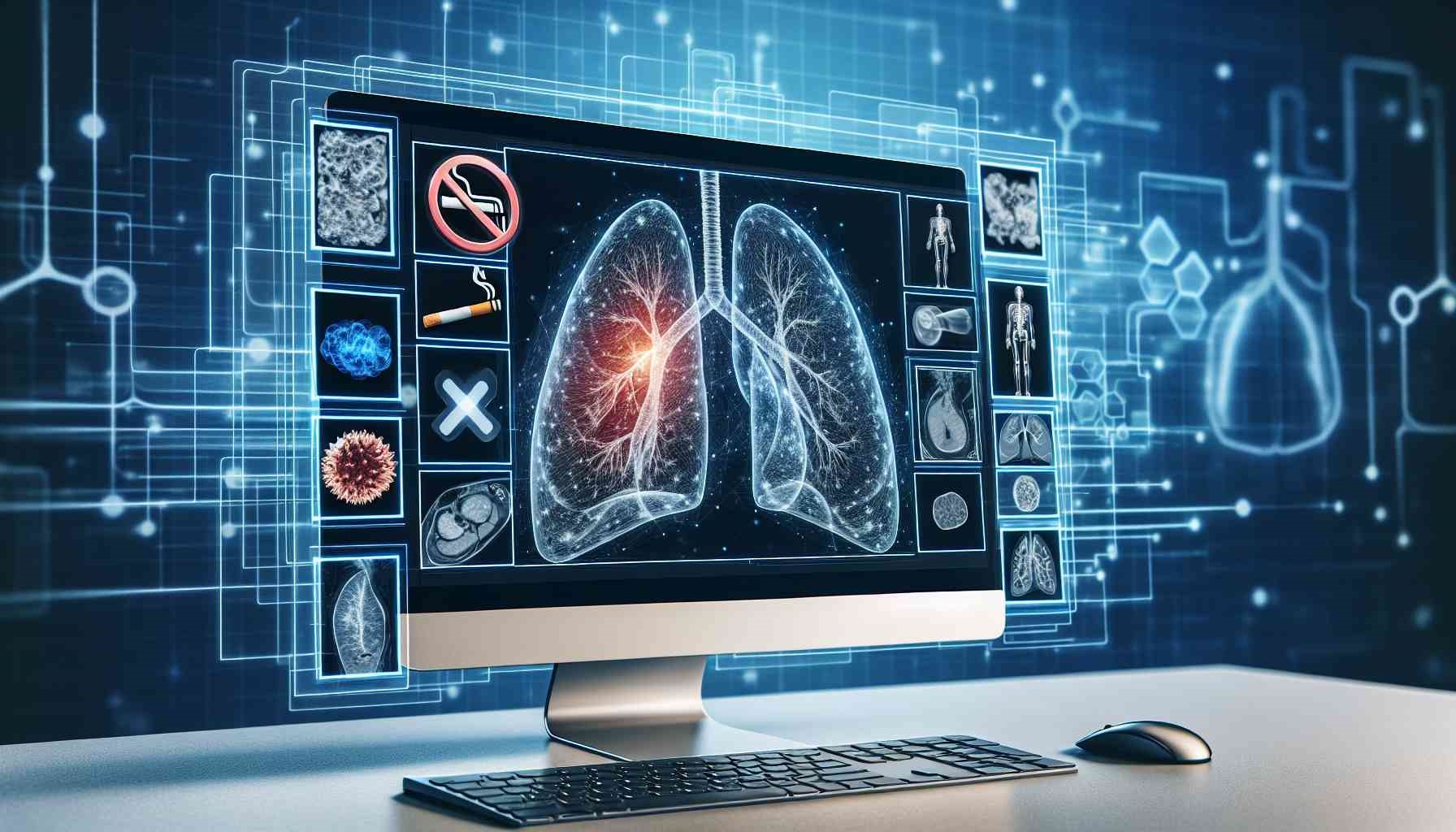
A deep learning model, CXR-Lung-Risk, harnesses routine chest X-rays to identify high-risk never-smokers for lung cancer. Developed and validated using extensive datasets, it accurately predicted lung cancer occurrence, aiding early detection. High-risk individuals, flagged by the model, demonstrated a 2.1 times higher likelihood of developing lung cancer. This groundbreaking approach provides a feasible screening method for non-smokers, filling the void in current guidelines and highlighting AI’s potential in revolutionizing early detection.
Lung cancer, a predominant threat, disproportionately impacts non-smokers, necessitating innovative screening approaches. Current guidelines focus on smokers, overlooking the rising incidence among never-smokers. Enter CXR-Lung-Risk, a pioneering AI model utilizing routine chest X-rays to accurately identify high-risk individuals. With a surge in advanced-stage diagnoses among never-smokers, this AI-powered approach stands poised to redefine screening protocols, catering to an overlooked demographic. Its simplicity and efficacy challenge conventional norms, signaling a paradigm shift in early lung cancer detection.
Revolutionizing Lung Cancer Detection for Non-Smokers
In a groundbreaking development presented at the Radiological Society of North America (RSNA) conference, a deep learning model has emerged as a game-changer in the early detection of lung cancer among non-smokers. This innovative model showcases a remarkable ability to pinpoint individuals at high risk of lung cancer solely through routine chest X-rays.
Lung cancer stands as the third most prevalent cancer in the United States, claiming more lives than any other cancer type, according to the Centers for Disease Control and Prevention (CDC). Historically, smoking has been identified as a primary cause of this disease, attributing to roughly 80 percent of lung cancer fatalities, especially in cases like small cell lung cancer (SCLC).
However, a notable revelation from research highlights that around 10 to 20 percent of lung cancer cases arise in individuals classified as “never-smokers,” comprising those who have either never smoked or have smoked minimally. Alarmingly, the incidence of lung cancer among this demographic is escalating, often leading to the detection of more advanced cancers compared to non-smokers.
While prevailing guidelines from the United States Preventive Services Task Force (USPSTF) advocate for lung cancer screening through low-dose computed tomography (LDCT) for individuals with substantial smoking histories, there remains a critical gap in screening for never-smokers.
Anika S. Walia, the lead author of the study and a medical student at Boston University School of Medicine, emphasized the urgency to reevaluate screening strategies: “Current recommendations overlook the rising cases of lung cancer among never-smokers, often diagnosed in advanced stages.”
To bridge this gap, researchers delved into the realm of artificial intelligence (AI) to devise an innovative risk prediction model, termed CXR-Lung-Risk. Leveraging electronic health record (EHR) chest X-ray images, this deep-learning model identifies intricate patterns associated with lung cancer, effectively stratifying patients into risk groups.
Walia highlighted the model’s simplicity and accessibility: “Our approach relies on a single chest X-ray image, a routine and readily available medical test, making it highly feasible for implementation.”
Developed using 147,497 chest X-rays from the Prostate, Lung, Colorectal, and Ovarian (PLCO) cancer screening trial, the model underwent external validation on a distinct cohort of never-smokers who underwent outpatient chest X-rays. Intriguingly, the tool successfully flagged 28 percent of participants as high risk, with 2.9 percent of them subsequently diagnosed with lung cancer—exceeding the risk threshold prompting screening.
Upon statistical adjustments considering various demographic and medical factors, non-smokers categorized as high-risk by the model exhibited a 2.1 times higher likelihood of developing lung cancer compared to their low-risk counterparts.
Senior author Michael T. Lu, MD, MPH, emphasized the significance of this AI tool in revolutionizing early detection: “This model enables targeted screening for high-risk never-smokers, utilizing existing chest X-rays in the electronic health record, a critical advancement in the wake of declining smoking rates.”
The potential of deep learning in identifying candidates suitable for lung cancer screening has surfaced in recent research. Earlier models have shown promise in predicting lung cancer risk, flagging eligible individuals for screening based on Medicare guidelines while also identifying those who fall outside the criteria but could benefit from screening.
The team behind this research aimed to address the limitations of current CMS guidelines, acknowledging that a substantial number of lung cancer cases occur in individuals not meeting eligibility criteria. Their solution involved creating an automated risk assessment tool tailored to complement the existing CMS criteria.
The advent of CXR-Lung-Risk signifies a breakthrough in lung cancer screening, particularly for non-smokers. Its ability to accurately predict risk using routine chest X-rays presents a game-changing opportunity for early detection. By addressing the shortcomings of existing guidelines, this AI model offers a viable solution to bridge the gap in screening protocols. The observed higher incidence of lung cancer among never-smokers demands tailored approaches, and this AI-driven innovation serves as a beacon of hope in reshaping screening strategies, ultimately fostering timely interventions and improved outcomes in combating lung cancer.