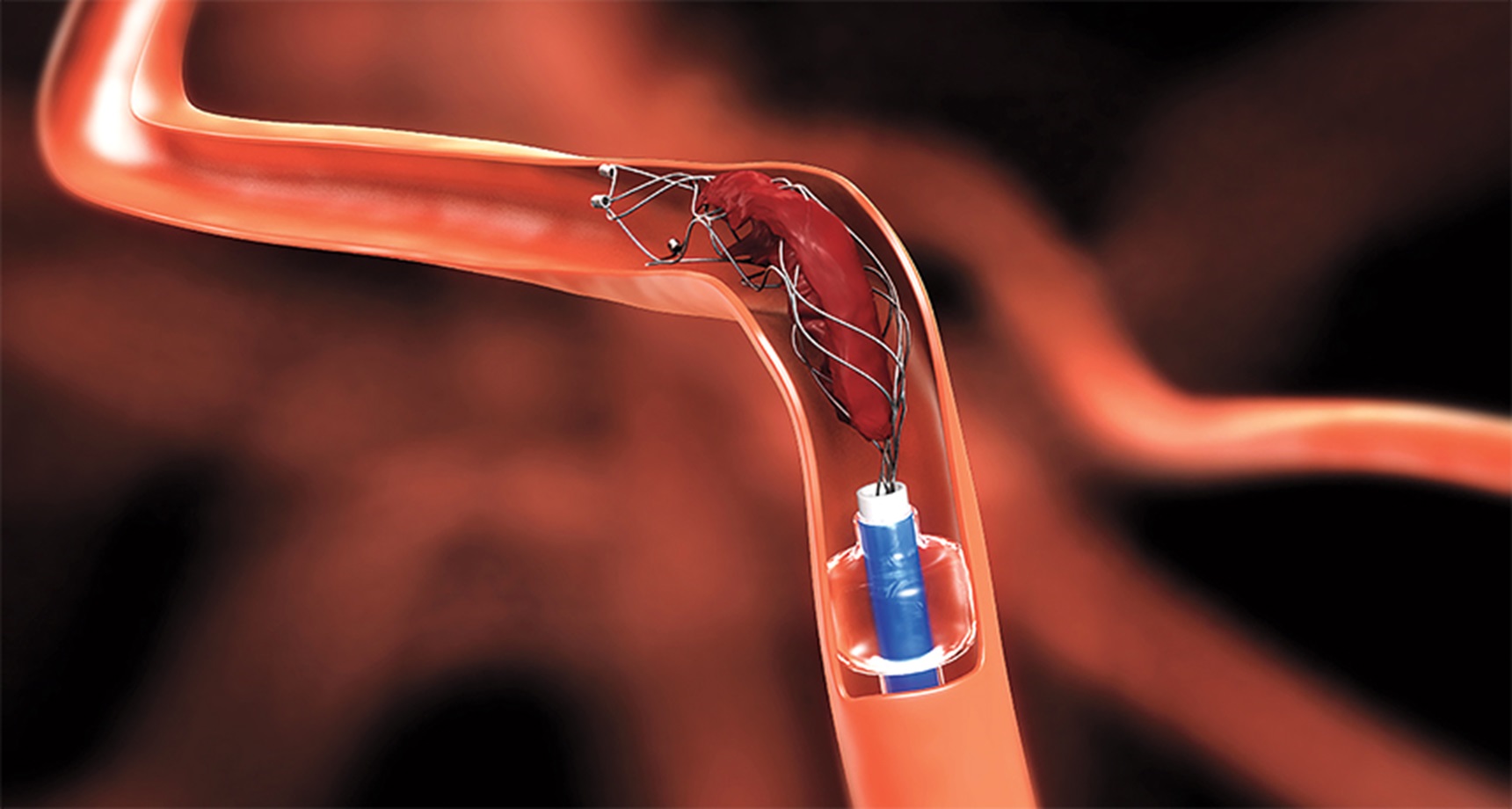
Vanderbilt University Medical Center scientists developed an AI tool to assess blood clot risks in pediatric patients, yet clinicians hesitated to adopt its recommendations. Analyzing 110,000 patient records, researchers identified 11 clot risk factors, creating a predictive model tested in a 15-month study involving 17,000 patients. Study group patients receiving AI-recommended anti-clot therapy showed no bleeding complications, similar clot rates to controls, but clinicians followed the tool’s advice only 26% of the time. Researchers stressed the necessity of pragmatic trials to ensure safe AI implementation and plan further studies to overcome clinicians’ reluctance and enhance AI’s healthcare impact.
Researchers at Vanderbilt University Medical Center (VUMC) have created an advanced artificial intelligence (AI) tool designed to accurately gauge the risk of blood clots in pediatric patients. However, despite its precision, clinicians frequently hesitated to comply with the tool’s suggestions.
Although blood clots are rare in pediatric cases, those affected often experience prolonged hospital stays and heightened risks of adverse outcomes. To address this, the researchers aimed to develop and validate an algorithm capable of identifying high-risk patients.
The process commenced with an analysis of electronic medical records (EMRs) from 110,000 patients at the Monroe Carell Jr. Children’s Hospital at Vanderbilt. From this data, the team pinpointed 11 factors associated with blood clot risks, such as prior surgeries, infectious diseases, cardiology consultations, and specific diagnoses.
Utilizing this information, the researchers created a predictive model that automatically assessed EMRs, calculating a risk score for each pediatric hospital admission daily. This approach enabled the team to focus more effectively on patients at the highest risk.
The AI tool’s risk predictions were tested over a 15-month period during the Children’s Likelihood of Thrombosis (CLOT) clinical trial, involving 17,000 patients. The patients were divided into a study group and a control group. While both groups received risk scores generated by the AI tool, only the study group’s scores were shared with their care teams.
For patients in the study group, risk scores were accompanied by recommendations to start anti-thrombolytic therapy, a treatment that prevents blood clot formation. Patients in the control group, identified as high risk by their clinicians, also received clot prevention therapy.
Interestingly, no bleeding complications were observed in patients receiving blood thinners as part of their anti-thrombolytic therapy. Moreover, there was no significant difference in blood clot rates between the two groups at the trial’s conclusion.
However, clinicians followed the AI tool’s recommendation to initiate anti-thrombolytic therapy in study group patients less than 26 percent of the time. Clinicians expressed concerns about potential major bleeds, even though this outcome was not observed in the study.
The researchers highlighted the importance of these findings for the future deployment of AI in healthcare. They emphasized the need for a system to assess AI models, ensuring safer and more effective patient care. The study underlined the significance of conducting pragmatic patient-level, randomized, controlled trials to determine the safety and efficacy of AI tools.
To delve deeper into clinicians’ reluctance to follow AI recommendations and identify solutions, the researchers plan to conduct another clinical trial. This effort aims to assess the value of predictive AI models in healthcare and enhance their implementation, ultimately benefiting patients who stand to gain the most from these innovations.