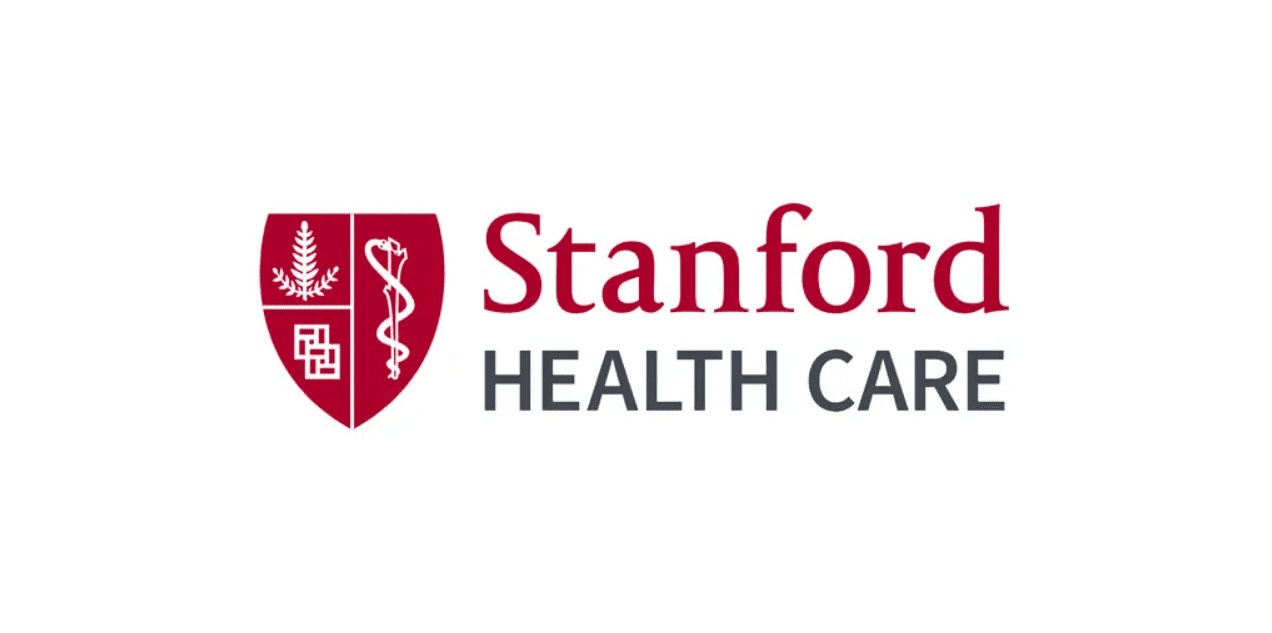
Stanford Health Care integrates AI and machine learning (ML) models to identify clinical deterioration in hospitalized patients. Their initiative aims to align care teams and streamline workflows, improving patient outcomes by predicting risks every 15 minutes. Dr. Shreya Shah, an expert in AI healthcare integration, highlights the challenges of detecting deterioration in complex patients. The AI-based system enhances communication among multidisciplinary teams, resulting in a 20% reduction in clinical deterioration events. Transparent integration of the ML model into Electronic Health Records (EHR) drives standardized assessments, leading to significant improvements in patient care.
Stanford Health leverages AI to mitigate clinical deterioration events, employing a machine learning model that refreshes predictions on hospitalized patients every 15 minutes. This model serves as an impartial evaluator of risk, aiding in harmonizing and streamlining patient care within an AI-infused framework.
The early identification of patient clinical deterioration holds immense potential for reducing mortality rates and enhancing overall outcomes, yet it poses a significant challenge across hospital and ambulatory settings.
Stanford Health Care has incorporated validated artificial intelligence and machine learning models into its clinical decision support systems to combat this challenge. Moreover, they’ve seamlessly integrated AI into clinical workflows, enhancing the patient experience. This integration has reduced wait times, improved care quality, and facilitated crucial conversations.
Dr. Shreya Shah, an esteemed academic internist and board-certified clinical informatics practitioner at Stanford Health Care, is a leading expert in the integration of artificial intelligence in healthcare. She is set to present Stanford Health’s AI initiatives at the upcoming 2023 HIMSS AI in Healthcare Forum, where she’ll share a compelling case study titled “How Stanford Health Transformed Patient Care by Combining Compassion with AI-Driven Innovations.”
In a conversation with Dr. Shah, she provided insight into the institution’s application of AI and ML:
Q: What makes detecting clinical deterioration in patients such a challenge?
A: Patients in hospitals are increasingly complex, with higher severity of illness, while lower acuity care shifts to outpatient settings or home care. Within academic medical centers, this complexity is magnified, with patients at high risk for clinical deterioration. Early signs can be subtle and vary widely among patients, making it akin to finding a needle in a haystack. This complexity, coupled with multi-person care teams managing vast amounts of evolving data, often leads to communication gaps, information overload, and cognitive biases. This can result in unforeseen clinical deterioration, necessitating emergency interventions or unplanned ICU transfers.
Standardized workflows that empower all team members in patient care decisions could alleviate these challenges.
Q: What prompted the decision to employ AI and ML in addressing this challenge?
A: We needed a system to identify at-risk patients and align the care team around a standardized clinical response. Recognizing that an ML model could identify high-risk patients without burdening our clinicians with additional tasks, we opted for this approach. The emphasis was not solely on the accuracy of predictions but on triggering a structured collaborative workflow among care team members for flagged patients. Our implementation efforts centered on integrating the ML model into the healthcare system, establishing effective collaborative workflows, and deploying scalable AI-integrated systems aligned with clinical needs.
Q: Could you share an example of how AI and ML models helped with clinical deterioration?
A: Our clinical deterioration model, validated on our data, was integrated transparently into our Electronic Health Record (EHR) system, including contributing factors, and supplemented with mobile alerts to the care team. This model updates patient predictions every 15 minutes, serving as an impartial risk evaluator and aiding coordination in patient care. Following site-specific validation, this approach led to increased standardized patient assessments by multidisciplinary teams, resulting in a notable 20% reduction in clinical deterioration events. Qualitative assessments revealed that the model effectively aligned mental models across teams, guiding necessary workflows for flagged patients with consensus among multidisciplinary team members. This continuous risk signal alignment empowered physicians and the care team to enact consistent workflows for improved patient care.
Stanford Health’s AI integration proves instrumental in identifying and addressing clinical deterioration challenges. Transparently embedding ML models into EHR facilitates standardized patient assessments and aligns multidisciplinary teams. Dr. Shreya Shah’s insights underscore the significance of collaborative workflows triggered by AI predictions. By empowering care teams to implement consistent workflows, the initiative leads to a substantial reduction in clinical deterioration events. Stanford Health’s approach not only prioritizes accurate risk assessment but also drives cohesive and efficient patient care, marking a transformative leap in healthcare integration of AI for improved patient outcomes.