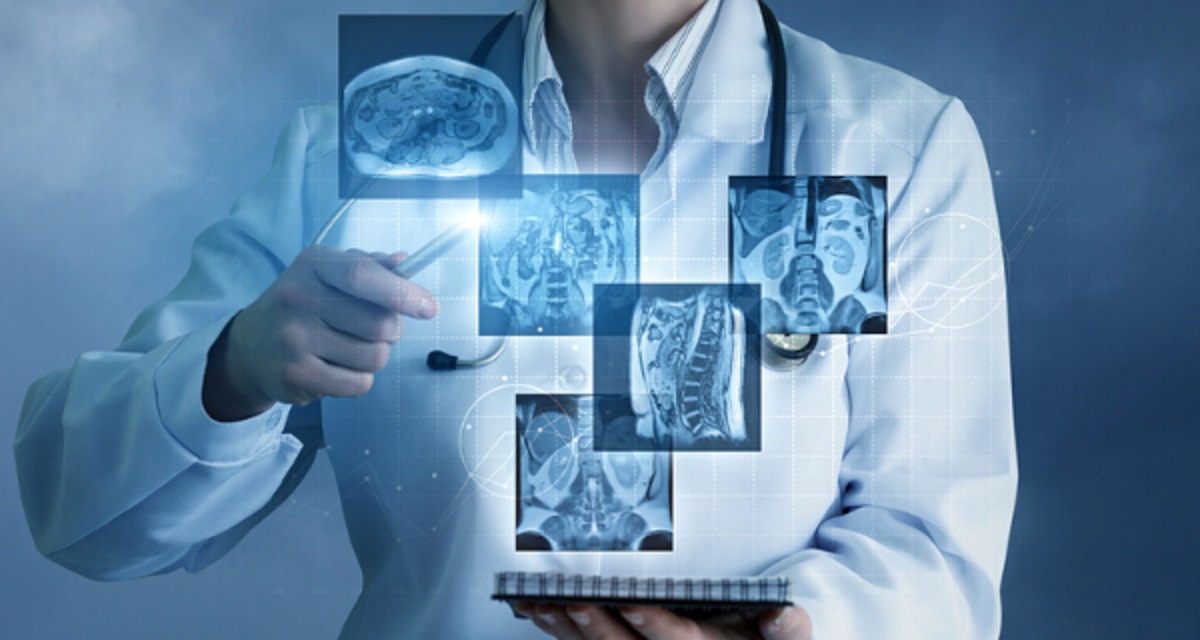
AI is reshaping radiology, from early CAD to advanced radiomics. Deep learning enhances diagnostic accuracy, while NLP and LLM revolutionize reporting and patient care. Challenges like standardization and privacy persist, met with solutions like RQS and TRIPOD. Education is key to maximizing AI’s potential, with institutes offering tailored programs. The debate on AI’s role in patient care continues, emphasizing its collaborative nature with clinicians.
Artificial Intelligence (AI) is ushering in a transformative era in healthcare by revolutionizing the capabilities of medical professionals and streamlining diagnostic processes. Radiology, a field heavily reliant on image processing, has been at the forefront of integrating AI tools to enhance diagnostic accuracy and improve workflow efficiency. The evolution of AI in radiology, from early attempts in computer-aided detection (CAD) to the emerging field of radiomics, demonstrates the potential for AI to reshape how diseases are diagnosed and treated.
The Evolution of AI in Radiology
The roots of AI in radiology trace back to 1992 with the introduction of CAD for detecting microcalcifications in mammography. Over the years, CAD faced skepticism due to reduced radiologist accuracy and a perceived lack of patient benefits. However, recent advancements, particularly in deep learning—a subset of machine learning (ML)—have revitalized the role of AI in radiology. The Radiological Society of North America (RSNA) reports that deep learning is “re-inventing” CAD by enhancing efficiency and minimizing false alarms. Deep learning employs neural networks to detect patterns within medical images, rivaling the diagnostic capabilities of practicing radiologists.
Radiomics: A Quantitative Approach
Radiomics, a burgeoning field, focuses on extracting quantitative metrics from medical images. These radiomic features provide valuable insights into tissue and lesion characteristics, complementing demographic, histologic, genomic, and proteomic data. Radiologists can translate qualitative interpretations of digital images into quantitative data, offering an additional tool for diagnostic and prognostic decision-making. Radiomics aids in overcoming the limitations of the human eye by highlighting subtle differences in image intensity, geometry, and texture.
Harnessing Natural Language Processing (NLP)
AI’s impact extends beyond image analysis to the realm of natural language processing (NLP). In radiology, statistical NLP has become a powerful tool for analyzing reports and research. Understanding the potential and limitations of NLP equips radiologists to leverage this technology for improved clinical workflows and extensive research analysis. Large language modeling (LLM), a deep learning algorithm, further enhances NLP capabilities, transforming treatment development through applications like report creation, speech recognition, and text classification.
AI’s Influence on Patient Care
AI’s integration spans the entire patient journey, impacting appointment scheduling, examination selection, insurance transactions, imaging appropriateness, and reporting workflows. With over 500 FDA-authorized AI-based medical devices, clinical decision support software, image viewing software, and medical record systems now incorporate various AI tools. AI-driven bone age estimation systems and software for detecting pulmonary metastases and tuberculosis showcase substantial reductions in reading time, enhancing overall patient care.
Emerging Challenges and Solutions
Despite the promising potential, AI in radiology faces challenges in technique standardization, limited datasets, and reproducibility issues. Addressing these challenges requires the implementation of guidelines such as the Radiomics Quality Score (RQS) and the Transparent Reporting of a Multivariable Prediction Model for Individual Prognosis or Diagnosis (TRIPOD) checklist. These tools assess the quality of radiomics studies and reports, promoting transparency and standardization within the field.
Privacy and data security concerns also loom large as AI becomes more widespread in healthcare. Industry-wide efforts to establish robust privacy measures and ensure secure data handling are imperative for building trust in AI applications.
The Role of Education in Maximizing AI Potential
To fully harness the capabilities of AI in radiology, there is a need for education and training programs. Radiology education institutes offer AI-oriented fellowships and training to empower technicians and clinicians to seamlessly integrate AI into their daily workflows. Seminars and discussions are essential for raising awareness about AI’s role as a valuable tool, emphasizing its role in assisting rather than replacing clinicians in patient care.
The integration of AI into radiology marks a significant shift in how diseases are diagnosed and treated. From the evolution of CAD to the emergence of radiomics and the utilization of NLP and LLM, AI is expanding the horizons of diagnostic capabilities. While challenges persist, guidelines and educational initiatives aim to address them, ensuring the responsible and effective integration of AI in radiology. As the debate about AI’s impact continues, its role as a valuable ally in enhancing patient care becomes increasingly evident.