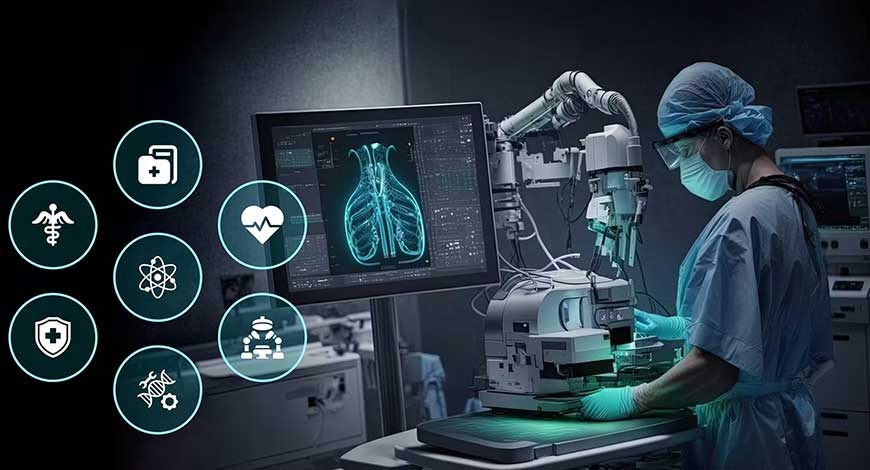
AI’s synergy with radiology reshapes healthcare by enhancing diagnostics, streamlining workflows, and amplifying precision. Evolving from early skepticism, AI, especially deep learning and radiomics, redefines diagnostics. AI’s integration spans the patient journey, optimizing processes and bolstering care. Despite challenges like standardization and data security, initiatives like RQS and TRIPOD ensure quality and reproducibility. Education and seminars spotlight AI’s role as a supportive tool. As debates persist, AI stands poised to bridge technology and healthcare, propelling transformative advancements in radiology for superior patient outcomes.
The amalgamation of AI and radiology marks a paradigm shift in healthcare. From John McCarthy’s foundational definition to AI’s contemporary prowess, the integration aims to replicate human intelligence in diagnostics. Innovations in deep learning and radiomics have redefined image analysis, optimizing accuracy and efficiency. AI permeates the patient journey, influencing scheduling, diagnostics, and care delivery. Challenges like standardization and privacy loom, addressed by quality evaluation tools. Education initiatives enlighten professionals on AI’s potential. Amidst debates, seminars advocate AI’s supportive role in patient care, heralding a transformative era in radiology.
Defining AI in Radiology
The concept of AI, initially coined by John McCarthy, delineates the science and engineering behind creating intelligent machines and computer programs. In its modern context, AI endeavors to enable machines to independently learn and reason, executing tasks that traditionally necessitate human intelligence—such as language comprehension, pattern recognition, problem-solving, and decision-making. This evolution has fostered a transformative impact on diverse industries, notably in healthcare sectors reliant on image processing, like radiology. AI’s integration within radiology practices has brought forth innovative tools that amplify healthcare professionals’ capabilities, optimize workflows, and significantly enhance diagnostic accuracy.
AI’s Evolution in Radiology
The inception of AI’s utilization in radiology dates back to the early ’90s, primarily in detecting microcalcifications in mammography through computer-aided detection (CAD). While CAD faced skepticism due to studies questioning its efficacy and impact on radiologists’ accuracy, the synergy of AI, particularly deep learning within CAD systems, has marked a pivotal shift. Reports from the Radiological Society of North America (RSNA) underscore how deep learning is essentially redefining CAD processes. By leveraging vast datasets, deep learning empowers computers to autonomously comprehend concepts, enabling the detection of pathology-associated findings in X-rays akin to seasoned radiologists’ proficiency.
The Emergence of Radiomics
Simultaneously, the emergence of radiomics, focusing on quantitative metric extraction from medical images, has unfolded as a burgeoning field. Radiomic features enable capturing nuanced tissue and lesion characteristics, facilitating clinical problem-solving when combined with demographic, histologic, genomic, and proteomic data. Radiologists can interpret digital images qualitatively, transforming them into invaluable quantitative data. Radiomics surmounts human visual limitations, highlighting subtle variations in image attributes like intensity, geometry, and texture. This enables early pathosis detection, aiding in treatment planning and regimen optimization.
AI’s Role in Patient Care
The comprehensive integration of AI into the patient journey spans scheduling appointments, selecting examinations, managing insurance transactions, ensuring imaging appropriateness, and optimizing reporting workflows. Over 500 AI-based medical devices have garnered authorization from the U.S. Food and Drug Administration (FDA), integrating into clinical decision support, image viewing, and medical record software systems, significantly enhancing patient care. Notably, AI-driven systems like BoneXpert for bone age estimation and AI software for detecting pulmonary metastases and tuberculosis on radiographs have remarkably reduced reading time and bolstered diagnostic accuracy.
Challenges and Solutions
Despite AI’s promising trajectory, the field encounters inherent challenges. Standardizing techniques, acquiring sufficiently large datasets, ensuring reproducibility, and accounting for various patient variables pose hurdles. Privacy concerns and data security also warrant attention as AI permeates healthcare. Initiatives like the Radiomics Quality Score (RQS) and the Transparent Reporting of a Multivariable Prediction Model for Individual Prognosis or Diagnosis (TRIPOD) checklist aim to address these challenges by evaluating study quality and predicting diagnostic effectiveness.
The Future of AI in Radiology
The potential of AI remains vastly untapped, prompting efforts to educate and enlighten industry professionals on harnessing AI’s full spectrum. Radiology education institutes offer specialized AI-oriented fellowships and training programs to equip technicians and clinicians to seamlessly integrate AI into their daily workflows. As debates persist about AI’s role—whether a bane or boon—seminars advocating AI’s role as a supportive tool in patient care continue to foster awareness and understanding.
AI’s integration propels radiology towards unparalleled advancements. Deep learning, radiomics, and AI-driven tools redefine diagnostics, enhancing accuracy and patient care across the continuum. Despite hurdles like standardization and data security, initiatives ensure quality and reproducibility. Education initiatives enlighten professionals on AI’s spectrum. Debates persist on AI’s role, yet seminars advocate its supportive nature in patient care. As AI bridges technology and healthcare, its judicious integration holds the key to unlocking transformative potentials, driving superior patient outcomes and revolutionizing radiology’s future.