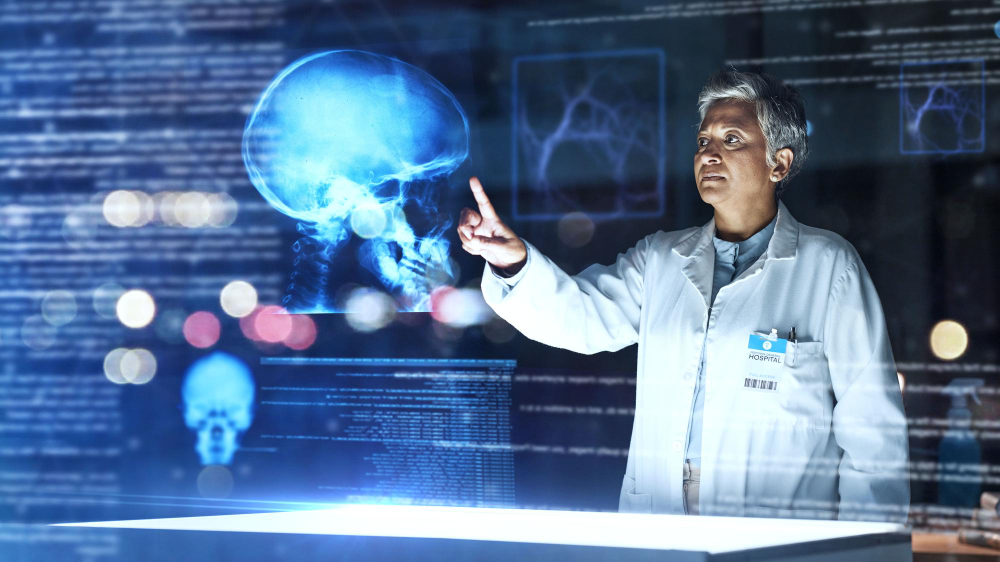
Researchers from the University of Texas at San Antonio, UT Health San Antonio, and the University of Pittsburgh have developed a generative AI tool to improve adaptive radiotherapy. By extracting high-quality textural and spatial features from pre-treatment CT scans and tumor shrinkage information from weekly CBCTs, the AI visualizes the tumor region affected by a patient’s radiotherapy, allowing for more accurate tumor evaluation. The study demonstrated improved tumor shrinkage predictions and a 35% decrease in the risk of radiation-induced pneumonitis. However, more research is needed before an AI-assisted approach can be implemented in clinical settings.
The University of Texas at San Antonio (UTSA), UT Health San Antonio, and the University of Pittsburgh have jointly developed a generative artificial intelligence (AI) tool to improve adaptive radiotherapy. Adaptive radiotherapy is a cutting-edge cancer therapy technique where treatment doses are continually adjusted based on changes in patient anatomy, such as weight loss or tumor shrinkage, or at predetermined intervals to provide the most precise dose of radiation possible. By doing so, clinicians have the potential to target tumors more effectively, reduce the amount of healthy tissue exposed to radiation, and minimize some side effects of treatment.
However, the location of a tumor in the body is normally determined using a computed tomography (CT) scan. A treatment strategy involving specialized radiation doses is then developed using this CT imaging and the location of the tumor. Cone-beam computed tomography (CBCT) is frequently utilized throughout the treatment plan, typically weekly or after each dose, to assess how much a tumor has shrunk and to alter dosage levels accordingly.
CBCTs, on the other hand, are low-quality images that can be difficult to read and interpret, leading to potential errors in treatment. To address this issue, the researchers turned to AI. The study was published in the May 2023 edition of Medical Image Analysis.
The researchers used domain adaptation techniques to extract high-quality textural and spatial features from pre-treatment CT scans and tumor shrinkage information from weekly CBCTs. The AI then aids in seeing the tumor area that a patient’s radiotherapy has affected, enabling a more precise tumor assessment.
Paul Rad, Ph.D., UTSA associate professor of information systems and cyber security, stated that the study was a multidisciplinary research project that involved multiple faculty members with different skill sets in AI, data analytics, and health care to address a challenge. “Our study aimed to analyze treatment doses administered and develop a precise map of a patient’s cancer progression while accounting for potential variability using uncertainty estimation,” he explained.
The study cohort included 16 cancer patients whose pre-treatment CT and mid-treatment weekly CBCTs were captured over six weeks. The researchers demonstrated that using the AI tool resulted in improved tumor shrinkage predictions and a 35% decrease in the risk of radiation-induced pneumonitis, a type of lung injury that can occur following radiotherapy.
With the use of this method, clinicians may be able to more accurately assess the amount of a tumor that shrinks each week and determine dosages for the ensuing weeks. The researchers stated that this increased level of accuracy has the potential to considerably enhance tumor targeting and enhance the personalization of ART planning. Before an AI-assisted ART strategy can be used in clinical settings, more research is required.
Arkajyoti Roy, Ph.D., UTSA assistant professor of management science and statistics, stated that the researchers were interested in exploring the limitations of the AI models in addition to developing more advanced models for radiotherapy. He believes that all models make errors, and for something as critical as cancer treatment, it is important not only to understand the errors but also to figure out how to limit their impact.