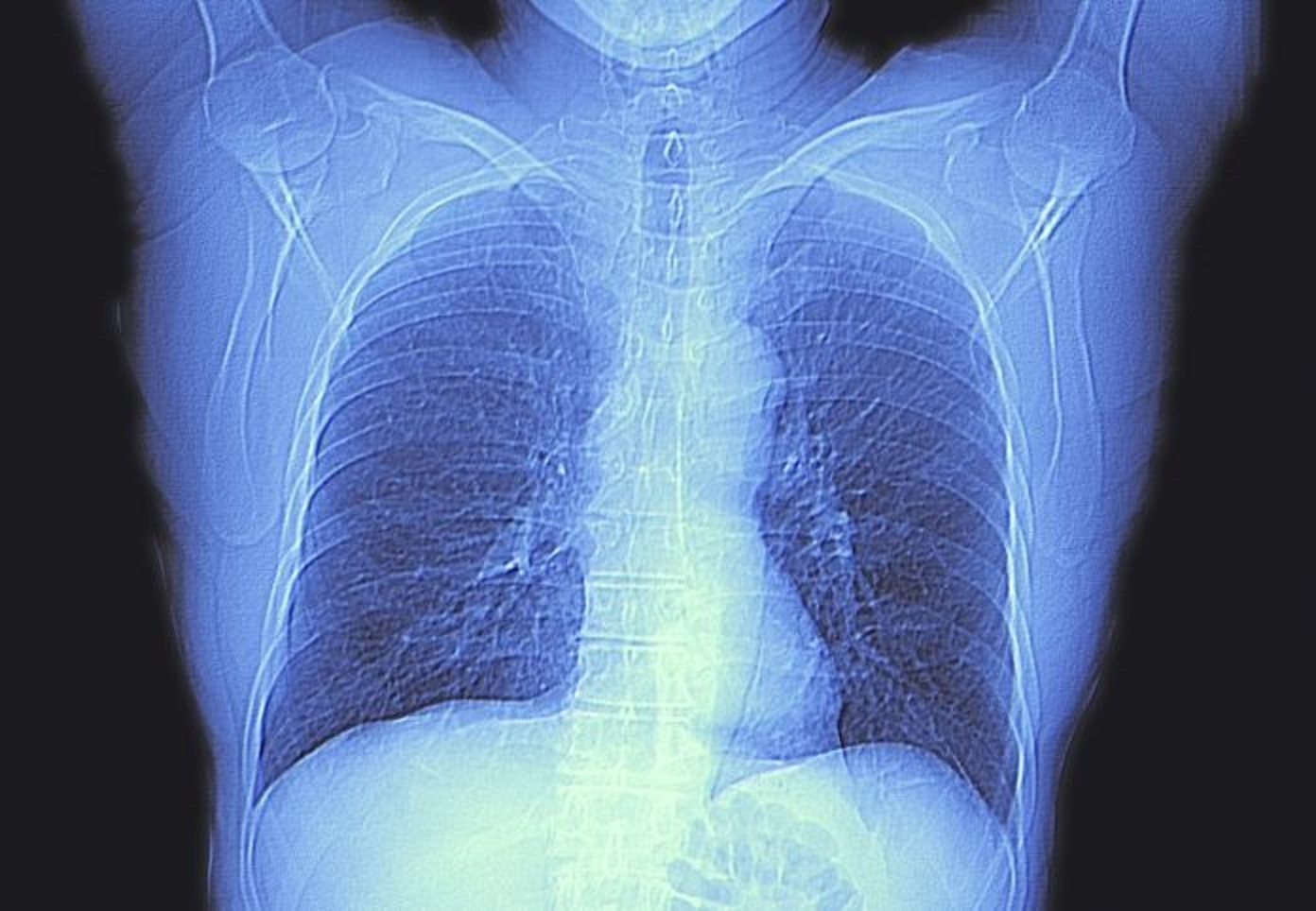
AI can leverage low-dose CT scan data of the lungs to enhance risk prediction for mortality from lung cancer and cardiovascular disease, as per a study in Radiology. The AI algorithm automatically derives body composition measurements, offering insights into chronic health conditions. This opportunistic screening could aid in early interventions for high-risk individuals, going beyond lung cancer detection. The study analyzed more than 20,000 participants and showed improved mortality risk prediction, particularly with fat within muscles as a strong predictor. Further research aims to explore longitudinal correlations between body composition changes and health outcomes.
According to a study published in Radiology, a journal of the Radiological Society of North America (RSNA), artificial intelligence (AI) can leverage data from low-dose CT scans of the lungs to significantly improve risk prediction for mortality caused by lung cancer, cardiovascular disease, and other factors.
The U.S. Preventive Services Task Force advocates for annual lung screening using low-dose CT (LDCT) of the chest for individuals between the ages of 50 and 80 years who are at high risk of developing lung cancer, especially longtime smokers. Apart from providing lung images, these scans also offer valuable information about other chest structures.
Kaiwen Xu, a Ph.D. candidate in the Department of Computer Science at Vanderbilt University in Nashville, Tenn, and lead author of the study explained that while CT images are primarily used to identify lung cancer nodules, they also contain significant anatomical data, including body composition information.
Xu and the research team had previously developed and publicly released an AI algorithm capable of automatically extracting body composition measurements from lung screening LDCT. Body composition, which encompasses fat, muscle, and bone percentages in the body, plays a crucial role in determining various health conditions, such as metabolic disorders. Studies have shown its relevance in risk stratification and prognosis for cardiovascular disease and chronic obstructive pulmonary disease, as well as its impact on survival and quality of life in lung cancer therapy.
In their latest study, the researchers evaluated the additional value of AI-derived body composition measurements. They analyzed CT scans from over 20,000 individuals who participated in the National Lung Screening Trial. The results demonstrated that integrating these measurements into the analysis improved the prediction of mortality risk from lung cancer, cardiovascular disease, and all-cause mortality.
Xu pointed out that the introduction of automatic AI body composition analysis extends the utility of lung screening with low-dose CT beyond merely detecting lung cancer. It enables the identification of high-risk individuals who may benefit from interventions like physical conditioning or lifestyle modifications even before the onset of any disease.
Notably, measurements related to fat within muscles showed particular promise as strong predictors of mortality, a discovery consistent with existing research. Myosteatosis, which refers to the infiltration of skeletal muscle with fat, is now believed to be a more indicative factor for health outcomes than a mere reduction in muscle bulk.
The integration of body composition measurements into lung screening LDCT exemplifies opportunistic screening, where imaging conducted for one purpose offers insights into other health conditions. This practice is expected to have significant potential for routine clinical use.
Xu emphasized that the CT images, originally ordered for lung cancer early detection, contain a wealth of additional information. Within the chest CT space used for lung cancer screening, valuable data on body composition and coronary artery calcification, directly linked to cardiovascular disease risk, can also be obtained.
As for future research, the team intends to conduct longitudinal studies, following individuals over time to observe how changes in body composition correlate with health outcomes.