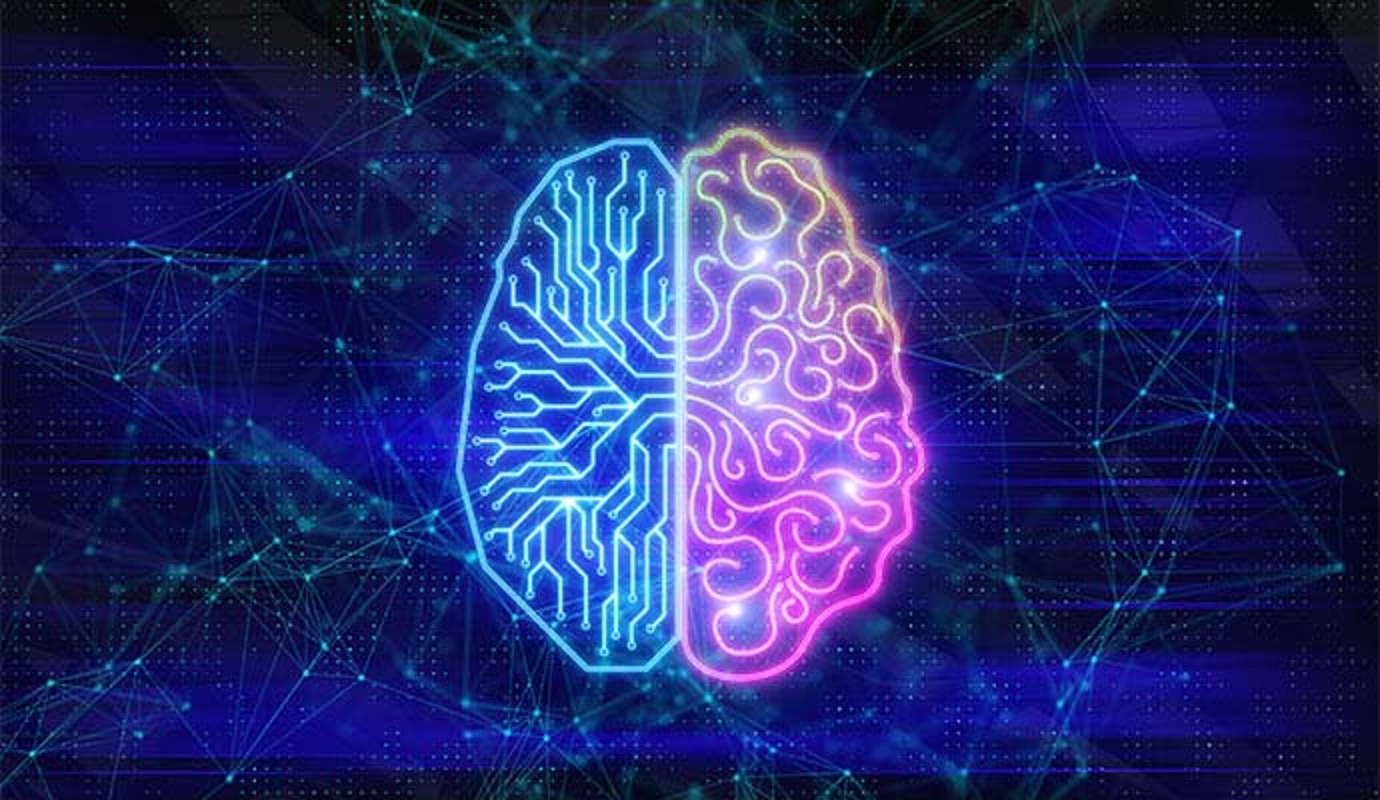
Cutting-edge deep learning techniques, coupled with metabolic biomarkers, are revolutionizing Alzheimer’s disease detection and prediction. Research teams from institutions like West Virginia University (WVU) and the University of Texas at Arlington (UTA) are at the forefront of this transformative endeavor. By harnessing the power of artificial intelligence and intricate metabolic data, these studies offer new hope in identifying AD long before its clinical onset, paving the way for proactive interventions. Through meticulous analysis and integration of diverse datasets, these pioneering efforts aim to mitigate the devastating impact of Alzheimer’s, enhancing patient care and quality of life.
Alzheimer’s disease represents a formidable challenge to healthcare systems worldwide, with its relentless progression exacting a heavy toll on individuals and caregivers alike. However, recent advancements in deep learning methodologies and metabolic biomarker research present a beacon of hope in the fight against this debilitating condition. Spearheaded by research teams from leading institutions such as WVU and UTA, these initiatives strive to unravel the intricate complexities of AD pathology and progression. By leveraging artificial intelligence and sophisticated data analytics, these studies seek to identify subtle biomarkers indicative of AD onset, enabling early detection and proactive management strategies.
At the heart of this pioneering effort lies a deep learning model meticulously crafted to sift through intricate metabolic data, unveiling subtle patterns indicative of impending Alzheimer’s pathology. Spearheading the study is Dr. Kesheng Wang, a distinguished professor in the WVU School of Nursing, who underscores the significance of artificial neural networks inspired by the intricate workings of the brain’s neurons. These networks, harnessed within the deep learning paradigm, exhibit unparalleled prowess in deciphering complex datasets, outperforming conventional machine learning methods in AD diagnosis with unprecedented accuracy.
Central to the research endeavor is the recognition that AD’s ominous shadow looms years, if not decades, before its clinical manifestations surface. Hence, the identification of predictive biomarkers assumes paramount importance, laying the groundwork for preemptive interventions to thwart disease progression. Employing the Least Absolute Shrinkage and Selection Operator (LASSO) algorithm, the team meticulously winnowed down a myriad of potential biomarkers extracted from Ultra Performance Liquid Chromatography Mass Spectrometry (UPLC-MS/MS) data. Among these, 21 emerged as pivotal players in the AD landscape, exerting tangible effects on hippocampal atrophy, a harbinger of AD and other dementias.
Crucially, these metabolites, intricately entwined within glucose, amino acid, and lipid metabolic pathways, bear profound correlations with clinical hallmarks of AD, such as plaques, cognitive metrics, and hippocampal volume. To put these findings to the test, the researchers integrated them into a series of deep learning models, subjecting them to rigorous evaluation using data gleaned from the Alzheimer’s Disease Neuroimaging Initiative (ADNI) dataset. The results were nothing short of astonishing, with the highest-performing model boasting an accuracy of 0.881, an F1-score of 0.892, and an area under the curve (AUC) of 0.873.
Yet, amidst the jubilation sparked by these remarkable achievements, the researchers remain cognizant of the nascent stage of deep learning applications in AD detection. They advocate for sustained inquiry, with a pledge to further enrich their model by integrating metabolomic and protein data. Dr. Wang elucidates the broader implications of their research, emphasizing the tantalizing prospect of identifying metabolic biomarkers that not only herald AD diagnosis but also delineate its inexorable progression.
In parallel strides, researchers at the University of Texas at Arlington (UTA) are forging ahead, leveraging advanced analytics to unravel the intricate tapestry of AD progression. Their novel framework, underpinned by a learning-based approach, endeavors to forecast the trajectory of AD evolution, empowering patients and caregivers alike to anticipate and address evolving care needs. Codifying the disease’s developmental stages into a cohesive framework dubbed the “disease-embedding tree” (DETree), this innovative tool offers a panoramic view of an individual’s clinical status and the trajectory of their AD journey.
By projecting patient data onto this comprehensive framework, clinicians can glean invaluable insights into the dynamic interplay between AD pathology and clinical manifestations, facilitating tailored interventions aligned with the evolving needs of patients. Moreover, the DETree framework serves as a beacon of hope, illuminating the path toward personalized medicine and proactive management of AD, thereby alleviating the burden borne by patients and caregivers alike.
Overall, the fusion of deep learning methodologies and metabolic biomarkers heralds a promising new frontier in Alzheimer’s disease research. Through collaborative efforts and interdisciplinary approaches, researchers are unraveling the mysteries of AD pathology, offering unprecedented insights into its early detection and prediction. As these transformative technologies continue to evolve, they hold the potential to revolutionize clinical practice, empowering healthcare professionals with the tools needed to combat Alzheimer’s disease proactively. With each breakthrough, we move closer to a future where timely interventions and personalized treatments mitigate the devastating impact of AD, offering renewed hope to millions affected by this insidious condition.