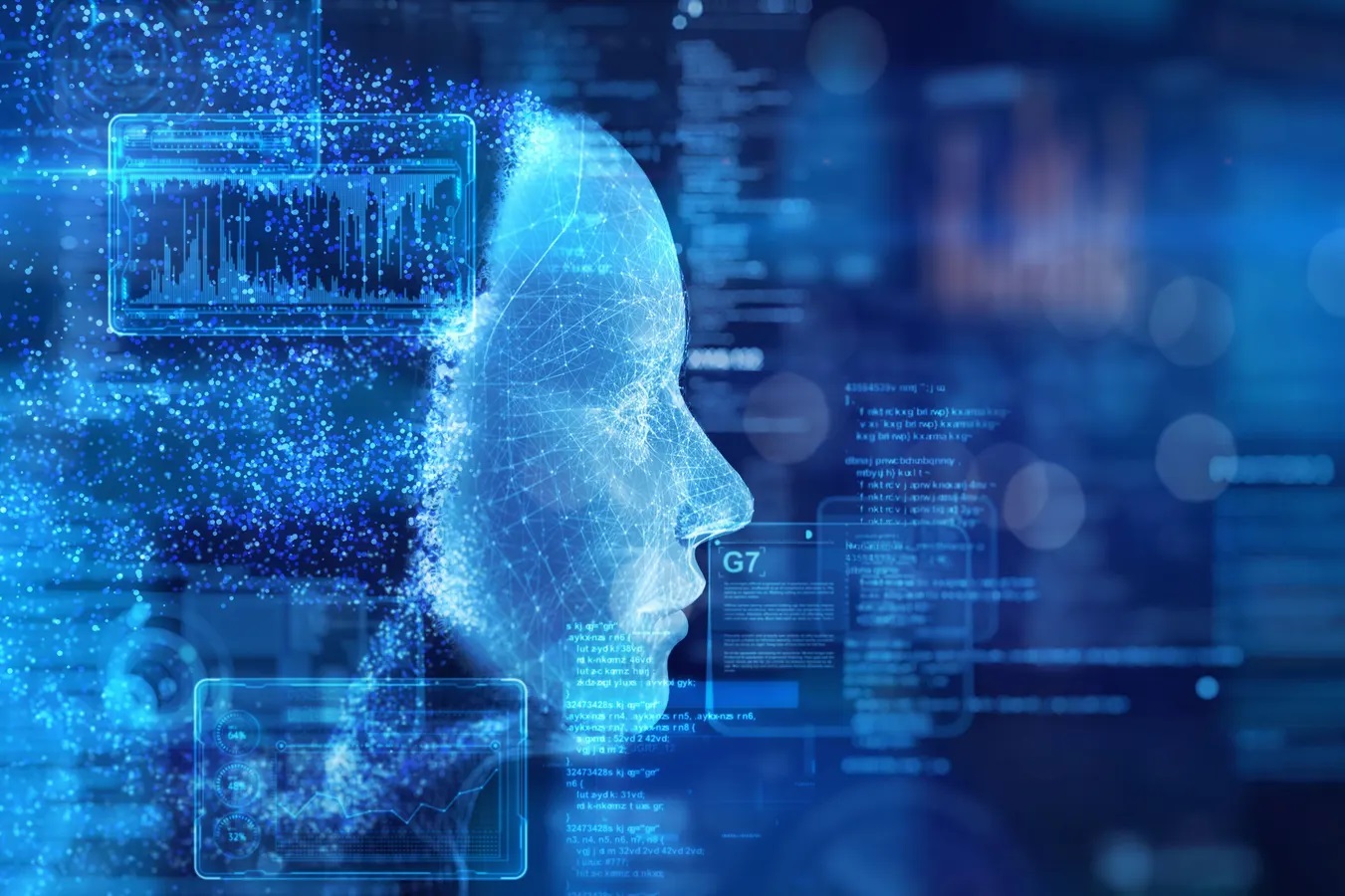
Machine learning is revolutionizing pediatric medicine, as evidenced by a novel tool developed by Boston Children’s Hospital researchers. This tool utilizes ultrasound findings to predict the risk of dilating vesicoureteral reflux (VUR) in infants with hydronephrosis. By leveraging data from 280 infants, a sophisticated machine learning algorithm achieved high accuracy in identifying VUR risk factors. This breakthrough offers clinicians a practical and intuitive tool for patient management, potentially reducing unnecessary testing and improving outcomes. Coupled with recent advancements such as an AI-driven smartphone application for diagnosing acute otitis media, these innovations underscore the transformative impact of AI in pediatric healthcare.
In the realm of pediatric medicine, technological advancements have opened new avenues for improving patient care. Notably, machine learning has emerged as a powerful tool, enabling researchers to tackle complex challenges in diagnosing and managing pediatric conditions. One such challenge is the accurate prediction of dilating vesicoureteral reflux (VUR) in infants with hydronephrosis, a condition that can lead to serious urinary complications. Traditional screening methods have limitations, prompting researchers from Boston Children’s Hospital to develop a novel machine-learning model. This model, based on ultrasound findings and patient data, aims to identify infants at high risk of VUR, facilitating targeted screening and informed clinical decisions. This introduction sets the stage for exploring the development and implications of this groundbreaking technology in pediatric urinary health.
Machine learning, the cutting-edge technology that allows computers to learn from data and make predictions, is revolutionizing the field of pediatric medicine. In a groundbreaking development, researchers from Boston Children’s Hospital have leveraged machine learning to predict the risk of dilating vesicoureteral reflux (VUR) in infants with hydronephrosis, a condition characterized by the backup of urine into the kidney.
Hydronephrosis is often detected through prenatal ultrasound screenings, but these tests have limitations in identifying dilating VUR, a major contributor to the condition. While a voiding cystourethrogram (VCUG) can provide more accurate results, it is invasive and costly. To address these challenges, the research team embarked on a mission to create a tool capable of utilizing ultrasound findings to identify infants at the highest risk of VUR, thereby facilitating targeted screening via VCUG.
In the realm of pediatric urology, assessing infants for hydronephrosis involves relying on a standardized system to classify urinary tract dilation (UTD) based on ultrasound findings before and after birth. While this approach helps gauge the severity of UTD by assessing kidney dilation, it falls short of providing a specific diagnosis or predicting patient outcomes.
To bridge this gap, the researchers developed a sophisticated machine-learning algorithm using data from 280 infants who underwent ultrasounds for hydronephrosis followed by VCUGs to confirm VUR at Boston Children’s Hospital. This algorithm incorporates imaging information, patient demographics, and UTD classification to predict the risk of dilating VUR in infants with hydronephrosis. Impressively, the final model exhibited high performance, boasting an area under the curve of 0.81.
Upon analysis, several factors emerged as significant predictors of VUR in the cohort, including gender, ureteral dilation, parenchymal thickness, parenchymal appearance, and central calyceal dilation. The researchers emphasize that their model not only achieves accuracy but also offers intuitive interpretability, making it readily usable by clinicians in routine clinical settings for counseling patients on the next steps in managing hydronephrosis.
Dr. Hsin-Hsiao (Scott) Wang, the lead author of the study and a urologist at Boston Children’s Hospital, highlighted the practicality of the model, stating that it can be easily reviewed and implemented in clinical practice, thereby streamlining decision-making processes for patient management.
One notable aspect of this research is its potential to be the first of its kind in predicting dilating VUR risk using early post-natal ultrasound findings. Looking ahead, the researchers aim to enhance the model by incorporating data from 15,000 patient records, with the ultimate goal of predicting whether a patient’s hydronephrosis will resolve spontaneously or necessitate medical intervention.
Dr. Wang expressed optimism about the future of pediatric urology, envisioning a scenario where unnecessary testing can be minimized through the use of predictive models. This sentiment resonates with the broader trend of utilizing artificial intelligence (AI) to enhance care for pediatric conditions.
In a recent development from the University of Pittsburgh (Pitt) and UPMC, researchers unveiled an AI-driven smartphone application designed to diagnose acute otitis media (AOM) in pediatric primary care settings. AOM and other ear infections are prevalent among pediatric patients, but accurately distinguishing between them is crucial for appropriate treatment. Unlike some ear infections, AOM requires antibiotics for resolution.
However, identifying AOM can be challenging, leading to misdiagnosis in primary care settings. To address this issue, the researchers developed a smartphone application capable of analyzing short videos of the eardrum captured using an otoscope. By detecting subtle visual cues, the application aids clinicians in diagnosing AOM accurately.