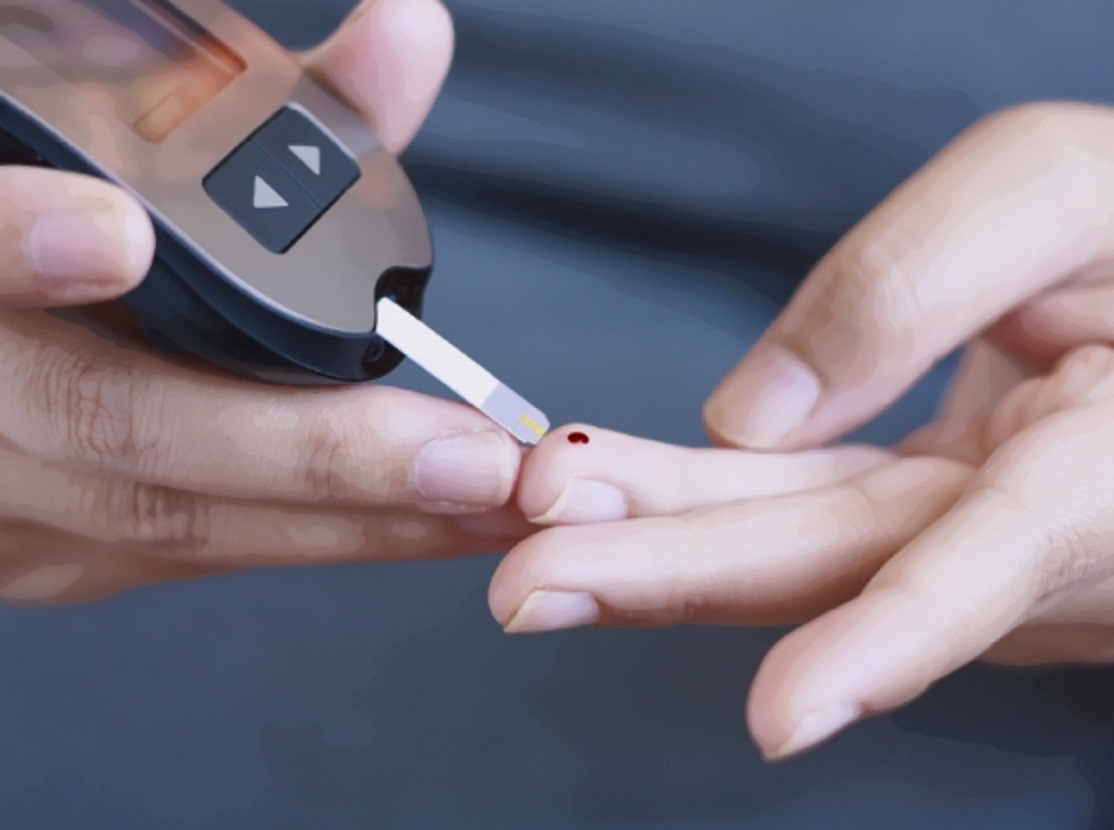
The ADA Scientific Sessions showcased advancements in diabetes care, including an AI tool for predicting diabetic retinopathy progression and a glucose sensing and delivery device for Type 1 diabetes. The AI tool improves risk estimation using retinal images, potentially reducing costs and improving outcomes. The novel device combines glucose sensing and insulin delivery, simplifying treatment and reducing the burden for patients. These studies contribute to the ongoing exploration of advanced technologies in diabetes management.
The recent ADA Scientific Sessions showcased the latest advancements in diabetes care, focusing on innovative devices such as AI-based retinopathy risk prediction and a novel glucose sensing and delivery system.
At the 83rd Scientific Sessions organized by the American Diabetes Association (ADA) in San Diego, California, researchers presented studies that highlighted significant developments in diabetes care technology. These included an artificial intelligence (AI) tool capable of predicting the progression of diabetic retinopathy and a groundbreaking glucose sensing and delivery device for individuals with Type 1 diabetes.
These presentations are particularly timely as AI and other advanced digital health technologies are gaining momentum in the healthcare industry, particularly in the realm of chronic disease management.
The first study, titled ‘Identifying the Risk of Diabetic Retinopathy Progression Using Machine Learning on Ultrawide Field Retinal Images,’ explored the potential of AI in estimating the risk of diabetic retinopathy progression. Diabetic retinopathy affects approximately one in three of the 37 million Americans with diabetes, making it the leading cause of blindness among working-age adults in the United States.
Early detection and treatment are vital in preventing the progression of this eye disease. However, accurately assessing the risk of progression can be challenging for healthcare providers. The study demonstrated that machine learning algorithms, when applied to ultrawide field (UWF) retinal images labeled for baseline diabetic retinopathy severity and progression, significantly improved the estimation of disease progression. The AI predictions for 91 percent of the images either matched the correct labels or indicated a greater estimated progression compared to the original labels. These findings suggest that machine learning algorithms can refine risk assessment, personalize screening intervals, and potentially improve vision-related outcomes while reducing costs.
The second study, ‘Feasibility of a Prototype Dual Function Glucose Sensing Insulin Delivery Cannula in People with Type 1 Diabetes,’ evaluated the viability of an automated insulin delivery (AID) system known as an artificial pancreas. These systems employ continuous glucose level sensors and insulin pumps to deliver insulin, primarily for individuals requiring intensive insulin therapy.
However, existing AID systems often involve multiple devices and separate insertions for the sensor and insulin-delivery cannula. This setup adds complexity for patients and can lead to frustrations resulting from unsynchronized device replacement timelines. To address these challenges, researchers examined the feasibility of a single through-the-skin device, named SynerG, that combines glucose sensing and insulin delivery functions.
The device was tested on 24 patients with Type 1 diabetes who were already using insulin pump therapy (IPT). It was subcutaneously inserted into the abdomen and connected to the pump. The device underwent calibration during its warm-up period, and participants consumed a standardized meal one hour after insertion. Blood glucose levels were measured every ten minutes before the meal and every 15 minutes for four hours afterward.
The results demonstrated reliable insulin delivery and no interference between the insulin and glucose sensor. This promising development represents a potential advancement in managing glucose levels for individuals with diabetes. The single-insertion subcutaneous device that combines glucose sensing and insulin infusion is expected to reduce the burden of care and alleviate psychological distress for those who require insulin.
These studies contribute to the ongoing evaluation of advanced technologies in diabetes care and management. In a previous year, researchers at the University of Houston developed an AI-based clinical decision support tool called Primary Care Forecast, which predicts the risk of diabetes complications by utilizing deep learning and analyzing a patient’s health history. By proactively identifying high-risk individuals, the tool enhances the Diabetes Complication Severity Index (DCSI) Progression Tool, which assesses diabetes complication risk by considering patient health history, social determinants of health, and environmental factors. The aim is to empower clinicians to take proactive measures in treating diabetes and effectively connect patients with suitable interventions.