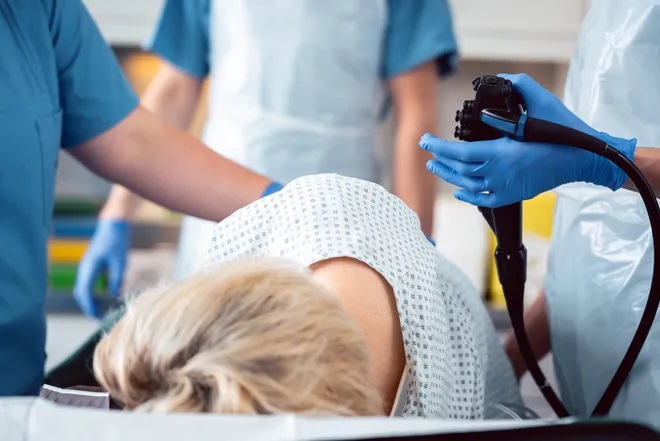
AI-aided colonoscopy shows promise in bolstering colorectal neoplasia detection, as per a recent meta-analysis. Evaluated against standard procedures, AI integration significantly reduces adenoma miss rates (AMRs) and enhances adenoma detection rates (ADRs). Findings reveal a marked increase in polyp and adenoma detection, benefiting specific patient cohorts. Despite positive outcomes, further investigation is vital to gauge long-term efficacy and cost-effectiveness.
Recent developments in medical technology have unveiled promising avenues for enhancing colorectal neoplasia detection through the aid of artificial intelligence (AI). A systematic review and meta-analysis of randomized clinical trials shed light on the potential benefits of AI-aided colonoscopy, presenting an encouraging path forward in medical diagnostics. Published in eClinicalMedicine, this research evaluated the efficacy of AI-integrated systems during colonoscopies, aiming to assess their impact on detecting colorectal neoplasia.
AI-Driven Colonoscopy: Enhancing Neoplasia Detection
The study delved into the advantages and drawbacks of leveraging AI technology to aid in the detection of colorectal neoplasia, emphasizing its potential to improve adenoma detection rates (ADRs) and reduce adenoma miss rates (AMRs). However, the findings across various studies displayed mixed results, underscoring the need for further investigation into this evolving field.
Employing Medical Subject Headings (MeSH) terms and keywords, researchers conducted an extensive electronic literature search across prominent databases such as Embase, Medline, and the Cochrane Library. The objective was to identify relevant randomized controlled trials conducted until October 4, 2023, comparing AI-assisted colonoscopy with the standard procedure for detecting colorectal neoplasia.
The primary outcomes scrutinized included AMR, ADR, and adenomas detected per colonoscopy (APC). Secondary outcomes comprised the polyp missed detection rate (PMR), polyp detection rate (PDR), and polyps detected per colonoscopy (PPC).
A comprehensive analysis encompassing 33 RCTs involving 27,404 patients revealed substantial findings. Patients undergoing AI-aided colonoscopy exhibited a notable decrease in PMR and AMR by 52.5 percent and 50.5 percent, respectively, in contrast to those undergoing standard colonoscopy procedures.
Moreover, the AI-aided colonoscopy group displayed a significant increase in PDR, ADR, PPC, and APC rates. A remarkable relative increase of 24.2 percent in ADR and 23.8 percent in PDR was observed, accompanied by a 39 percent increase in APC and a 38.8 percent increase in PPC.
These enhancements resulted in the identification of an average of 0.271 more PPCs and 0.202 more APCs, albeit with a 20-second increase in mean inspection time. The study emphasized specific beneficiary groups, including younger patients with lower body mass index (BMI) and endoscopists with lower ADR or PDR and shorter inspection times.
Moreover, factors like the time of day, anesthesia, and bowel preparation significantly influenced the efficacy of AI-aided colonoscopy, as discerned from the reviewed RCTs. The study concluded that AI-aided colonoscopy holds promise in significantly improving the detection of patients with advanced adenomas, particularly those with non-neoplastic lesions. Furthermore, it could enhance surveillance strategies for small adenomas, potentially reducing the risk of interval colorectal cancer (CRC).
Despite these encouraging findings, the research team stressed the necessity for additional studies to evaluate the cost-effectiveness and long-term benefits of employing AI-aided colonoscopy to curtail cancer incidence.
In a parallel realm of research, studies led by the Mayo Clinic demonstrated the potential of AI, particularly deep learning (DL) frameworks, in predicting recurrence and survival in CRC patients. These advancements aim to address the challenges in predicting cancer recurrence using tumor images, thereby assisting clinicians in determining appropriate treatment plans.
The DL model showcased high predictive performance, accurately discerning patients requiring intensive treatment and those who might not, thus holding promise for improving patient outcomes and survival rates in CRC care.
AI integration in colonoscopy signifies a breakthrough in improving colorectal neoplasia detection. Notable reductions in AMRs and increases in ADRs indicate their promising potential, especially for specific patient groups. Yet, the need for extensive exploration into its cost-effectiveness and long-term benefits remains crucial for widespread implementation in clinical settings. Continued research will elucidate AI’s role in transforming colorectal cancer care.