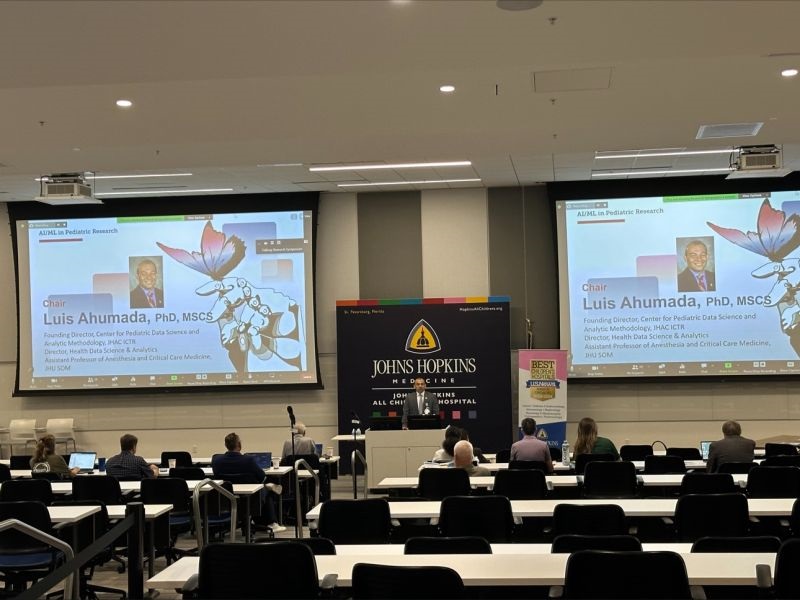
Dr. Ahumada, John Hopkins’ Health Data Science Director, shares invaluable insights at HIMSS AI in Healthcare Forum. He delineates AI’s role in clinical workflows, emphasizing AI’s ongoing evolution. Ahumada highlights their emphasis on improving clinical documentation and data quality while facing challenges of data integrity and accessibility. His discussion encompasses diverse AI use cases, stressing the importance of data integrity as the linchpin for AI success. However, he acknowledges the cost barriers, underlining the need for equity in AI accessibility across healthcare systems.
Dr. Luis Ahumada, the Director of Health Data Science and Analytics at Johns Hopkins All Children’s Hospital, is set to share his experiences and insights on effective AI deployment within clinical settings at the upcoming HIMSS AI in Healthcare Forum in San Diego. With a focus on practical use cases, Ahumada aims to shed light on the nuances, challenges, and best practices for integrating AI into healthcare systems.
Ahumada’s journey as an early adopter of AI and machine learning models has been centered on leveraging these technologies to enhance clinical workflows and optimize care delivery processes. He emphasizes the importance of aligning advanced data analytics models with clinical workflows to achieve seamless integration and effectiveness.
John Hopkins has seen significant strides in using AI-enabled tools to streamline clinical diagnostics, automate tasks, and facilitate better patient care. Ahumada highlights the ongoing nature of their AI initiatives, acknowledging that while AI has proven beneficial, it remains an evolving project demanding continuous attention and refinement.
The hospital’s approach to AI involves recognizing the value of different types of AI, each with distinct characteristics. Ahumada delineates two essential categories: Language Model Models (LLMs) and traditional machine learning. While acknowledging the potential, he emphasizes the hospital’s historical focus on traditional machine learning for predictive models and risk calculators.
Within their realm of use cases, John Hopkins All Children’s Hospital places a keen emphasis on enhancing clinical documentation. High-risk calculators for various medical scenarios have been developed, yet Ahumada points out that inefficiencies in the documentation process often underlie many problems, necessitating resolution as a priority.
Improving data quality is a critical facet of their AI initiatives. Ahumada emphasizes the significance of acquiring superior data through various means, including machine learning and LLMs. However, he acknowledges the challenges posed by missing data, validation, and data integrity issues that plague the healthcare data landscape.
One of the primary hurdles encountered is the difficulty in aggregating vast amounts of data required for effective traditional machine learning. Despite these challenges, Johns Hopkins remains committed to advancing various AI and machine learning use cases, seeking solutions to these obstacles and harnessing the potential of generative AI technologies.
Ahumada emphasizes the pivotal role of data integrity, considering it the foundation of their AI and machine learning endeavors. However, he underscores concerns about the costs of implementing AI solutions, acknowledging that accessibility to such technologies remains a challenge, particularly for smaller healthcare systems with limited resources.
Ahumada stresses the disparity in AI adoption between larger and smaller health systems, citing cost as a significant barrier. He highlights the necessity for specialized expertise in deploying AI tools and acknowledges the financial implications involved in acquiring and maintaining such talent.
The narrative extends to the broader landscape of AI in healthcare, raising pertinent questions about equity in access to AI technologies. Ahumada points out that while AI presents numerous benefits, its implementation comes at a cost that may hinder its accessibility to all healthcare systems.
Dr. Luis Ahumada’s insights provide a comprehensive perspective on the complexities surrounding AI deployment in healthcare. His experiences underscore the need for ongoing efforts to improve data quality, address challenges in integration, and make AI technologies more accessible across diverse healthcare settings.
Dr. Ahumada’s discourse elucidates the multifaceted landscape of AI deployment in healthcare. Despite AI’s transformative potential in streamlining clinical processes, challenges regarding data integrity and accessibility persist. He stresses the need for ongoing efforts to enhance data quality, overcome cost barriers, and ensure equitable AI access for all healthcare systems. Ahumada’s insights underscore the imperative of continuous refinement and integration of AI technologies to optimize healthcare delivery while acknowledging the complexities and hurdles inherent in their implementation.