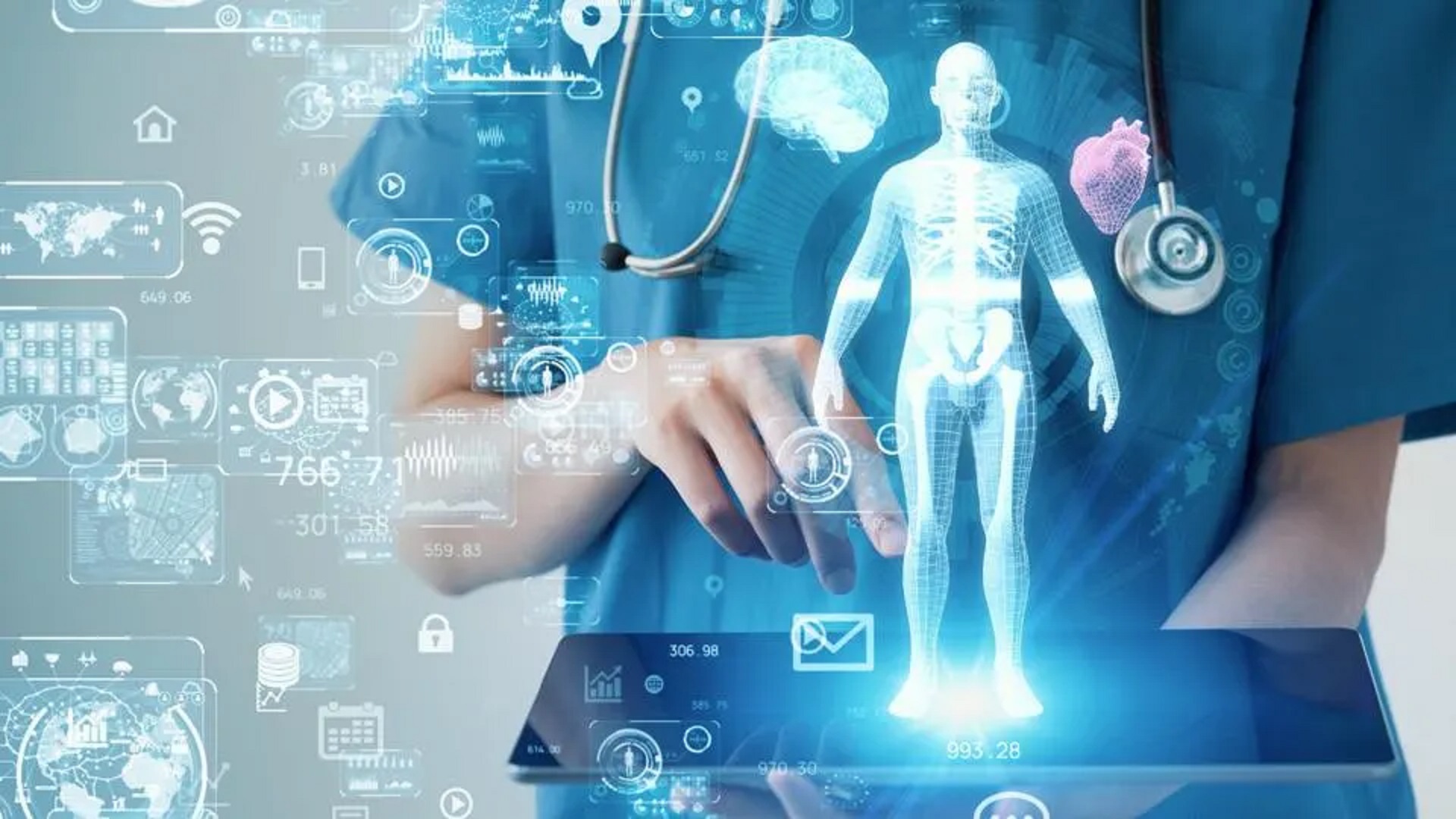
Experts emphasize the medical community’s role in shaping large language model (LLM) use in healthcare. In a JAMA article, researchers argue for active involvement to influence LLM development and training for medical tasks. They caution against using non-medical LLMs without medical record training and validation. By directing LLM creation, healthcare stakeholders can assess training data, benefits, and risks, ensuring accurate medical insights. Researchers also propose shared instruction tuning datasets and open-source LLMs to improve healthcare applications.
As the interest in utilizing large language models (LLMs) grows, experts argue that the medical field should take an active role in guiding their application within healthcare.
In a recent article published in the Journal of the American Medical Association (JAMA), researchers discussed the development and integration of large language models in healthcare. They emphasized that the medical community should play a proactive role in shaping this process.
While some stakeholders in healthcare are currently using pre-existing LLMs developed by technology companies to explore their potential impact on medical practices, the researchers propose a different approach. They suggest focusing on how the intended medical applications of LLMs and chatbots can influence their development and training.
The authors noted that certain LLM-based applications are being introduced to healthcare without proper training on medical records and without validating their potential benefits. To counter this trend, the researchers recommend that the medical community actively guide the creation and deployment of healthcare-focused LLMs. This guidance would involve providing relevant training data, defining desired benefits, and assessing the advantages through real-world testing.
To achieve this, healthcare stakeholders should consider two critical questions: Are LLMs being trained with suitable data and appropriate self-supervision methods? Are the proposed benefits of using LLMs in healthcare being substantiated?
The researchers explained that LLMs function by learning the probabilities of word sequences within a body of text, akin to predictive text tools. These probabilities are learned from enormous text datasets, resulting in models with billions of potential parameters. This process enables LLMs to perform tasks like summarizing text, answering questions, and more, even without explicit training for these tasks.
While general-purpose LLMs are capable of many medically relevant tasks, the authors highlighted the fact that they are not trained on medical records during their self-supervised training phase, and only a few are specifically tuned for medical tasks.
The authors argued that technology companies should not dictate the role of LLMs in medicine without involving the medical community in shaping their use. They cautioned against repeating the past mistake of letting external stakeholders dominate the creation, design, and adoption of health information technology systems.
Given the potential of advanced technologies to enhance healthcare, the authors emphasize the need to avoid making the same mistake with LLMs. By questioning the training data and self-supervision methods of LLMs, the medical community can contribute to their development. The authors recommend discussions among healthcare stakeholders to establish shared instruction-tuning datasets and examples of LLM prompts.
The researchers also propose that health systems create and train open-source LLM models using their data. Additionally, technology companies should provide clarity about whether their LLMs were trained on medical data and whether their training approach aligns with healthcare use cases.
To assess the potential benefits of LLMs in medicine, the authors suggest that medical stakeholders play a role in quantifying the benefits of each model’s use. They note that current assessments of LLMs do not adequately capture the potential advantages of collaboration between humans and models, which is crucial in healthcare settings.
The authors raise concerns about the contamination of training datasets and the reliance on standardized human-based tests to evaluate LLMs. They use the analogy of a driver’s license test to illustrate that just passing a medical licensing exam doesn’t necessarily qualify an LLM to provide medical advice.
Overall, the authors stress that it’s essential to define the benefits of technologies like LLMs and conduct proper evaluations to verify these benefits. Striking a balance between creating healthcare-focused LLMs and validating their assumed advantages is crucial to effectively augment clinicians’ judgment.
These warnings about the potential challenges of healthcare LLMs come at a time when such models are already being employed for various purposes within health systems. For instance, researchers at New York University’s Grossman School of Medicine developed an LLM called NYUTron, capable of predicting clinical outcomes by analyzing electronic health records. This tool demonstrated a five percent improvement in predicting readmissions compared to standard models and has been adopted by NYU Langone Health.