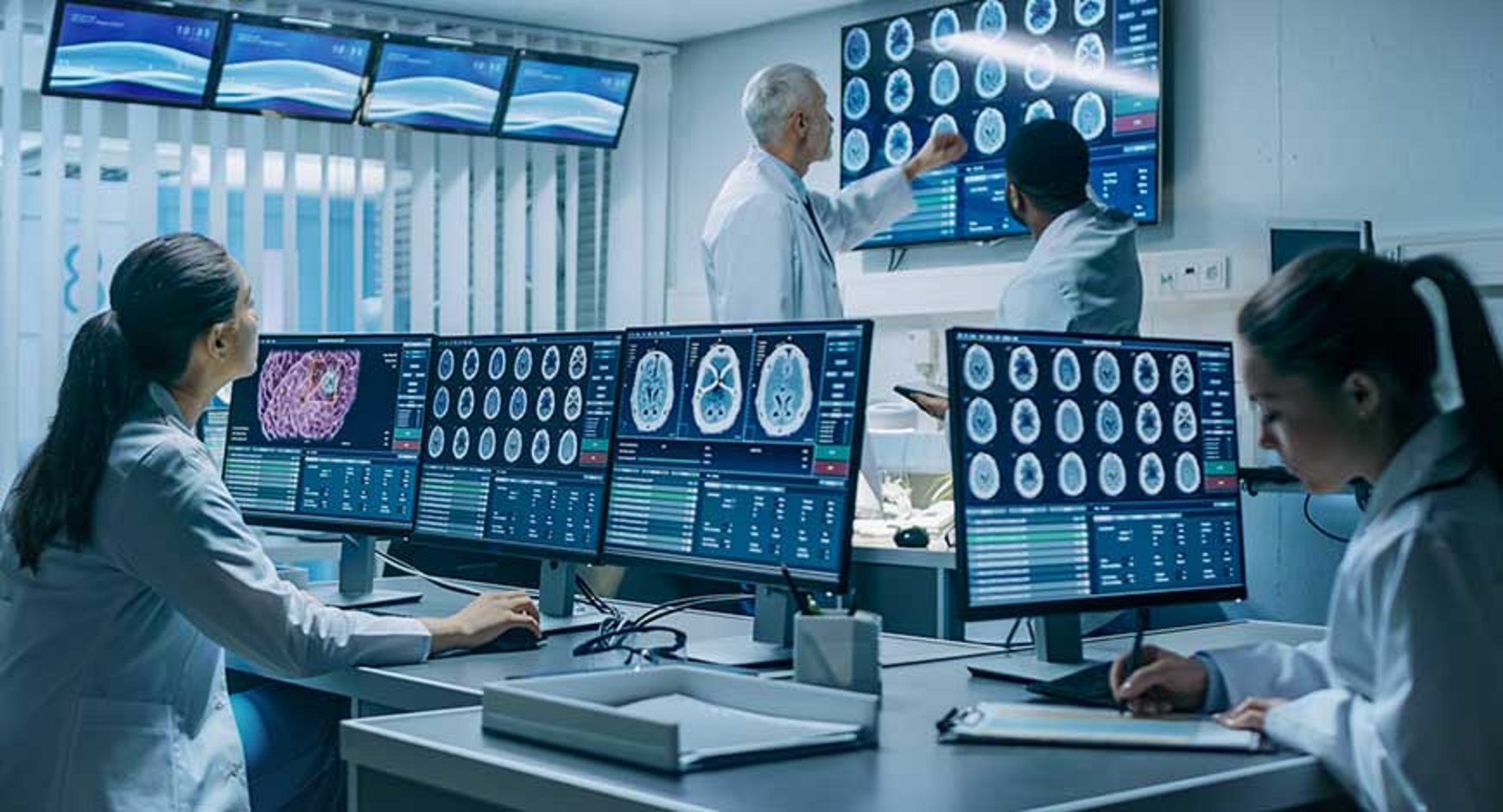
In this study, AI’s influence on radiologist performance was explored, revealing a diverse range of responses among practitioners. While AI tools showed potential to improve diagnostic accuracy, the impact varied significantly across individuals, challenging assumptions about standardized integration. The findings underscore the importance of personalized AI solutions tailored to individual clinician needs. By fostering collaboration between developers and clinicians, healthcare systems can harness AI’s transformative potential while mitigating risks associated with its implementation.
Artificial intelligence (AI) presents unprecedented opportunities to revolutionize radiology, promising enhanced diagnostic capabilities and improved patient outcomes. However, integrating AI into clinical practice poses challenges, particularly regarding its impact on individual radiologist performance. This study aims to unravel the complexities of AI’s influence on clinician decision-making, paving the way for personalized AI solutions that empower radiologists to deliver more accurate and efficient care.
AI’s Varied Impact on Radiologists: A Study Reveals Complex Dynamics
Artificial intelligence (AI) has emerged as a promising tool in healthcare, particularly in assisting radiologists with diagnostic tasks. However, recent research conducted by teams from Harvard Medical School (HMS), the Massachusetts Institute of Technology (MIT), and Stanford University sheds light on the unpredictable effects of AI on radiologist performance. While AI can potentially enhance diagnostic accuracy, its impact varies significantly among radiologists. This raises crucial questions regarding the standardized integration of AI tools in healthcare settings.
Study Overview
Published in Nature Medicine, the study examined how AI-based assistive tools influenced the performance of 140 radiologists across 15 diagnostic tasks involving chest X-ray analysis. The researchers assessed radiologists’ ability to identify abnormalities in patient cases, both with and without AI assistance. Surprisingly, the results unveiled a diverse range of responses to AI intervention, with performance improvements observed in some radiologists while others experienced worsened outcomes.
Unanticipated Findings
Contrary to initial expectations, the study revealed that factors such as specialty area, years of practice, and prior exposure to AI did not reliably predict the impact of AI on individual performance. Notably, lower-performing radiologists did not universally benefit from AI assistance. While some exhibited enhanced performance, many saw their diagnostic accuracy decline, while others experienced no significant change. Conversely, higher-performing radiologists maintained consistent accuracy levels irrespective of AI assistance.
Implications for Healthcare AI
The study highlighted the necessity for comprehensive testing and validation of AI tools before their integration into clinical practice. Moreover, it emphasized the complexity of the machine-human interaction in medical decision-making. Dr. Nikhil Agarwal, co-senior author of the study, emphasized the need to discernmanyde of factors influencing this interaction to ensure optimal patient care.
Personalized AI Integration
Acknowledging the diverse expertise, experience, and decision-making styles among clinicians, the researchers underscored the importance of personalized AI systems. They advocated for collaborative efforts between developers and clinicians to tailor AI tools according to individual needs and preferences. By accommodating variability in clinician capabilities, AI integration can maximize benefits and minimize potential harms.
Future Directions
As AI continues to evolve in healthcare, addressing questions regarding its impact on clinician performance remains paramount. The study’s findings prompt further investigation into understanding the intricate dynamics of human-AI interaction. By unraveling these complexities, healthcare practitioners can harness the full potential of AI while mitigating risks associated with its implementation.
Broader Implications
The discussion surrounding AI’s influence on clinician behavior extends beyond individual performance assessments. Healthcare institutions must grapple with concerns regarding clinician dependence on AI as technology advances. Collaborative efforts between academia, healthcare providers, and industry stakeholders are essential in shaping policies and practices that promote responsible AI utilization in patient care.
As the healthcare landscape continues to evolve, the integration of AI into radiology holds immense promise for enhancing diagnostic accuracy and streamlining workflow processes. However, realizing the full potential of AI requires a nuanced understanding of its impact on individual clinician performance. By fostering collaboration between developers, clinicians, and healthcare institutions, personalized AI solutions can be developed to meet the diverse needs of radiologists, ultimately improving patient care and advancing the field of radiology into a new era of innovation and excellence.