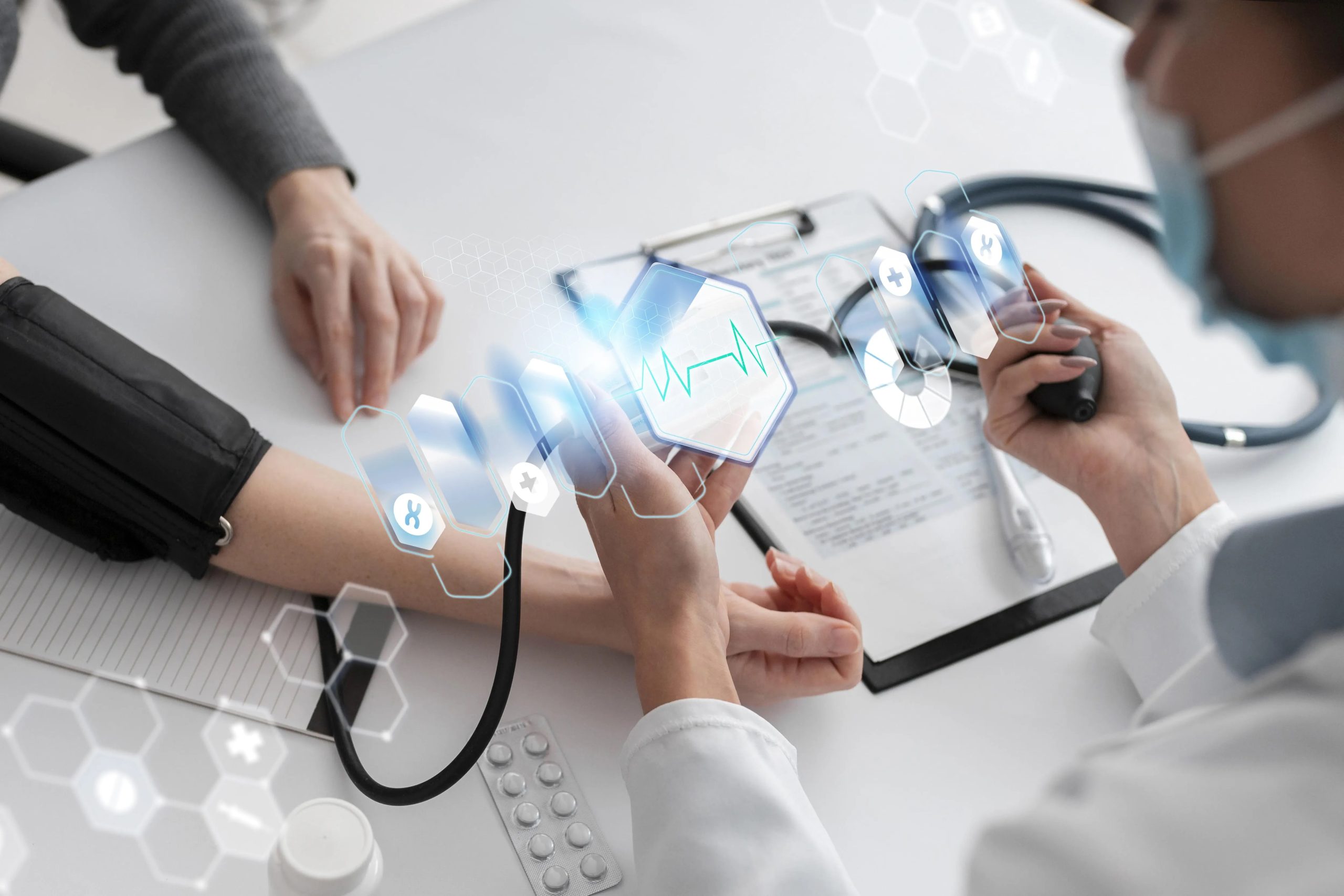
Eisai’s collaboration with Oita University heralds a milestone in Alzheimer’s research, presenting an innovative AI model utilizing wearable device data for prediction. The study’s comprehensive approach, encompassing both biological and lifestyle data, results in a predictive model demonstrating noteworthy accuracy in anticipating amyloid-beta protein accumulation. This breakthrough is crucial, providing a more accessible and cost-effective pre-screening tool for Alzheimer’s, addressing the limitations of traditional testing methods. The research aligns with the broader trend of leveraging AI and wearable technology for neurodegenerative disease detection, paving the way for transformative advancements in Alzheimer’s diagnostics.
Eisai, in collaboration with Oita University in Japan, has made significant strides in Alzheimer’s disease research by developing what could potentially be the world’s first artificial intelligence (AI) model utilizing data from wearable devices to predict the onset of Alzheimer’s disease, the most prevalent form of dementia. The groundbreaking findings of their research, aiming to create a cost-effective and practical tool for pre-screening individuals suspected of developing Alzheimer’s, were published in the journal Alzheimer’s Research & Therapy.
The study involved collecting biological and lifestyle data from 122 individuals aged 65 and older, all exhibiting mild cognitive impairment or subjective memory impairment. Wristband sensors were employed to gather biological data, encompassing physical activity, sleep patterns, and heart rate. Participants wore these sensors for seven days every three months from 2015 to 2019. Lifestyle data, including employment status, frequency of outdoor activities, means of transportation, community engagement, and background information such as age, education history, alcohol use, and medical history, were also obtained through medical consultations. Additionally, participants underwent annual amyloid PET (positron emission tomography) examinations, crucial for detecting the accumulation of amyloid-beta protein in the brain – a key biomarker associated with Alzheimer’s.
The research team harnessed three machine learning technologies—support vector machine, Elastic Net, and logistic regression—to develop a predictive model that integrated all the collected data. This model aimed to determine the likelihood of each participant testing positive for amyloid-beta protein accumulation through PET screening. The evaluation of the AI model demonstrated its “sufficient” capability to predict amyloid-beta protein accumulation, identifying 22 contributing factors, including physical activity, sleep, heart rate, conversation frequency, age, education length, living arrangements, means of transportation, presence of companions during hospital visits, communication patterns, and number of outings.
The significance of this research lies in addressing the imperative need for screening in Alzheimer’s, a currently incurable disease. Traditional testing methods, such as amyloid PET and cerebrospinal fluid (CSF) testing, are costly, limited, and invasive. Eisai and Oita University researchers recognize the potential of AI to make Alzheimer’s testing more accessible, especially in countries like Japan experiencing accelerated aging. While existing studies have employed AI to predict amyloid-beta protein accumulation, they primarily relied on cognitive function tests, blood tests, and brain imaging. Eisai and Oita University’s research, however, offers unique value by focusing on both lifestyle and biological data.
Building on their pioneering work, the research team envisions broader applications for their AI model in pre-screening individuals for Alzheimer’s, particularly those with limited access to conventional testing methods like amyloid PET and CSF testing. This approach aligns with the broader trend of utilizing wearable devices for Alzheimer’s research, as witnessed in initiatives such as Alzheimer’s Research UK’s 2020 project, backed by Bill Gates, to develop a wearable diagnostic device for early neurodegenerative disease detection.
In recent years, various projects worldwide have explored the integration of AI technology to enhance Alzheimer’s detection. Australia’s CSIRO and Queensland University of Technology introduced an AI-based benchmark for measuring brain atrophy, deemed a global first. Fujifilm developed an AI tool demonstrating accurate predictions of Alzheimer’s progression within two years. South Korea’s Lotte Healthcare and iMediSync collaborated to explore AI-driven healthcare services, including neuropsychiatric disorder screening. Concurrently, Eisai remains committed to Alzheimer’s research, evidenced by the launch of its digital health business, Theoria. Theoria, scheduled to launch in April, will unveil a flagship solution—a risk prediction algorithm for early detection of mild cognitive impairment.
Overall, Eisai and Oita University’s collaborative effort marks a significant leap forward in Alzheimer’s prediction, offering a pioneering solution through AI and wearable device integration. The identified factors contributing to amyloid-beta protein accumulation provide valuable insights. With potential applications extending beyond research, the developed AI model holds promise for widespread pre-screening, particularly benefiting individuals with limited access to conventional testing methods. This transformative approach signifies a critical stride towards managing the impact of Alzheimer’s, showcasing the potential of cutting-edge technology to revolutionize diagnostics and enhance accessibility in the field of neurodegenerative diseases.