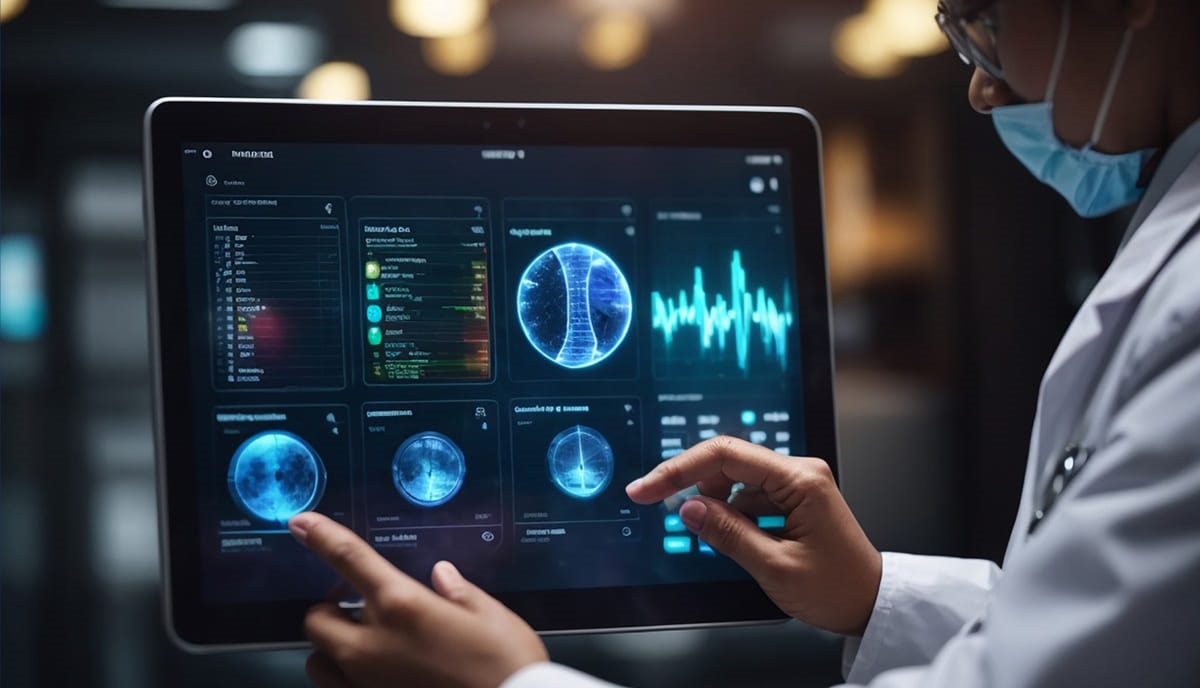
The study evaluates the efficacy of a predictive tool, the SMART Choice (Knee) model, in informing patients’ decisions regarding total knee arthroplasty (TKA). Conducted among individuals with knee osteoarthritis, the research finds that while the tool provides individualized information, it does not significantly alter patients’ willingness for surgery. This highlights the challenges in integrating predictive analytics into clinical practice and emphasizes the necessity for continued research to optimize tool design and enhance its utility in healthcare decision-making processes.
The integration of predictive analytics into healthcare decision-making presents both opportunities and challenges. Recent research delves into the impact of predictive tools, such as the SMART Choice (Knee) model, on patients’ decisions regarding total knee arthroplasty (TKA). Despite expectations, findings reveal minimal influence on patients’ willingness for surgery. This highlights the complexities of implementing predictive analytics in orthopedic contexts and underscores the need for further investigation. As healthcare embraces data-driven approaches, understanding the nuances of predictive tools is paramount for enhancing patient-centered care.
In recent years, predictive analytics has emerged as a promising tool in healthcare decision-making, aiming to forecast outcomes and personalize treatment approaches. However, its effectiveness in influencing patient decisions, particularly regarding surgical interventions such as total knee arthroplasty (TKA), remains an area of active investigation. A recent study published in JAMA Network Open sheds light on the role of predictive tools in informing patients’ willingness to undergo TKA, emphasizing the need for further research and optimization in this domain.
Understanding the Study:
The study, conducted by a team of researchers, aimed to evaluate the impact of a predictive model, the SMART Choice (Knee) tool, on patients’ willingness to undergo TKA. This randomized clinical trial (RCT) involved 211 patients with unilateral knee osteoarthritis who had previously attempted non-surgical interventions. The intervention group (105 patients) was provided access to the SMART Choice tool, while the control group received standard treatment without access to the predictive tool. Over a six-six participants’ willingness for surgery, treatment preferences, and decision-making processes were assessed.
Key Findings:
Contrary to expectations, the study found that the utilization of the predictive tool did not significantly alter patients’ willingness to undergo TKA. Even after adjusting for baseline differences, the tool’s impact on surgical decision-making was minimal. These findings highlight the complexities involved in integrating predictive analytics into clinical practice, particularly in orthopedic settings. While predictive tools hold promise in providing individualized information and improving health outcomes, their effectiveness in influencing patient decisions warrants further investigation.
Implications and Future Directions:
Despite the lack of immediate impact on surgical decisions, the researchers suggest that predictive tools could still offer benefits in managing knee osteoarthritis. They emphasize the need for continued research to optimize tool design, address limitations, and understand their role in the decision-making process. This study underscores the importance of a nuanced approach to integrating predictive analytics into healthcare, acknowledging both its potential and current limitations.
Expanding Applications of Predictive Analytics:
Beyond knee surgery, predictive analytics demonstrates potential in various healthcare domains. Recent research from the University of Buffalo highlights its application in predicting outcomes of bariatric surgery. By analyzing psychosocial and genetic factors, a predictive tool originally developed for assessing addiction risk accurately predicted post-surgical outcomes in patients undergoing bariatric procedures. Identifying genetic predispositions and tailoring interventions based on predictive insights could enhance treatment efficacy and mitigate adverse outcomes in this population.
The study on predictive tool use in knee surgery decision-making underscores the evolving landscape of healthcare decision support systems. While predictive analytics holds promise in optimizing treatment strategies and improving patient outcomes, its integration into clinical practice requires careful consideration of its impact and limitations. Continued research efforts are essential to refine predictive models, enhance their usability, and maximize their utility in informing patient-centered care. As healthcare continues to embrace data-driven approaches, understanding the nuances of predictive analytics is crucial for advancing precision medicine and delivering personalized healthcare interventions.