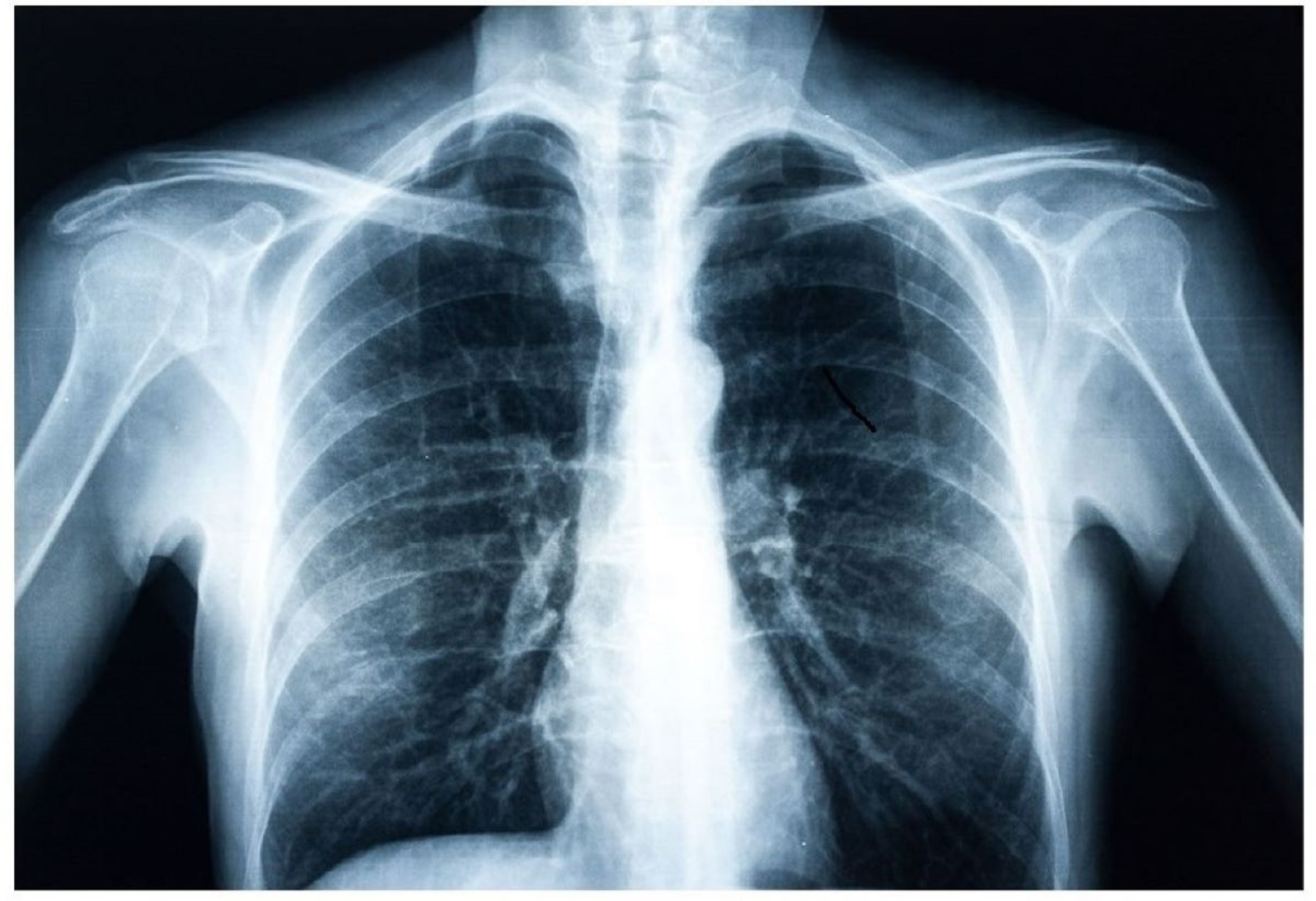
Emory University researchers have unveiled a groundbreaking deep-learning model that utilizes routine chest x-rays and electronic health records to predict early signs of diabetes, even in patients not meeting conventional risk criteria. The AI-driven tool’s ability to identify diabetes risk up to three years before diagnosis holds significant potential for early intervention and tailored treatment. The model’s reliance on chest X-rays as an “opportunistic” method aligns with recent research linking visible fatty tissue locations to diabetes risk. The model’s accuracy surpasses traditional clinical data, fostering optimism for enhanced diabetes care.
Scientists at Emory University have unveiled a pioneering deep learning (DL) model that can identify early indicators of diabetes using routinely collected chest X-rays and electronic health record (EHR) data. The model’s effectiveness is particularly notable in patients who do not fit the established criteria for elevated diabetes risk.
By harnessing the power of artificial intelligence, this tool holds the potential to revolutionize the detection of diabetes and consequently mitigate the associated complications. The research underscores the significance of detecting diabetes at its early stages, especially given the substantial increase in diabetes prevalence across the United States in recent decades.
In the year 2021, the United States Preventive Services Task Force (USPSTF) recommended that individuals aged 35 to 70 years, who are asymptomatic and have obesity or overweight conditions, should undergo screening for prediabetes and type 2 diabetes.
However, the researchers noted that this screening protocol might not effectively identify a significant number of individuals at risk, particularly those from diverse racial and ethnic backgrounds for whom the body mass index (BMI) is not a reliable predictor of diabetes risk.
Undiagnosed diabetes can precipitate a range of serious complications, including heart disease, kidney disease, vision impairment, and even mortality.
Interestingly, many individuals undergo chest X-rays annually for various reasons, and these X-ray images are stored as part of their electronic health records. The advent of AI in healthcare has sparked research into the potential impact of this technology on medical imaging. Studies have revealed that radiographs can hold clues to various diseases, including diabetes.
The stored X-ray images in electronic health records could be analyzed to glean insights into the presence of diabetes or related conditions.
Dr. Judy Wawira Gichoya, an assistant professor of radiology and imaging sciences at Emory, who played a leading role in the study, emphasized that chest X-rays offer an “opportunistic” alternative for diabetes detection. She noted that this application of AI to extract valuable information from tests performed for other purposes could significantly enhance patient care.
To develop their model, the researchers trained a deep learning algorithm to identify features that most accurately predicted future diabetes diagnoses. They generated a risk score based on 270,000 X-ray images from a retrospective cohort of 160,000 patients. Notably, the approach employed differed from standard methods for forecasting diabetes risk, prompting the research team to incorporate explainable artificial intelligence techniques to comprehend the model’s decision-making process.
The researchers uncovered that the location of visible fatty tissue in chest X-rays plays a crucial role in predicting diabetes risk. This finding aligns with recent research indicating that visceral fat in the upper body and abdomen is linked to conditions like hypertension, insulin resistance, and type 2 diabetes.
The Emory team validated the model using chest x-ray data from an additional 10,000 patients, revealing that the model outperformed a version using only non-image clinical data for predicting diabetes risk. Furthermore, the model demonstrated the capability to identify high diabetes risk up to three years before an official diagnosis.
The researchers propose that the risk scores generated by the model could assist healthcare providers in tailoring treatment approaches for their patients.
Dr. Francisco Pasquel, an associate professor in Emory’s Division of Endocrinology, Metabolism, and Lipids, highlighted the importance of body fat distribution in diabetes and its complications. He praised the innovative method of employing chest X-rays to identify individuals at high risk of diabetes, even before significant fluctuations in blood sugar levels manifest, as a promising avenue for early intervention and prevention.
In the future, the research team aims to further validate the model and integrate it into electronic health records, encouraging care teams to consider diabetes screening for patients outside the USPSTF guidelines.
Meanwhile, other researchers are also exploring AI’s potential in predicting chronic diseases. In 2020, a team from MIT’s Computer Science and Artificial Intelligence Laboratory (CSAIL) introduced a machine learning model capable of forecasting heart failure risk using a single X-ray.
This tool gauges the severity of excess fluid in patients’ lungs, which often serves as an early indicator of impending heart failure and guides treatment decisions. Given the complexity of quantifying fluid levels, the model employs subtle features in X-rays for accurate diagnoses, achieving a 90 percent success rate in identifying severe cases.