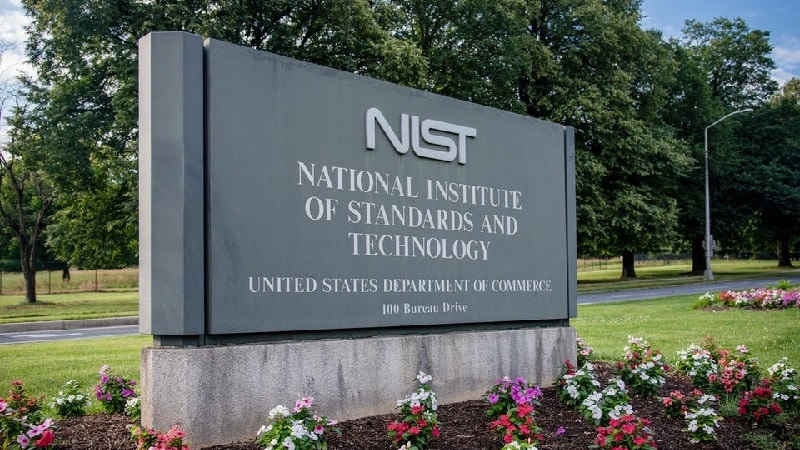
NIST’s Draft Guidance on Differential Privacy navigates data release while safeguarding individual identities. This framework, responding to Biden’s AI directive, aims to balance privacy and accuracy. Highlighting the necessity for standardized practices, NIST’s draft bridges the gap for users. It illustrates scenarios where data benefits society while preserving individual privacy. The publication guides federal agencies, developers, and policymakers, streamlining the understanding and assessment of differential privacy claims. Despite its maturity, the framework acknowledges evolving challenges. Public comments are invited until 2024, emphasizing the ongoing refinement process.
The National Institute of Standards and Technology (NIST) responds to the call for privacy protection in AI-driven research via its Differential Privacy Guidance. Aligned with the Biden Administration’s AI directives, this framework aims to harmonize data utility and individual privacy. It tackles the lack of standardized practices, offering a roadmap for evaluating differential privacy. With practical scenarios, it elucidates the challenge of data utilization while ensuring individual anonymity. Intended for federal bodies, developers, and policymakers, it seeks to demystify differential privacy claims. However, it acknowledges the evolving nature of this field and invites public feedback for further refinement.
Under the Biden Administration’s Executive Order on AI Offers, the U.S. National Institute of Standards and Technology unveiled preliminary guidelines for evaluating data privacy protection concerning artificial intelligence (AI) applications. This draft guidance aims to strike a balance between privacy and accuracy for organizations centered around data.
Differential privacy, a well-established privacy-enhancing technology in data analytics, lacks standardized practices, posing challenges to its effective implementation, as highlighted by NIST in its recent announcement. The agency envisions its new guidelines on differential privacy guarantees as instrumental in enabling users to navigate this intricate landscape.
In illustrating a practical scenario, NIST posed a hypothetical predicament: health researchers seeking access to consumer fitness tracker data to enhance medical diagnostics. The challenge, as NIST outlines, is obtaining valuable and precise information for societal benefit while preserving individual privacy.
While the draft guidance, named Draft NIST Special Publication (SP) 800-226, Guidelines for Evaluating Differential Privacy Guarantees, is directed towards federal agencies in compliance with the executive order, it is a valuable resource for software developers, business owners, and policymakers. It intends to foster a clearer understanding and consistent evaluation of claims associated with differential privacy.
The genesis of this algorithm stems from the Privacy-Enhancing Technologies Prize Challenge, a joint initiative between the U.S. and the U.K. that allocated a $1.6 million prize pool for leveraging federated learning to create innovative cryptography, ensuring data encryption during AI model training. These Privacy-Enhancing Technologies (PETs) have diverse applications, ranging from novel cryptography to combating money laundering and predicting public health emergencies. Over 70 solutions underwent rigorous testing via Red Team attacks to gauge their ability to safeguard raw data.
Arati Prabhakar, assistant to the President for science and technology and director of the White House Office of Science and Technology Policy, emphasized the pivotal role of privacy-enhancing technologies in balancing data value and individual privacy in a White House announcement earlier.
Despite its maturity, differential privacy, which introduces noise through central or multiple aggregators, is still evolving, according to Damien Desfontaines, a staff scientist at Tumult Labs specializing in differential privacy.
Naomi Lefkovitz, the manager of NIST’s Privacy Engineering Program and an editor on the draft, highlighted associated risks. She stressed the publication’s role in aiding organizations to evaluate differential privacy products and validate the accuracy of claims made by their creators.
Lefkovitz elucidated that while differential privacy is currently the most robust method for post-training privacy protection, it doesn’t offer absolute immunity against all types of attacks. Developers must critically evaluate real-world privacy assurances, considering various factors outlined in NIST’s “differential privacy pyramid.” This pyramid categorizes factors affecting privacy guarantees into top-level direct measures, middle-level undermining factors, and bottom-level underlying aspects like the data collection process.
Public comments on the draft are invited until January 25, 2024, with the final version anticipated to be published later in the same year.
In a broader context, the rise of powerful AI models facilitated by quantum computing presents an expanded attack surface for organizations managing substantial datasets, particularly in sensitive domains like healthcare. NIST has recently issued draft algorithms for quantum-resistant cryptography, seeking feedback on standards aimed at withstanding cyberattacks empowered by quantum computing.
Dan Draper, founder and CEO of CipherStash, emphasized the imperative nature of protecting data reliant on public key cryptography in the face of advancing quantum computing capabilities. He highlighted the urgency for organizations to update their software swiftly, akin to the Y2K challenge.
NIST’s Draft Guidance on Differential Privacy marks a significant stride in reconciling data utility and privacy in AI research. Responding to governmental directives, it strives to establish standardized practices. The framework aims to assist federal agencies, developers, and policymakers in comprehending and evaluating differential privacy claims. Although acknowledging the ongoing evolution of this field, it underlines the importance of ensuring data utility while safeguarding individual identities. Open for public input until 2024, this initiative signifies a commitment to refining and streamlining privacy practices in the realm of artificial intelligence.