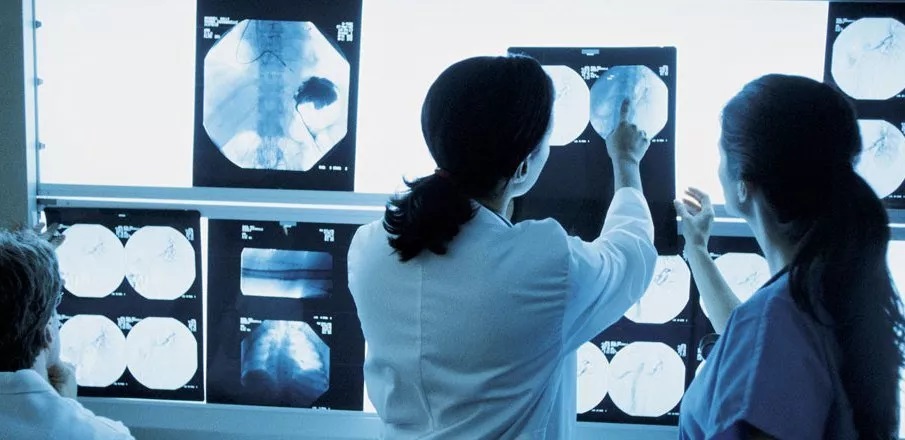
Exploring the intersection of radiology and artificial intelligence (AI), this summary encapsulates insights from RSNA 2022’s keynote on AI fundamentals for radiologists. It highlights Katherine P. Andriole and Linda Moy’s expertise, addressing AI’s role in diagnostics and workflow, while urging careful AI integration into practices. It emphasizes the importance of trust, bias mitigation, and value assessment in adopting AI tools, spotlighting the need for evidence-based adoption over industry hype.
The rapid incorporation of machine learning (ML) and artificial intelligence (AI) into the field of radiology has sparked significant interest and activity, prompting the editorial team at ITN to extend its report on this evolving technological landscape. Insights and progress from industry front-runners have been central to this coverage.
This article distills the essence of a multi-segment feature based on a keynote session from RSNA 2022 titled “Back to the Basics: What Do Rads Need to Know About Radiology AI.” This session offered a foundation in the application of ML and AI within radiology, featuring summaries from two distinguished experts, along with contributions from panelists Dania Daye, MD, Ph.D., and Walter Wiggins, MD, Ph.D., as covered previously in our July/August edition.
As RSNA 2023 approaches, shining a spotlight on new updates and breakthroughs, ITN presents a synopsis of pivotal discussions from that influential session. Highlighted here are insights from notable field authorities, Katherine P. Andriole, Ph.D., and Linda Moy, MD, illustrating their profound involvement and pioneering roles in this arena.
Katherine P. Andriole, Ph.D., awarded the RSNA 2022 Gold Medal, serves as an Associate Professor of Radiology at Harvard Medical School and holds leadership roles within the Mass General Brigham Data Science Office. Her extensive involvement with the RSNA Radiology Informatics Committee and as a faculty member for the RSNA Imaging AI Certificate Program underscores her significant contributions to the field.
Linda Moy, MD, a Professor of Radiology at the NYU Grossman School of Medicine, oversees breast MRI and co-leads an international AI team across the NYU Langone Health network. As the first female editor of the RSNA journal Radiology and Vice President of the Society for Breast Imaging (SBI), Moy’s experience is substantial.
Fundamentals of AI and ML for Radiology
Dr. Andriole’s presentation on the “Basic Principles of Artificial Intelligence/Machine Learning in Radiology” was an instructive journey, spanning from algorithm introductions to discussing AI’s current and future roles in radiology. She differentiated between AI, ML, and deep learning, with a special emphasis on how these technologies pertain to image analysis.
She elucidated on supervised machine learning, where models are trained using labeled data, and discussed convolutional neural networks (CNNs), pivotal in image-based ML. The focus was not only on understanding these technologies but also on the comprehensive steps required to build a model, which includes developing a relevant clinical question and the meticulous work involved in data preparation.
Evaluating AI’s Role in Radiology
Dr. Moy directed attention to the evaluative aspects of AI technology integration into radiology practices. Her talk provided a practical lens, posing critical questions regarding system integration, cost analysis, and overall impact. She urged the consideration of AI’s scope, problem-solving capabilities, coordination, return on investment, and ultimately its benefit to patients or the practice’s mission.
Underlining the importance of trust in AI systems, Moy emphasized the need for rigorous testing on diverse datasets to minimize bias and enhance generalizability. She highlighted the issue of automation bias, cautioning against over-reliance on AI systems and advocating for continued scrutiny even as these technologies are adopted.
Integrating AI: Efficiency and Infrastructure Considerations
Delving into the practicalities of AI adoption, Moy suggested a thorough interrogation of a system’s value before integration, advising practitioners to differentiate between essential tools and ‘nice-to-have’ options. She encouraged inquiries about IT infrastructure requirements, operational impacts, and system interoperability, advising collaboration with IT professionals early in the decision-making process.
The Value Proposition of AI
Moy concluded by stressing the importance of critically evaluating AI solutions beyond the hype, to understand their true potential and realistic benefits for radiological practices. She also noted the challenge in quantifying indirect benefits, such as improved radiologist satisfaction and reduced burnout, which are crucial yet harder to measure.
In sum, the overarching message from both experts was clear: While AI in radiology holds great promise, its successful implementation is contingent on thoughtful consideration, critical analysis, and collaborative effort across various disciplines within the healthcare system.