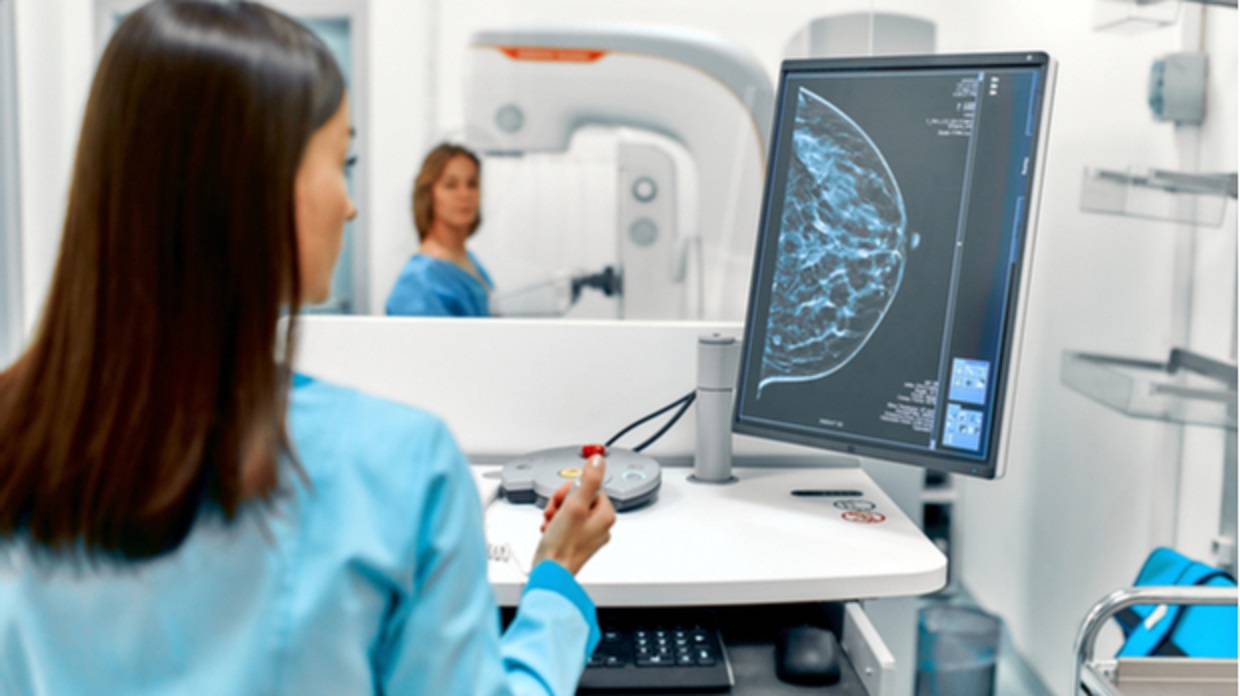
According to a recent US study, combining AI-powered image analysis with machine learning enhances forecasts for invasive breast cancer. According to a study from the Mayo Clinic and the University of California, combining AI with image-based risk scores can effectively estimate a person’s future risk of developing breast cancer. The AI program minimized variability in estimating breast density by precisely characterizing breast tissue. The study focused on the possibility of AI-driven diagnostic tools to improve individualized screening and prevention efforts for at-risk women.
AI has shown promising results in improving predictions for invasive breast cancer, according to a recent study conducted in the United States. The study explored the combination of machine learning and AI-powered image analysis to enhance mammography screening, streamline radiology workflows, and enable earlier detection for women at high risk.
Researchers from the Mayo Clinic and the University of California, San Francisco, conducted the study, which revealed that the integration of AI-powered image analysis with image-based risk scoring can effectively predict long-term breast cancer risk. The study, published in the Journal of Clinical Oncology, utilized Screenprint Medical’s Transpara Exam Score in conjunction with Volpara’s TruDensity software.
The researchers analyzed two-dimensional full-field digital mammograms performed 2–5.5 years before the diagnosis of invasive breast cancer in 2,412 women, comparing them with a control group of 4,995 women from two US mammography cohorts. They found that employing AI-powered image analysis with an image-based risk tool allowed radiologists to accurately quantify breast tissue and reduce variability in assessing breast density.
The TruDensity AI algorithm, incorporating X-ray physics and machine learning, generated precise volumetric measurements of breast composition, eliminating the inconsistencies that may arise from human interpretation. Dr. Ralph Highnam, Volpara Health’s chief science and innovation officer, emphasized the importance of an objective, volumetric measurement of breast density in assessing breast cancer risk.
The retrospective study corroborated the findings of a previous study conducted in Norway, which integrated Transpara’s exam scores and TruDensity’s image analysis. The Norwegian study evaluated screening outcomes from 2010 to 2018, including 949 screen-detected breast cancers, 305 interval cancers, and 13,646 negative examinations. The researchers observed that the AI scores improved the prediction of all cancer types, particularly advanced cancer. However, statistical significance was not achieved for interval cancer or breast cancer detected between mammography screenings.
The introduction of AI-driven diagnostic tools is driven by the need to address the classification of missed cancers and the shortage of European radiologists. The accuracy of AI systems has been compared to double reading protocols by Norwegian researchers, highlighting the potential of AI to support or replace radiologists in the reading process.
While the application of AI in breast cancer detection offers significant advantages and efficiencies, it is essential to remember that the final diagnosis and medical decisions lie with physicians. AI cannot independently provide a definitive diagnosis or replace the expertise of medical professionals.
Dr. Nico Karssemeijer, chief scientific officer at Screenpoint Medical and professor at Radboud University, emphasized the advancements made in understanding the combined effects of breast density and image-based risk in driving personalized medicine for women. The integration of AI technology holds promise for improving breast cancer detection, advancing early intervention, and reducing breast cancer mortality rates worldwide.