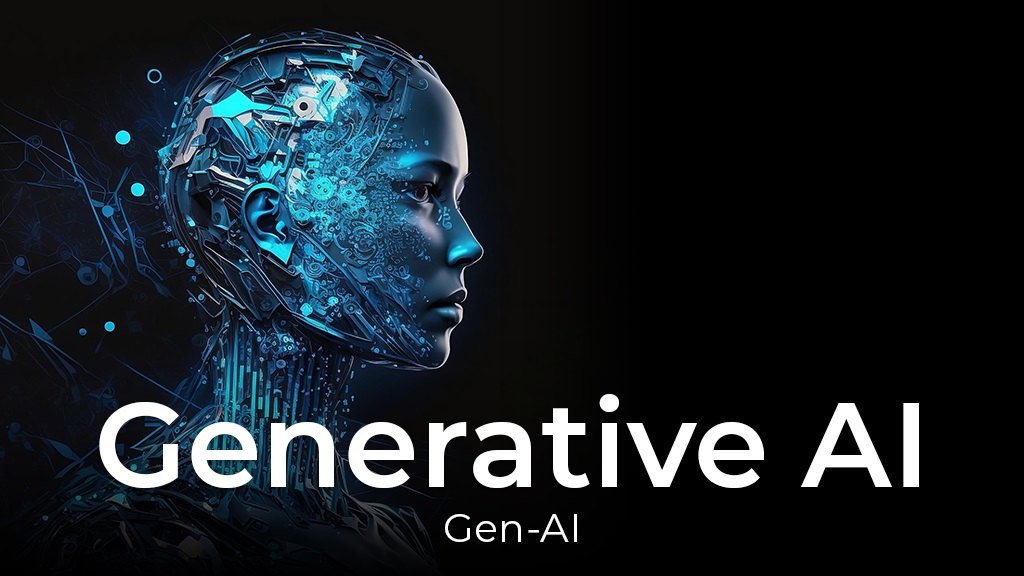
Generative AI emerges as a promising solution for bolstering healthcare-associated infection (HAI) surveillance, offering advanced capabilities for early detection and intervention. Recent research evaluates the efficacy of large language models (LLMs) in identifying central line-associated bloodstream infection (CLABSI) and catheter-associated urinary tract infection (CAUTI) cases. While demonstrating promising results, challenges such as ambiguous information underscore the importance of human oversight. The study underscores the potential of generative AI to empower healthcare providers, enhance patient safety, and streamline surveillance efforts.
Healthcare-associated infections (HAIs) pose a significant challenge to patient safety and care delivery worldwide. In light of this, the integration of advanced technologies like generative artificial intelligence (AI) holds promise for augmenting existing surveillance programs. This study examines the efficacy of generative AI-driven tools in detecting instances of CLABSI and CAUTI, aiming to enhance infection surveillance within healthcare settings. By leveraging large language models (LLMs), researchers explore the potential of AI to streamline workflow, improve detection accuracy, and ultimately, mitigate the prevalence of HAIs.
The Role of Generative AI in Healthcare Surveillance
Generative artificial intelligence (AI) emerges as a valuable tool in healthcare-associated infection (HAI) surveillance, offering enhanced capabilities for early detection and intervention. As healthcare providers strive to mitigate the prevalence of HAIs, the integration of AI-driven solutions presents a promising avenue for bolstering existing surveillance efforts.
Addressing the HAI Challenge
HAIs represent a substantial burden on healthcare systems, with statistics revealing alarming prevalence rates among hospitalized patients. Despite advancements in infection prevention protocols, the incidence of HAIs remains a pressing concern, underscoring the need for innovative approaches to surveillance and containment.
Evaluating AI-driven Surveillance Tools
A recent study conducted by researchers from Saint Louis University and the University of Louisville School of Medicine delves into the potential of generative AI in enhancing HAI surveillance programs. The investigation focuses on assessing the efficacy of two large language models (LLMs) – OpenAI’s ChatGPT Plus and an open-source model known as Mixtral 8x7B – in detecting central line-associated bloodstream infection (CLABSI) and catheter-associated urinary tract infection (CAUTI) cases.
Methodology and Findings
The study employs a comprehensive methodology, utilizing clinical scenarios of varying complexities to evaluate the performance of the LLMs. By feeding data from the National Health Care Safety Network dataset into the models, researchers assess their ability to accurately identify instances of CLABSI and CAUTI. While both ChatGPT and Mixtral 8x7B demonstrate promising results in scenario analysis, challenges such as missing or ambiguous information highlight the importance of human oversight in AI-assisted surveillance efforts.
Implications for Healthcare Practice
The findings underscore the potential of generative AI in augmenting HAI surveillance within healthcare settings. Lead author Timothy L. Wiemken emphasizes the significance of continued development and refinement of AI tools, emphasizing the need for real-world patient data to support infection preventionists. With ongoing user education and AI model optimization, these surveillance tools can empower healthcare providers, enabling them to allocate resources more efficiently and focus on patient care.
Leveraging AI for Enhanced Patient Safety
Tania Bubb, President of the Association for Professionals in Infection Control and Epidemiology (APIC), acknowledges the role of AI-powered tools in strengthening surveillance programs. By offering a cost-effective means of improving HAI surveillance, generative AI presents a valuable asset in the arsenal of infection preventionists. The study’s implications extend beyond mere detection, highlighting AI’s potential to streamline healthcare workflows and enhance patient safety outcomes.
Beyond Surveillance: Expanding AI Applications in Healthcare
While infection surveillance represents a critical domain for generative AI, its utility extends to diverse areas within healthcare. Researchers from NYU Langone Health explore alternative applications of AI, demonstrating its capacity to expedite the development of digital health software. By leveraging tools like ChatGPT, healthcare innovators can streamline the design process, accelerating the deployment of interventions such as personalized automatic messaging systems (PAMS) in chronic disease management.
Facilitating Innovation through AI Integration
The integration of AI into healthcare systems heralds a new era of innovation, enabling stakeholders to overcome traditional barriers in software development and implementation. By harnessing the power of generative AI, healthcare organizations can drive efficiencies, improve patient outcomes, and navigate complex challenges with greater agility. As research continues to unveil the potential of AI-driven solutions, the healthcare landscape stands poised for transformation, with generative AI at the forefront of this paradigm shift.
The findings of this study underscore the transformative potential of generative artificial intelligence (AI) in revolutionizing healthcare surveillance. While demonstrating promising results in detecting healthcare-associated infections (HAIs), the study highlights the importance of ongoing refinement and human oversight in AI-assisted surveillance efforts. By leveraging AI-driven tools, healthcare providers can optimize resource allocation, enhance patient safety, and navigate complex challenges with greater efficiency. As the healthcare landscape continues to evolve, the integration of generative AI stands poised to drive innovation, improve outcomes, and ultimately, transform the delivery of care.