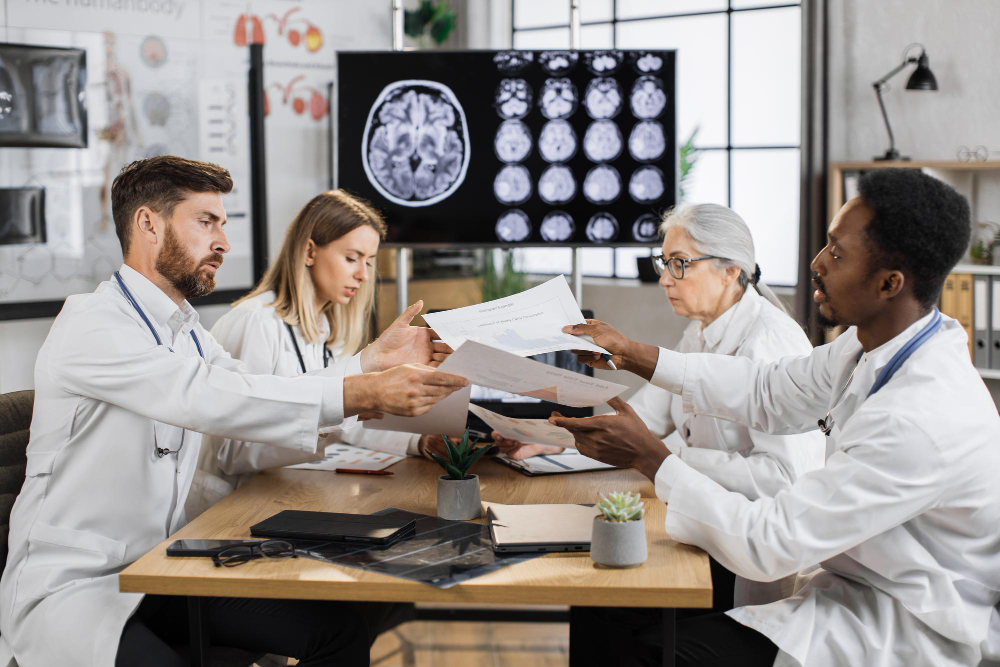
The Radiological Society of North America (RSNA) organized the RSNA Screening Mammography Breast Cancer Detection AI Challenge to foster the development of AI models that accurately detect breast cancer. With participation from nearly 1,700 teams, the challenge witnessed intense competition. The winning algorithms underwent evaluation by volunteer AI experts, and among the successful teams were Mr. Robot and Cancer Detection Man, who shared a prize pool of $50,000. This challenge directly addressed concerns regarding the performance of AI in diverse patient populations and leveraged a comprehensive dataset sourced from mammography screening programs. The availability of this diverse dataset will contribute to future research and the evaluation of AI tools in clinical settings, ultimately pushing forward the progress of mammographic diagnosis.
The Radiological Society of North America (RSNA) has recently disclosed the outcomes of the RSNA Screening Mammography Breast Cancer Detection AI Challenge, a research competition aimed at developing artificial intelligence (AI) models capable of accurately detecting breast cancer from mammography images. The challenge, which is part of a series of similar events conducted by RSNA since 2015, seeks to enhance the diagnostic process for radiologists by providing AI tools that can facilitate more precise and timely diagnoses.
Breast cancer stands as the most prevalent form of cancer worldwide, according to the World Health Organization, with 2.3 million new diagnoses and 685,000 deaths recorded in 2020 alone. The implementation of breast cancer screening has demonstrated its effectiveness in reducing cancer-related fatalities. With the potential to enhance the efficiency and effectiveness of screening, AI tools offer promising opportunities in the field.
Dr. Linda Moy, a professor of radiology at the NYU Grossman School of Medicine and editor of the journal Radiology, highlighted the concerns among radiologists regarding the performance of AI systems in diverse patient populations. The RSNA Mammography AI Challenge aimed to address these concerns by employing a well-curated and diverse dataset that can be used to evaluate the generalizability of AI systems across different patient demographics. Dr. Moy emphasized the collaborative nature of the challenge, emphasizing its potential to improve diagnostic accuracy and ultimately save lives.
The challenge was hosted on the Kaggle platform, provided by Kaggle, Inc., an Alphabet company. It attracted nearly 1,700 teams from around the world and commenced on November 28, 2022, running through February 2023. Following the conclusion of the challenge, the top-scoring algorithms were reviewed by a team of volunteer AI experts to confirm the results. The eight teams with the highest scores shared a total prize pool of $50,000.
The winning teams in the RSNA Screening Mammography Breast Cancer Detection AI Challenge were: Mr. Robot, Cancer Detection Man, H.B.M.F., CDI, Racers, Chiral Mistrals, Luddite & MT, and BCC. Additionally, the review committee awarded the Educational Merit Award to the Mr. Robot team in recognition of the clarity of their solution and the accompanying presentation materials.
The dataset utilized in the challenge was contributed by mammography screening programs in Australia and the United States. It encompasses detailed labels featuring evaluations by radiologists and follow-up pathology results for suspected malignancies. The dataset will remain available for further research purposes.
This challenge is part of a broader research initiative that aims to evaluate the performance of the competition-generated models against previously unseen data and compare their effectiveness to that of expert human observers. These evaluations are crucial in determining the feasibility and performance of AI tools in clinical settings.
Dr. John Mongan, a professor of radiology at the University of California, San Francisco, and chair of the RSNA Machine Learning Steering Committee, expressed enthusiasm about the significant participation in the competition, reflecting the substantial interest in leveraging large, high-quality datasets to advance mammographic diagnosis. Dr. Mongan expects that the dataset and the work of the participants will provide a solid foundation for rapid advancements in breast imaging AI.