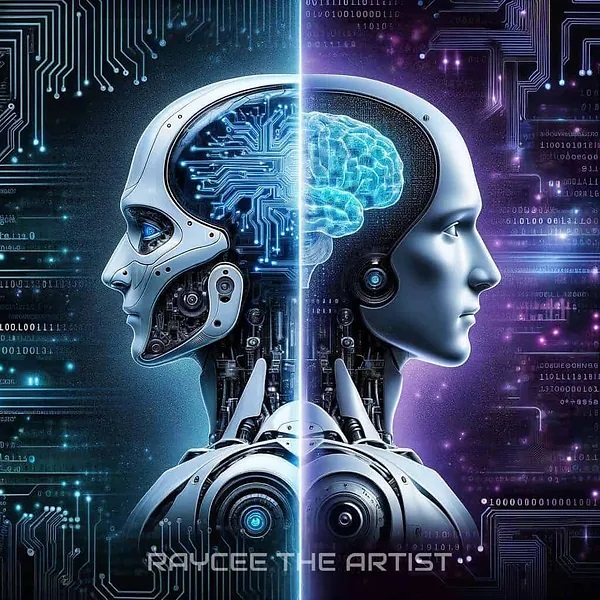
Artificial General Intelligence (AGI) is reshaping radiation oncology, boosting precision and efficiency. AGI models like GPT-4, PaLM 2, and LVMs such as SAM excel in auto-segmentation, treatment planning, and outcome prediction. Their few-shot and zero-shot learning capabilities yield adaptable models for radiotherapy stages. AGI’s fusion with vision data creates powerful multimodal models, paving the way for personalized therapy. While revolutionizing oncology, AGI emphasizes complementing human expertise.
Within radiation oncology, Artificial General Intelligence (AGI) marks a transformative phase. AGI models, like GPT-4 and PaLM 2, coupled with Large Vision Models (LVMs) such as the Segment Anything Model (SAM), exhibit prowess in processing clinical data. With strengths in few-shot and zero-shot learning, AGI promises robustness in creating adaptable AI models for radiotherapy. The amalgamation of vision data and LLMs produces intricate clinical pattern deciphering, advocating for a data-driven, personalized approach to treatment complementing human expertise.
Every year, cancer claims the lives of an estimated 600,000 individuals in the United States. While surgeries, chemotherapy, and immunotherapy stand as crucial treatments, radiotherapy has emerged as a standard and effective option for nearly 50-70 percent of cancer patients.
The process of radiotherapy encompasses six fundamental stages: initial consultation, simulation, treatment planning, treatment delivery, treatment verification, and patient follow-up. With the advent of Artificial Intelligence (AI), a profound transformation is underway within the realm of radiotherapy. AI technologies have demonstrated capabilities that achieve human-level accuracy in several critical facets of radiotherapy, including auto-segmentation, tumor staging, image registration, automatic treatment planning, quality assurance, and outcome prediction. This technological leap substantially enhances the accuracy, precision, and efficiency of radiation therapy procedures.
A recent review featured in the KeAi journal Meta-Radiology sheds light on pioneering AGI models like GPT-4 and PaLM 2, alongside Large Vision Models (LVMs) such as the Segment Anything Model (SAM). These AGI models exhibit remarkable proficiency in processing extensive texts and imaging data, respectively. What sets AGI apart is its advanced prowess in few-shot and zero-shot learning, paving the way for the development of highly robust and adaptable AI models. These models are pivotal for seamless integration into various aspects of radiation oncology.
The fusion of vision data with LLMs has led to the creation of powerful multimodal models capable of elucidating nuanced clinical patterns. Together, AGI stands poised to catalyze a paradigm shift towards data-driven, personalized radiation therapy. However, the authors emphasize that these models should complement human expertise and care, rather than replace it.
The findings presented in this review offer an insightful overview of how AGI stands to revolutionize radiation oncology, elevating the standards of patient care. A key revelation lies in AGI’s exceptional ability to harness multimodal clinical data at scale. Additionally, researchers delve into the potential future directions of AGI in radiation oncology, addressing the possible developments and bottlenecks in this burgeoning field. These advancements in clinical applications are poised to further enhance the effectiveness of radiation therapy, ultimately leading to more positive outcomes for cancer patients.
Artificial General Intelligence (AGI) is gradually reshaping the landscape of radiation oncology by introducing unprecedented levels of precision and efficiency. Its applications in auto-segmentation, tumor staging, image registration, automatic treatment planning, quality assurance, and outcome prediction mark a significant leap forward in the field. The remarkable capabilities of AGI models like GPT-4, PaLM 2, and Large Vision Models (LVMs) such as the Segment Anything Model (SAM) offer promise for the seamless integration of AI into the various stages of radiotherapy.
One of the defining attributes of AGI is its ability to engage in few-shot and zero-shot learning, enabling the creation of highly adaptable and robust AI models. These models, adept at processing extensive textual and imaging data, are instrumental in comprehensively transforming radiation oncology practices. Through the amalgamation of vision data with LLMs, AGI has birthed powerful multimodal models that decipher intricate clinical patterns, laying the groundwork for a data-driven, personalized approach to radiation therapy.
The authors of the review stress the importance of these models acting as complements to human expertise and care rather than replacements. This partnership between AI and human professionals is poised to elevate the standard of patient care in radiation oncology, promising a future where the precision and efficacy of radiation therapy reach new heights.
Artificial General Intelligence’s integration in radiation oncology heralds a promising future for cancer care. AGI’s capacity in processing multimodal clinical data and its robust learning abilities indicate a shift towards personalized, data-driven therapy. While AGI models like GPT-4 and PaLM 2 elevate precision in radiotherapy, it emphasizes partnering with human expertise rather than replacing it. The fusion of vision data with LLMs has unlocked potential for nuanced clinical insights. AGI’s responsible inclusion alongside human proficiency anticipates more precise, effective cancer treatments for improved patient outcomes.