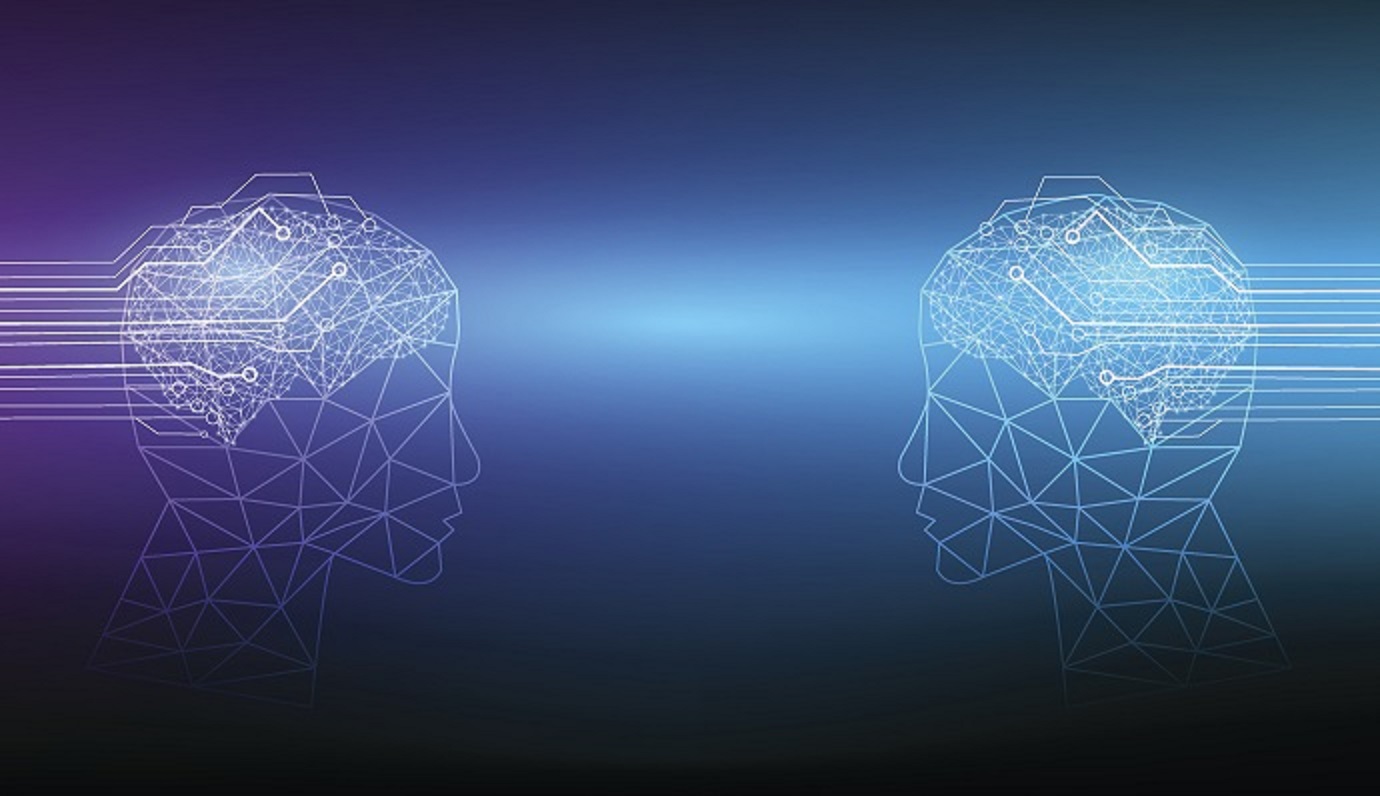
In the quest to refine medical text summarization, researchers at Pennsylvania State University have devised a groundbreaking NLP framework, FaMeSumm. This framework aims to address concerns regarding the fidelity of AI-generated medical summaries by fine-tuning language models and minimizing errors. By analyzing diverse datasets and developing contrastive summaries, FaMeSumm demonstrates consistent improvements in faithfulness and accuracy across various medical contexts. The study not only highlights the efficacy of FaMeSumm in enhancing summarization tools but also underscores the transformative potential of fine-tuned LLMs in healthcare decision-support systems.
The evolution of natural language processing (NLP) has ushered in a new era of medical text summarization, promising streamlined access to crucial patient information. However, concerns regarding the reliability and fidelity of AI-generated summaries persist, necessitating innovative solutions. Against this backdrop, researchers at Pennsylvania State University have introduced the Faithfulness for Medical Summarization (FaMeSumm) framework. This pioneering framework seeks to mitigate the risk of inaccuracies and inconsistencies in medical summaries by leveraging advanced NLP techniques. By analyzing prevalent error patterns and refining language models, FaMeSumm endeavors to enhance the efficiency and safety of medical summarization tools.
Unveiling FaMeSumm: A Paradigm Shift in Medical Summarization
These summarization tools play a pivotal role in distilling extensive patient information into concise yet comprehensive summaries applicable across various medical contexts such as electronic health records, insurance documentation, and immediate clinical decision-making. However, the utilization of AI for generating these summaries introduces concerns regarding the fidelity and reliability of the synthesized information.
Nan Zhang, the primary author of the study and a graduate student at the PSU College of Information Sciences and Technology (IST) underscored the challenge of ensuring absolute consistency between generated summaries and the original medical records. Zhang emphasized the criticality of maintaining fidelity to prevent any potential misinformation or misinterpretation that could adversely impact patient care outcomes.
Existing medical summarization models often rely on human oversight to mitigate the risk of generating inaccurate or inconsistent summaries. Nonetheless, understanding the underlying sources of unfaithfulness within these models is imperative for optimizing their efficiency and safety.
To delve into the complexities of model unfaithfulness, researchers scrutinized three distinct datasets originating from prevailing tools in radiology report summarization, medical dialogue summarization, and online health question summarization. Through meticulous manual comparison between randomly selected summaries and their corresponding source medical reports, the researchers identified various error categories contributing to unfaithful summarization, including discrepancies and instances of “hallucination” where summaries included erroneous supplementary information.
In response to these identified challenges, the research team devised the Faithfulness for Medical Summarization (FaMeSumm) framework. This framework, developed through the analysis of contrastive summaries – categorically classified as either ‘faithful’ and devoid of errors or ‘unfaithful’ with discernible errors – leverages annotated medical terms to refine existing medical text summarization tools.
Distinctively, FaMeSumm eschews a simplistic word-matching approach in favor of fine-tuning pre-trained language models to rectify errors and ensure precise summarization, particularly concerning medical terminology. Zhang emphasized the importance of preserving the intended meaning of medical terms, including nuanced qualifiers like “no,” “not,” or “none,” to minimize inaccuracies.
The efficacy of FaMeSumm was validated across diverse training datasets encompassing clinicians’ notes and intricate patient inquiries, consistently demonstrating enhanced faithfulness in summarization outputs. Medical professionals corroborated these findings, affirming the utility of FaMeSumm in improving the reliability of generated summaries.
Furthermore, the study underscores the potential of fine-tuned large language models (LLMs) in revolutionizing healthcare practices. Comparative analysis revealed the superior performance of the fine-tuned models over prominent models like GPT-3, indicating promising avenues for their integration into medical summarization workflows.
Looking ahead, Zhang envisions a future where AI-driven models streamline the generation of medical summaries, potentially reducing the burden on healthcare professionals who may only need to perform minor edits for validation. This transformative shift holds the promise of significantly expediting the summary creation process while upholding standards of accuracy and reliability.
Beyond medical summarization, the study resonates with broader efforts to harness generative AI and LLMs for augmenting clinical decision-support tools. Recent research from the New York Eye and Ear Infirmary of Mount Sinai (NYEE) showcased the proficiency of OpenAI’s Generative Pre-Training–Model 4 (GPT-4) in matching or surpassing ophthalmologists in managing complex cases of glaucoma and retinal conditions. In a specialty characterized by high patient volumes and intricate case management, AI-driven solutions offer transformative potential in enhancing patient care delivery.
In essence, the development of FaMeSumm represents a significant stride in advancing the field of medical text summarization. Through meticulous analysis and fine-tuning of language models, FaMeSumm demonstrates a tangible improvement in the faithfulness and accuracy of generated summaries. Moreover, its versatility across diverse medical datasets underscores its potential as a robust solution for various healthcare contexts. As the healthcare industry continues to embrace AI-driven innovations, frameworks like FaMeSumm offer promising avenues for enhancing clinical decision support systems and optimizing patient care delivery. With further refinement and integration, FaMeSumm stands poised to revolutionize medical summarization practices, ushering in an era of more efficient and reliable information synthesis in healthcare settings.