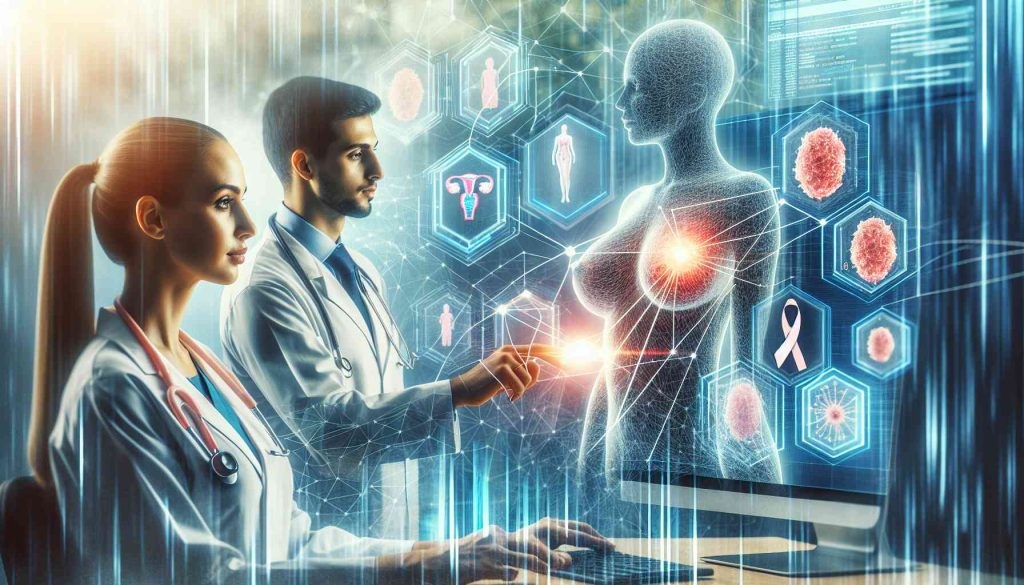
AI-driven predictive models, like Northwestern University’s innovative tool, are transforming breast cancer care. Utilizing cell patterns, these tools forecast disease progression, potentially sparing patients from unnecessary chemotherapy. The AI assesses 26 properties of breast tissue, delivering comprehensive prognostic scores and aiding in personalized treatment planning. Its broader implications encompass refining risk assessments and monitoring treatment responses, bridging the gap in cancer care for patients in community settings. These advancements align with recent AI strides in predicting cancer outcomes, such as UCLA’s epigenetic factor-based model, heralding a new era of precision medicine in oncology.
Northwestern University’s groundbreaking AI tool in breast cancer care signifies a paradigm shift. Addressing complexities in evaluating cancer cell appearances, this AI model leverages cell patterns to predict disease courses. It offers a comprehensive evaluation of breast tissue properties, empowering clinicians to devise personalized treatment plans. Beyond prognosis, it holds promise in refining risk assessments and monitoring treatment responses, potentially reshaping cancer care in diverse healthcare settings. This innovation aligns with recent AI advancements, like UCLA’s model using epigenetic factors, signifying a transformative trajectory toward precision oncology.
AI-Powered Breast Cancer Tool: Revolutionizing Treatment Precision
Breast cancer, affecting one in eight American women, constitutes nearly 30% of all new female cancers annually. Conventionally diagnosed through imaging or biopsy, determining its stage and grade guides treatment decisions. However, evaluating cancer cell appearances remains challenging for clinicians, prompting the exploration of non-cancerous cells’ role in disease progression.
Recognizing these complexities, the Northwestern team aimed to create an AI model capable of analyzing digital breast tissue images, discerning cancerous and non-cancerous cell characteristics, and understanding their interactions.
Dr. Lee Cooper, the study’s lead author and an associate professor at Northwestern University Feinberg School of Medicine, emphasized the AI’s role: “The AI model measures these patterns and presents information to the pathologist in a way that makes the AI decision-making process clear to the pathologist.”
By assessing 26 properties of breast tissue, the AI delivers an overall prognostic score. Additionally, it generates scores for individual cancer, immune, and stromal cells, aiding pathologists in devising personalized treatment plans for patients.
Moreover, the tool’s potential extends beyond prognosis and treatment planning. It could enhance risk assessments for individual cases and monitor treatment responses, potentially enabling adjustments in chemotherapy intensity or duration.
Crucially, this AI model might bridge the gap in evaluating breast cancer for patients in community settings lacking specialized pathologists. Cooper envisions its utility in assisting generalist pathologists tasked with assessing breast cancers.
The researchers aim to validate this model for clinical use and develop AI models for diverse breast cancer types, like triple-negative or HER2-positive, acknowledging variations in tissue patterns across cancers. Dr. Cooper highlighted, “This will improve our ability to predict outcomes and will provide further insights into the biology of breast cancers.”
This groundbreaking work aligns with recent AI advancements in predicting cancer outcomes. UCLA Health’s Jonsson Comprehensive Cancer Center unveiled an AI model utilizing epigenetic factors to predict cancer survival outcomes. Evaluating gene expression patterns in tumors allowed the categorization of these factors, empowering the AI to forecast outcomes across various cancer types.
The model’s predictive accuracy surpassed traditional metrics like cancer grade and stage, showcasing its potential to enhance prognostic accuracy.
AI-driven tools are redefining breast cancer care, exemplified by Northwestern’s predictive model. These advancements herald a new era by enhancing treatment personalization, prognostic accuracy, and monitoring responses. Beyond specialized settings, they promise to bridge care disparities across different healthcare setups, impacting patient outcomes. Parallel strides in AI, like UCLA’s epigenetic factor-based model, further cement the trajectory towards precision medicine in oncology. As these models continue evolving, the landscape of cancer care is poised for transformation, offering unprecedented avenues for individualized treatments and improved patient prognosis.