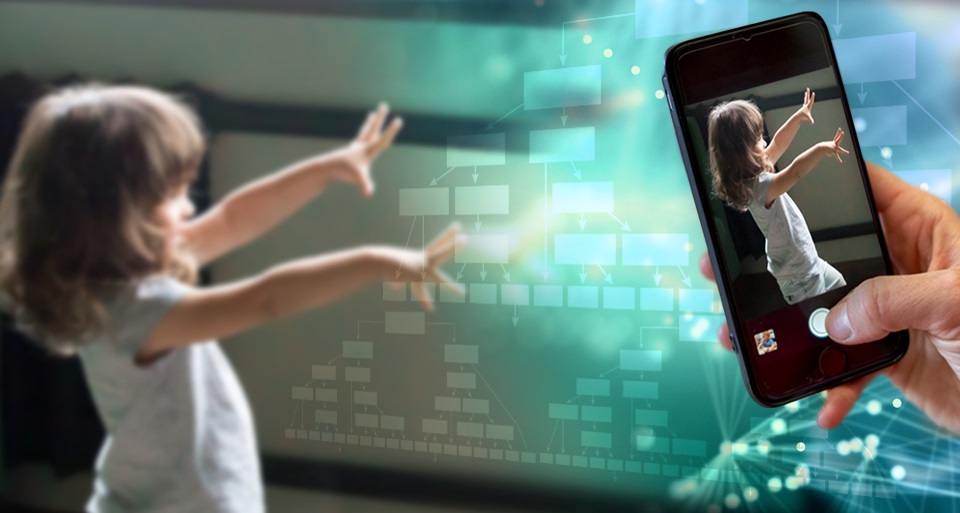
An innovative AI system, showcased at RSNA, accurately detects autism in 24 to 48-month-old children with a 98.5% success rate by analyzing specialized brain MRIs. Developed by a multi-disciplinary team led by Mohamed Khudri and Gregory N. Barnes, the AI interprets diffusion tensor MRI scans, identifying connectivity markers that reveal autism traits. This groundbreaking method offers a promising pathway for early intervention before age three, potentially enhancing long-term outcomes. Overcoming diagnostic delays highlighted by the CDC, this AI tool aims to streamline assessment, significantly reducing costs and time associated with diagnosis and treatment.
Presenting at RSNA, a pioneering AI system promises a transformative shift in early autism diagnosis. Developed by a team led by Mohamed Khudri and Gregory N. Barnes, this AI analyzes specialized MRIs to accurately identify autism in children aged 24 to 48 months with a remarkable 98.5% accuracy. By scrutinizing diffusion tensor MRI scans, the AI recognizes connectivity markers indicative of autism traits, presenting an unprecedented opportunity for early intervention. Addressing prevalent diagnostic delays outlined by the CDC, this breakthrough technology seeks to revolutionize pediatric care by expediting assessments, thereby reducing costs and facilitating timely interventions.
AI Breakthrough: Early Autism Diagnosis via Novel AI System
The upcoming annual meeting of the Radiological Society of North America (RSNA) will showcase a groundbreaking development in the field of autism diagnosis. A newly engineered artificial intelligence (AI) system, designed to scrutinize specialized MRIs of the brain, has demonstrated an extraordinary capability: it accurately identifies autism in children aged 24 to 48 months with an exceptional 98.5% accuracy rate.
The brains behind this innovation include Mohamed Khudri, B.Sc., a visiting research scholar at the University of Louisville, and a multi-disciplinary team. This team conceived a three-stage AI system tailored to analyze and categorize diffusion tensor MRI (DT-MRI) scans. DT-MRI, a specialized technique, tracks the movement of water along white matter tracts within the brain.
Khudri elaborated, “Our algorithm is trained to pinpoint deviations that indicate whether an individual is autistic or neurotypical.”
This sophisticated AI methodology involves extracting brain tissue images from DT-MRI scans and identifying imaging markers indicative of the connectivity between various brain regions. A machine learning algorithm then compares these marker patterns in the brains of children with autism to those in typically developed brains.
Co-author Gregory N. Barnes, M.D., Ph.D., who serves as a professor of neurology and directs the Norton Children’s Autism Center in Louisville, explained, “Autism predominantly manifests as improper connections within the brain. DT-MRI captures these abnormal connections, which underlie the symptoms commonly observed in children with autism, such as impaired social communication and repetitive behaviors.”
To test the system’s efficacy, researchers examined DT-MRI brain scans from 226 children between 24 and 48 months old, sourced from the Autism Brain Imaging Data Exchange-II. This dataset encompassed scans of 126 autistic children and 100 typically developing children. The AI technology exhibited remarkable metrics: 97% sensitivity, 98% specificity, and an overall accuracy rate of 98.5% in identifying children with autism.
Khudri emphasized, “Our approach represents a groundbreaking leap in enabling the early detection of autism in infants under two years of age. We strongly believe that intervening therapeutically before the age of three can yield better outcomes, potentially enhancing independence and raising IQ levels among individuals with autism.”
However, prevailing statistics, such as those from the CDC’s 2023 Community Report on Autism, reveal concerning gaps. Fewer than half of children diagnosed with autism spectrum disorder undergo developmental evaluation by the age of three, and 30% of those meeting the criteria for autism spectrum disorder remain undiagnosed until they are 8 years old.
Dr. Barnes elucidated, “The rationale behind early intervention hinges on leveraging brain plasticity, the brain’s capacity to reorganize and normalize function through therapy.”
The delay in diagnosing infants and young children with autism stems from various factors, including limited capacity at testing centers. Khudri highlighted that their AI system could streamline precise autism management, simultaneously cutting down the time and expenses associated with assessment and treatment.
Dr. Barnes projected, “Imaging holds the promise of swiftly and objectively detecting autism. We envision an autism assessment protocol that begins with DT-MRI, followed by a condensed session with a psychologist to confirm the results and guide parents on subsequent steps. This streamlined process could potentially reduce psychologists’ workload by up to 30%.”
This AI system generates a comprehensive report delineating the affected neural pathways, their anticipated impact on brain function, and a severity grade that can direct early therapeutic interventions.
The researchers are actively working on commercializing their AI software and obtaining FDA clearance, anticipating a transformative impact on early autism diagnosis and intervention.