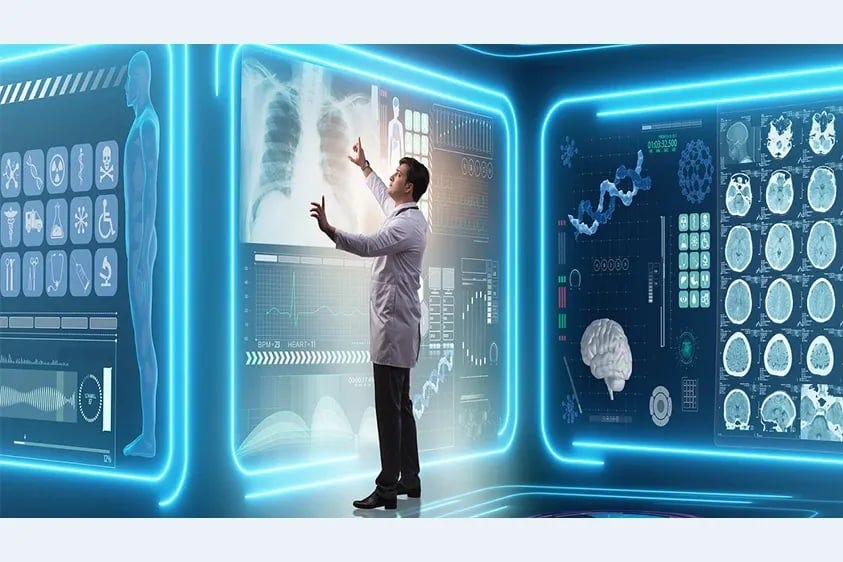
Johns Hopkins Medicine researchers have created a machine learning tool to estimate the percentage of necrotic tissue (PN) in intramedullary osteosarcoma patients. Accurate PN calculation is vital post-chemotherapy for prognosis. Traditional methods are labor-intensive and vary between pathologists. The ML model, requiring minimal annotation, showed a positive correlation of 85% with pathologist calculations, with a 99% correlation after removing an outlier. This tool has the potential to expedite prognosis and reduce the workload of musculoskeletal pathologists.
A team of researchers at Johns Hopkins Medicine has unveiled a machine learning (ML) tool designed to estimate the percentage of necrotic tissue (PN) in patients suffering from intramedullary osteosarcoma, a form of bone cancer originating in the bone’s central region.
Post-chemotherapy calculation of PN is crucial for providing patients with a reliable prognosis for their survival. PN quantifies the extent of tumor tissue that has become necrotic or inactive, serving as an indicator of the efficacy of chemotherapy treatment.
Their findings, published recently in the Journal of Orthopaedic Research, demonstrate that a PN of 99% implies that 99% of the tumor is no longer viable, indicating a successful treatment and improved chances of survival.
Determining PN traditionally involves pathologists interpreting and annotating whole-slide images (WSIs), which are thin sections of tissue specimens mounted on slides and observed under a microscope. In the context of intramedullary osteosarcoma, these specimens are bone tissue samples that necessitate assessment by a musculoskeletal pathologist.
Christa LiBrizzi, MD, co-first author of the study and a resident at Johns Hopkins Medicine’s Department of Orthopaedic Surgery, explained that PN calculation is labor-intensive, requiring extensive annotation data from musculoskeletal pathologists. Moreover, it suffers from low interobserver reliability, with different pathologists often reporting varying conclusions when calculating PN from the same WSIs. Due to these challenges, the researchers embarked on an effort to explore alternative methods for PN calculation.
The team set out to develop a “weakly supervised” ML model, which would require only a small amount of annotation data for training. This approach would significantly reduce the workload of musculoskeletal pathologists who could provide partially annotated WSIs to the tool for PN calculations.
To build the model, the researchers collected WSIs and other patient data from individuals with intramedullary osteosarcoma who had undergone surgery and chemotherapy at Johns Hopkins’ US tertiary cancer center between 2011 and 2021.
An expert musculoskeletal pathologist partially annotated three tissue types on each WSI: active tumor, necrotic tumor, and non-tumor tissue. The pathologist also calculated PN for each patient in the cohort, and this information was used to train the ML tool.
Zhenzhen Wang, co-first author of the study and a doctoral student in biomedical engineering at the Johns Hopkins University School of Medicine, explained that the model was trained to recognize image patterns. The WSIs were divided into small patches and grouped based on the pathologist’s labels, providing a robust frame of reference for the model.
Following training, the tool and musculoskeletal pathologist both analyzed six WSIs from two osteosarcoma patients. The researchers discovered an 85% positive correlation between the PN calculations and tissue labeling by both the pathologist and the ML model. The tool had some difficulty labeling cartilage in the slides, creating an outlier in one of the WSIs, but after its removal, the positive correlation increased to 99%.
Dr. LiBrizzi noted that if the model is validated and implemented, it could expedite the assessment of chemotherapy’s effectiveness and provide patients with prognosis estimates sooner, reducing healthcare costs and alleviating the workload on musculoskeletal pathologists.
In the future, the research team plans to incorporate cartilage tissue in the model’s training dataset and include a wider range of WSIs to encompass different types of osteosarcoma beyond intramedullary cases.
Leave a Reply