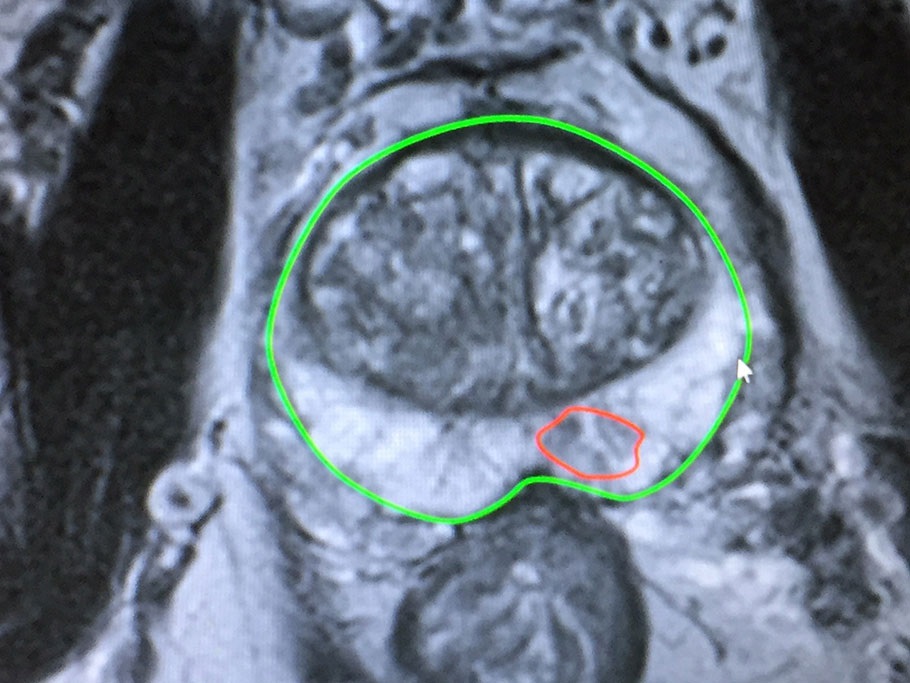
Cutting-edge MRI-based models show promise in prostate cancer risk prediction but may underestimate risk in specific cohorts. Evaluating four models across European, North American, and biomarker-based cohorts revealed variations in performance. While all models demonstrated good discrimination in European populations, calibration differences were noted in North American groups. The need for tailored risk models incorporating advanced biomarkers is evident to address underestimation tendencies. Additionally, advancements in precision medicine, exemplified by deep learning models for predicting brain metastasis in non-small cell lung cancer, signify a broader paradigm shift towards personalized oncology care.
MRI-based risk prediction models offer a positive outlook for prostate cancer detection, potentially enhancing traditional diagnostic tools. However, their efficacy across diverse cohorts remains underexplored. This study aims to fill this gap by comparing the performance of four prominent MRI-based models across European, North American, and biomarker-dependent cohorts. Understanding the strengths and limitations of these models is crucial for optimizing prostate cancer screening and management strategies.
Magnetic Resonance Imaging (MRI) has emerged as a promising tool for predicting prostate cancer risk. Recent research published in JAMA Network Open sheds light on the performance of MRI-based risk calculators across different cohorts. While these calculators show promise in European and North American populations, they exhibit limitations, particularly in underestimating risk in advanced serum biomarker cohorts.
Research Overview
The study aimed to compare the efficacy of four MRI-based risk calculators in predicting clinically significant prostate cancer risk across three distinct cohorts. These cohorts comprised European and North American patients who underwent MRI before biopsy, as well as a cohort reliant on the Prostate Health Index (PHI) as an advanced serum biomarker before MRI or biopsy.
Comparative Analysis of MRI-Based Models
The four evaluated models include the Prospective Loyola University Multiparametric MRI (PLUM) tool, the University of California, Los Angeles (UCLA)-Cornell model, the Van Leeuwen model, and the Rotterdam Prostate Cancer Risk Calculator–MRI (RPCRC-MRI).
Performance in European and North American Cohorts
In European cohorts, all models exhibited good diagnostic discrimination, with an impressive Area Under the Curve (AUC) of 0.90. However, performance varied slightly when applied to North American patients, with AUCs ranging from 0.83 to 0.85. Notably, the RPCRC-MRI and PLUM models demonstrated superior calibration in this cohort.
Underestimation in PHI Cohort
Across all models, an underestimation of clinically significant prostate cancer risk was observed in the PHI cohort. Despite this, both the UCLA-Cornell and PLUM models displayed better calibration and discrimination, with AUCs of 0.83 and 0.82, respectively.
Decision Curve Analysis
While each model provided comparable net benefits in the European cohort, the PLUM and RPCRC-MRI models offered higher benefits in the North American cohort. The UCLA-Cornell model exhibited the highest net benefit in the PHI cohort.
Implications and Future Directions
The study underscores the potential of RPCRC-MRI and PLUM models in MRI-based prostate cancer screening pathways. However, it highlights the necessity for tailored risk models specific to screening pathways incorporating advanced biomarkers to address the tendency of MRI-based models to underpredict risk in certain cohorts.
Advancements in Precision Medicine
Beyond prostate cancer, recent developments in data analytics and precision medicine are revolutionizing risk prediction models in oncology. For instance, researchers at Washington University School of Medicine have pioneered a deep-learning approach to predict brain metastasis in patients with non-small cell lung cancer (NSCLC).
Deep Learning in NSCLC
Brain metastases pose a significant challenge in NSCLC management, yet no effective method exists for identifying at-risk patients. Leveraging lung biopsy images, the deep learning model developed by the research team outperformed expert pathologists in identifying abnormal features associated with brain metastasis.
The findings underscore the potential of MRI-based models in prostate cancer risk assessment, particularly in European populations. However, discrepancies in performance across cohorts highlight the necessity for tailored risk prediction tools incorporating advanced biomarkers. The emergence of deep learning models for predicting brain metastasis in non-small cell lung cancer signifies a broader trend towards personalized oncology care, emphasizing the transformative impact of precision medicine in cancer management.