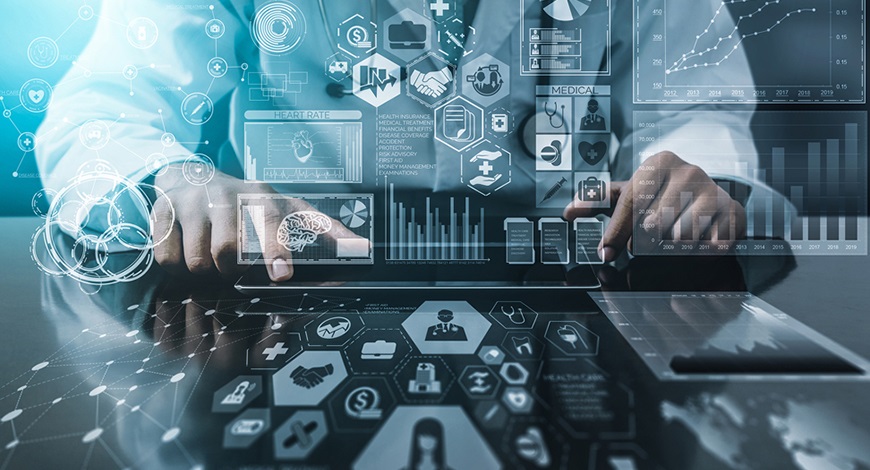
The University of Michigan’s JAMA-published research dissected AI’s role in diagnosing hospitalized patients. Across 13 states, clinicians evaluated biased AI algorithms, aiming to gauge their influence on diagnostic decisions. Surprisingly, disclosing AI workings failed to aid clinicians in detecting systemic biases. Nine patient vignettes were provided, leading to critical revelations. Standard AI models amplified diagnostic accuracy by 4.4% when coupled with explanations. However, exposure to systematically biased AI predictions led to an 11% accuracy decline, with explanations offering no shield. The study underscores the imperative to address AI biases, recognizing their detrimental impact on diagnostic precision in healthcare.
The JAMA-published study by the University of Michigan scrutinized the integration of artificial intelligence (AI) models in diagnosing hospitalized patients. Focused on comprehending the influence of AI bias, the research engaged clinicians from diverse backgrounds across 13 U.S. states. The central query revolved around whether disclosing AI model workings would assist clinicians in identifying and rectifying potential biases. A comprehensive survey involving nine clinical vignettes was conducted, revealing pivotal insights. While standard AI models showed promise by elevating diagnostic accuracy, the presence of systemic biases within these models led to substantial accuracy reductions. Furthermore, explanations accompanying biased AI predictions failed to counteract their adverse impact, raising concerns about AI’s reliability in clinical diagnoses.
The study involved a randomized clinical vignette survey conducted across 13 states, engaging hospitalist physicians, nurse practitioners (NPs), and physician assistants (PAs). Their focus was to systematically evaluate biased algorithms within image-based AI guides and how these influenced clinicians’ diagnostic judgments.
The investigation aimed to assess whether exposing clinicians to insights about the inner workings of AI models, including potential biases or limitations, could help them identify and rectify systematically biased algorithms. However, contrary to expectations, the study revealed that providing explanatory guides did not enable clinicians to recognize systematically biased AI models effectively.
Throughout the survey, participating clinicians were presented with nine clinical vignettes portraying patients hospitalized with acute respiratory failure. These vignettes included comprehensive details such as presenting symptoms, physical examination findings, laboratory results, and chest radiographs.
The clinicians were tasked with determining the likelihood of pneumonia, heart failure, or chronic obstructive pulmonary disease (COPD) as the underlying cause(s) for each patient’s acute respiratory failure based on the presented information.
The survey methodology involved presenting two vignettes without AI model input initially. Subsequently, the clinicians were randomly assigned to review six vignettes: three with AI model input, including both standard-model predictions and systematically biased model predictions. Importantly, half of these vignettes had accompanying AI model explanations.
The study’s outcomes revealed crucial insights. When clinicians reviewed patient vignettes with standard AI model predictions and accompanying explanations, diagnostic accuracy notably increased by 4.4% compared to the baseline accuracy.
Conversely, when clinicians were presented with vignettes featuring systematically biased AI model predictions, diagnostic accuracy decreased significantly by over 11%. Notably, the provision of model explanations did not safeguard against the adverse effects of inaccurate predictions stemming from systematically biased models.
The findings underscored that while standard AI models had the potential to enhance diagnostic accuracy, the presence of systematic bias within these models detrimentally impacted accuracy levels. Moreover, the commonly employed image-based AI model explanations failed to mitigate the deleterious effects of this bias on diagnostic accuracy.
This research sheds light on the complexities associated with integrating AI models into clinical decision-making processes. Despite the promise of AI in healthcare, the study highlights the critical need for addressing and rectifying systematic biases within these models, as their presence can substantially impair diagnostic accuracy.
JAMA’s University of Michigan-led investigation illuminated the intricate interplay between AI systems and diagnostic accuracy in healthcare. The study’s profound findings highlight the pressing need to confront and mitigate systematic biases embedded within AI models. Despite the potential of standard AI models to enhance diagnostic precision, the revelation of systemic biases unveiled their detrimental effect on accuracy levels. Alarmingly, commonly used AI model explanations proved ineffective in offsetting the adverse impacts of biased predictions. This study serves as a clarion call, emphasizing the imperative for robust strategies to identify, rectify, and prevent AI biases in healthcare. Addressing these concerns is critical to ensure the reliability and efficacy of AI-driven diagnostic tools for improved patient care.