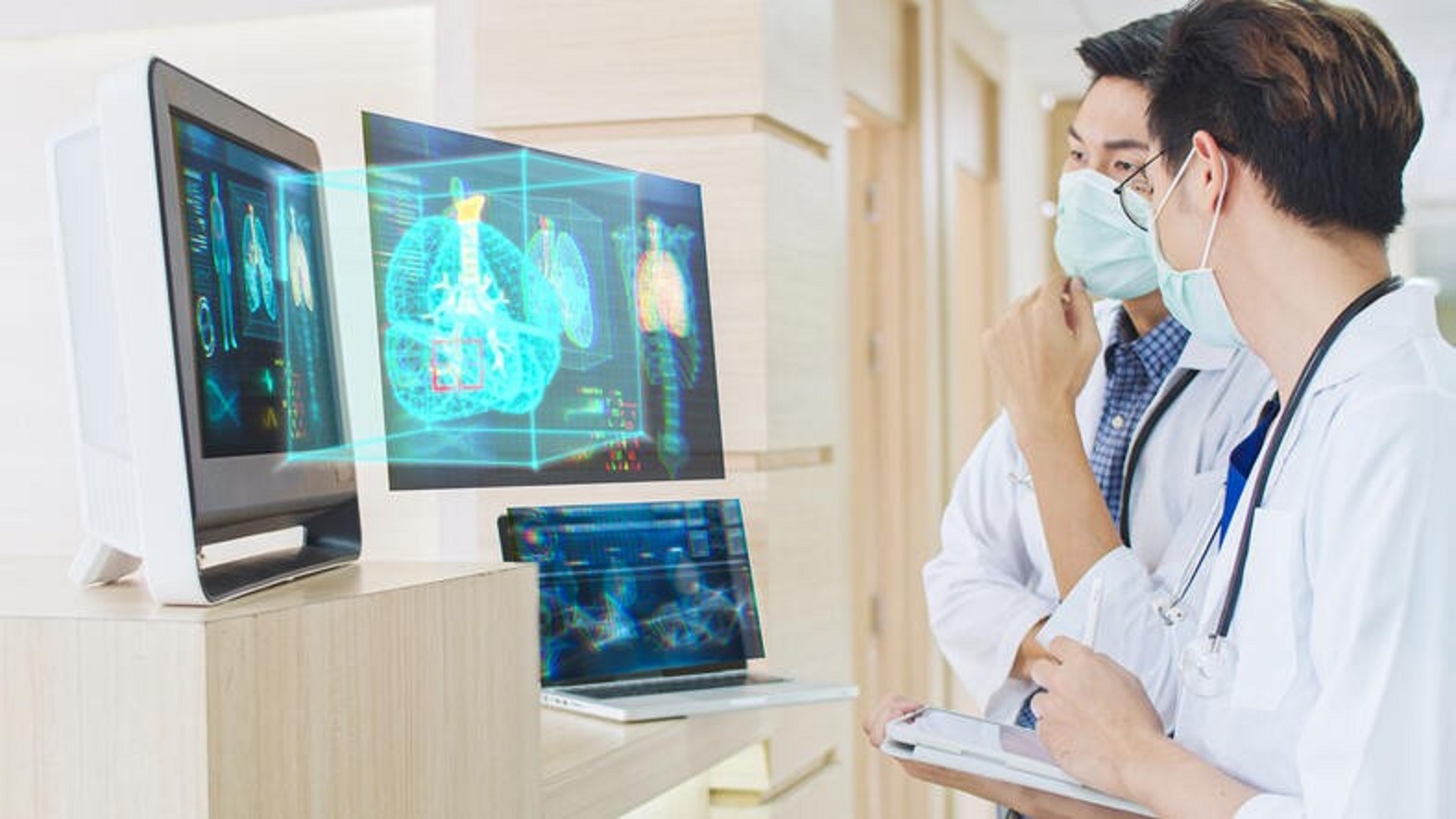
Artificial intelligence (AI) and machine learning are being leveraged in healthcare organizations to effectively manage and protect vast amounts of patient data. These technologies help categorize and prioritize data, apply appropriate security measures, and prevent cyber threats. Michael K. Giannopoulos of Dell Technologies emphasizes the importance of developing AI models with input from clinical stakeholders. Radiology is leading the adoption of AI-assisted scans, and digital pathology is expected to follow suit. The key advice is to prioritize patient well-being while harnessing the potential of AI and advanced analytics.
Artificial intelligence (AI) and machine learning are being leveraged in healthcare organizations to effectively manage and protect vast amounts of patient data. In the realm of healthcare organizations, the accumulation of overwhelming amounts of data, often referred to as “data sludge,” has become a pressing challenge.The continuous generation of healthcare and life sciences data from various sources, including medical practices, laboratories, pharmaceutical companies, payers, electronic health records, imaging systems, and Internet of Things (IoT) devices, has contributed to this predicament.
Michael K. Giannopoulos, the Chief Information Security Officer and Chief Technology Officer for Dell Technologies’ Healthcare and Life Sciences division, warns about the repercussions of failing to manage this data effectively.
Giannopoulos emphasizes that the sheer volume of data and the multitude of sources have reached a point where human management is becoming unfeasible. Consequently, he describes the resulting data as “amorphous blobs” that necessitate meticulous organization based on relevance.
In this context, the integration of artificial intelligence and machine learning technologies offers significant potential for healthcare organizations to regain control over their data. These advanced tools not only synthesize data sets to derive actionable insights but also enable rapid parsing, prioritization, and secure protection of patient data.
Giannopoulos clarifies that the objective is not to replace human input but rather to enhance human capacity and improve care delivery. To achieve this, it is crucial to develop AI models with input from clinical stakeholders, in addition to data scientists.
AI can assist healthcare IT teams in categorizing data and applying cost-effective security guidelines based on data classifications. This approach ensures that different types of data receive appropriate protection based on their relevance. For instance, electronic medical records and imaging data, falling under Tier 1, would require a more robust cybersecurity infrastructure compared to Tier 2-level user access data logs.
Importantly, Giannopoulos emphasizes that this tiered approach does not compromise the security of any data. Rather, it rationalizes the impact on operations and recovery time objectives (RTO), potentially leading to cost savings.
One notable AI application gaining attention is generative AI, exemplified by OpenAI’s ChatGPT, which has the potential to distill vast amounts of data into manageable fragments. Giannopoulos envisions that these large language models can eventually assist healthcare organizations in determining appropriate data protection levels and preventing cyber threats such as ransomware and data exfiltration.
Giannopoulos envisions the development of private generative AI systems within health systems, utilizing internal data to automate and implement suitable security guidelines. He notes that currently available generative AI products, such as ChatGPT, which rely on the broader internet, may produce inaccurate responses or fabricate patient studies and results.
Giannopoulos believes that radiology, with its focus on detecting anomalies in images, is at the forefront of AI-assisted scans. Digital pathology, being image-based as well, is expected to follow suit in embracing AI, as it is conducive to building rule sets for identifying abnormalities.
Finally, Giannopoulos offers a key piece of advice to health systems interested in leveraging AI and advanced analytics to protect and validate patient data while reducing “data sludge”: “Know your data and remember… Your North Star is always the patient.”