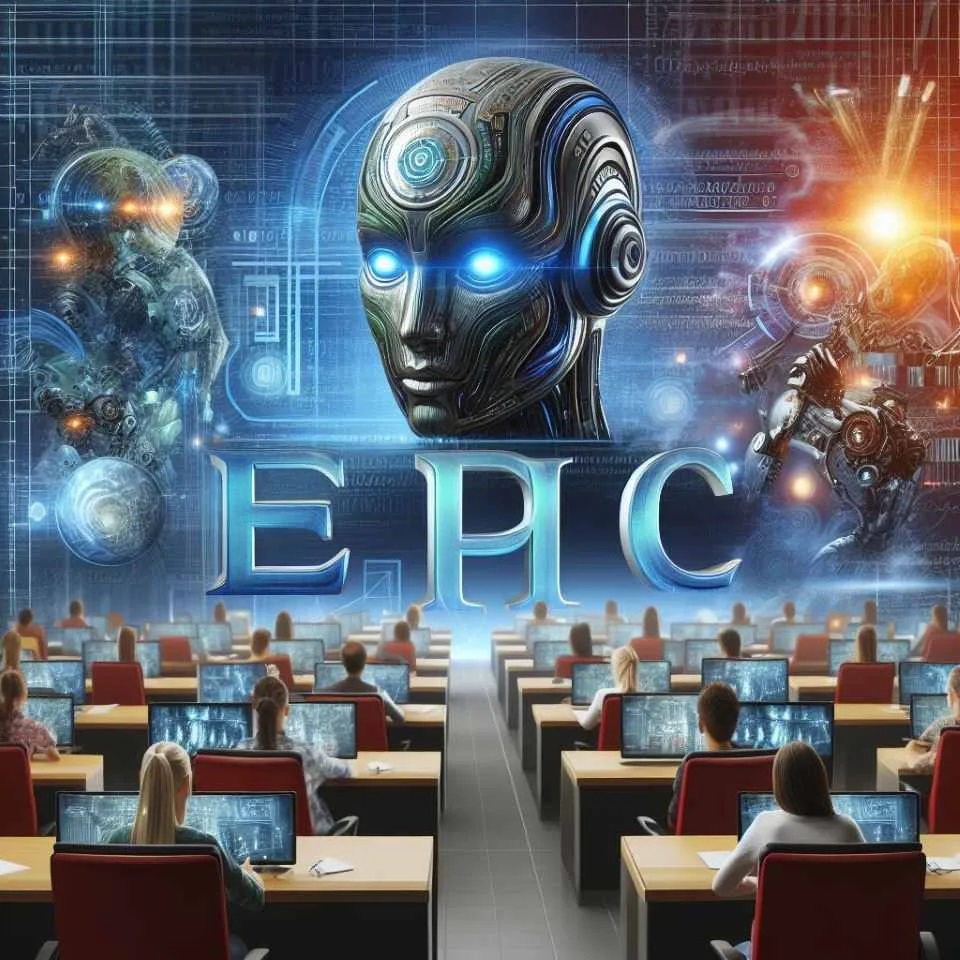
Delving into the efficacy of sepsis prediction models like the Epic Sepsis Model (ESM), recent research from the University of Michigan sheds light on critical limitations. Through a comprehensive analysis of over 77,000 adult inpatients, the study reveals that the timing of data collection significantly impacts the predictive accuracy of the ESM. Furthermore, the study emphasizes the importance of aligning predictive models with clinical workflows to maximize their utility in real-world settings. Despite challenges, ongoing efforts, such as the development of advanced AI-driven approaches like COMPOSER, offer promising avenues for improving sepsis management and patient outcomes.
In recent years, the medical community has witnessed a surge in the development and deployment of artificial intelligence (AI) tools aimed at predicting the onset of sepsis—a life-threatening condition resulting from the body’s extreme response to an infection. Among these tools, the Epic Sepsis Model (ESM) has gained significant attention due to its integration within the widely used Epic electronic health record (EHR) system. However, the clinical utility and accuracy of such predictive models have come under scrutiny, with recent studies shedding light on their limitations.
A groundbreaking study conducted by researchers at the University of Michigan has brought to light crucial insights regarding the efficacy of the Epic Sepsis Model. Published in the esteemed New England Journal of Medicine (NEJM) AI, the study reveals that while the ESM holds promise in identifying high-risk patients, its predictive accuracy may be significantly hindered by certain factors, particularly the timing of data collection in relation to the onset of sepsis.
The research team, led by Jenna Wiens, PhD, and her colleagues, embarked on a comprehensive analysis using data from over 77,000 adult inpatients hospitalized at the University of Michigan Health system between 2018 and 2020. Their objective was to evaluate the performance of the ESM in predicting sepsis onset across different stages of patients’ hospitalization and treatment.
One of the key findings of the study was the substantial impact of the timing of data collection on the predictive accuracy of the ESM. When considering predictions made both before and after clinical recognition of sepsis, the model exhibited an area under the receiver operating characteristic curve (AUROC) of 0.62—an indication of moderate predictive performance. However, when restricting predictions to data collected strictly before sepsis criteria were met, the AUROC plummeted to 0.47, suggesting a significant decrease in predictive accuracy.
Furthermore, the study highlighted the importance of considering the clinical workflow in evaluating the utility of predictive models such as the ESM. Donna Tjandra, a doctoral student involved in the research, emphasized that assessing the model’s performance with data collected after clinicians had already suspected sepsis might artificially inflate its apparent effectiveness. Such an approach fails to align with the practical needs of clinicians in real-world settings, where early and accurate prediction of sepsis is paramount for timely intervention and improved patient outcomes.
The findings of the University of Michigan study underscore a critical challenge facing sepsis prediction models—the risk of incorporating information that reflects clinicians’ suspicions rather than objectively predicting sepsis onset based on patient data alone. This phenomenon raises questions about the true clinical utility of such models and calls for a reevaluation of their design and implementation strategies.
While the Epic Sepsis Model has faced scrutiny for its limitations, efforts are underway to develop more robust and effective predictive tools. For instance, researchers at the University of California (UC) San Diego School of Medicine have recently introduced an AI model known as COMPOSER, designed to continuously monitor patients in emergency departments for early signs of sepsis.
Unlike traditional models, COMPOSER leverages deep learning algorithms to analyze real-time data from various sources, including laboratory results and patient demographics, enabling it to accurately predict sepsis onset. Upon identifying high-risk patients, the system promptly alerts care teams through the EHR system, facilitating timely intervention.
Since its implementation, COMPOSER has demonstrated remarkable success, leading to a notable 17 percent reduction in sepsis-related mortality in emergency department settings. This encouraging outcome underscores the potential of advanced AI-driven approaches in revolutionizing sepsis management and improving patient outcomes.
The findings of the University of Michigan study underscore the pressing need for continued research and innovation in the realm of sepsis prediction models. While the Epic Sepsis Model and similar tools hold promise in identifying high-risk patients, their effectiveness may be hindered by factors such as the timing of data collection and the integration of clinician suspicions. Moving forward, efforts to develop more robust and clinically relevant predictive models, exemplified by initiatives like COMPOSER, offer hope for enhancing sepsis management and ultimately improving patient outcomes.