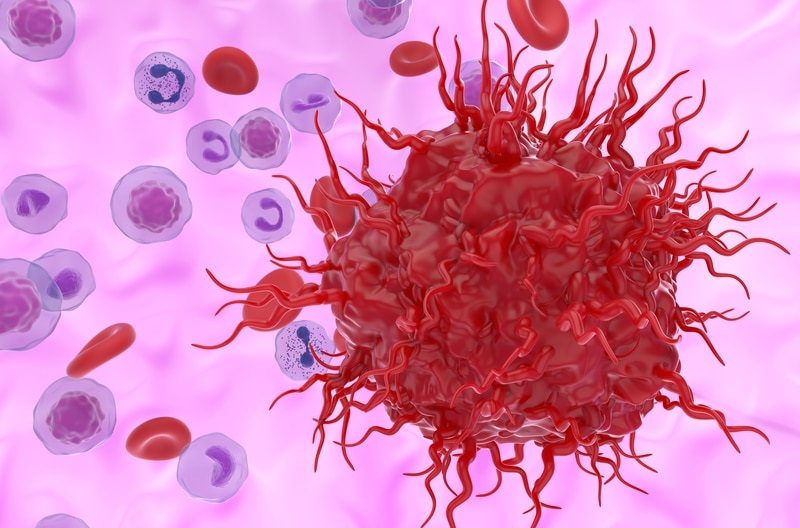
The Tsukuba team’s innovative CT-based radiomics deep learning model exhibits an 89% accuracy in predicting lymph node metastasis in nonfunctional pancreatic neuroendocrine tumors (NF-PNETs). Validated with external hospital data, the model maintains a remarkable 91% success rate across varied tumor sizes, offering a reliable tool for surgeons in treatment decision-making. Beyond revolutionizing NF-PNET treatment, the model contributes to personalized medicine, improving patient outcomes by guiding optimal surgical procedures and treatment strategies. Continuous refinement and collaboration hold the promise of further advancements in precision medicine for NF-PNETs.
Nonfunctional pancreatic neuroendocrine tumors (NF-PNETs), though rare, pose a significant challenge in the medical field, primarily being treated through surgical interventions. The decision-making process regarding surgery is intricately linked to the presence or absence of lymph node metastasis. Particularly contentious is the necessity of surgery for tumors smaller than 2 cm, as current clinical guidelines lack a clear consensus on this matter. Conventional methods for preoperative diagnosis of lymph node metastasis have proven to be inadequate, necessitating innovative solutions to enhance diagnostic accuracy.
Addressing the Challenge:
In response to this pressing challenge, the Tsukuba team has pioneered a groundbreaking approach. They have developed a predictive model that leverages radiomics features extracted from computed tomography (CT) and magnetic resonance imaging (MRI) scans using state-of-the-art artificial intelligence deep-learning techniques. The objective is to enhance the accuracy of predicting lymph node metastasis and provide a valuable tool for surgeons to make informed decisions about the most appropriate treatment strategies.
Model Development and Validation:
The Tsukuba team’s predictive model has exhibited remarkable success, achieving an 89% accuracy rate in predicting lymph node metastasis. Even more compelling is its robust performance when validated with data from an external hospital, where the success rate soars to an impressive 91%. This underscores the model’s generalizability and reliability across diverse clinical settings.
Importantly, the model’s predictive power remains consistent, regardless of the tumor size, addressing the ongoing debate regarding the necessity of surgery for tumors smaller than 2 cm. The ability of the model to maintain its efficacy across varied tumor sizes is a testament to its potential to revolutionize the decision-making process for surgeons faced with challenging cases.
Clinical Significance:
The implications of this predictive model are profound. Surgeons now have a powerful tool at their disposal, assisting them in navigating the complexities of NF-PNET treatment. The model’s accuracy in predicting lymph node metastasis enables surgeons to make more informed decisions about the necessity of surgery and select the most appropriate treatment approaches.
The transformative potential of this model lies not only in its predictive capabilities but also in its ability to contribute to personalized medicine. Tailoring treatment strategies based on the individual characteristics of each case becomes more feasible with the integration of advanced technologies like CT-based radiomics deep learning.
Beyond providing a higher level of precision in decision-making, the model offers a tangible means to improve patient outcomes. By aiding in the selection of optimal surgical procedures and treatment strategies, the model has the potential to enhance the overall quality of care for individuals with NF-PNETs. This is particularly significant in a medical landscape where treatment decisions often hinge on the delicate balance between the invasiveness of surgery and the potential benefits for the patient.
Challenges and Future Directions:
While the Tsukuba team’s model represents a significant advancement, challenges and opportunities for refinement remain. Continuous validation and refinement of the model with larger and more diverse datasets will be essential to enhance its robustness and generalizability further. Additionally, the integration of multi-modal imaging data and the exploration of additional radiomics features may contribute to a more comprehensive and nuanced understanding of NF-PNETs.
Furthermore, collaboration among medical institutions and research centers is crucial for the collective advancement of predictive models in the field. The sharing of data and insights can facilitate the development of more sophisticated algorithms and foster a collaborative environment where breakthroughs can be accelerated.
Overall, the Tsukuba team’s pioneering work in CT-based radiomics deep learning represents a significant leap forward in predicting lymph node metastasis in nonfunctional pancreatic neuroendocrine tumors. The model’s high accuracy, validated across diverse clinical settings, positions it as a transformative tool for surgeons grappling with treatment decisions for NF-PNETs.
The model’s potential to revolutionize patient outcomes by aiding in the selection of optimal surgical procedures and treatment strategies cannot be overstated. As the medical community continues to embrace the era of personalized medicine, innovative technologies like CT-based radiomics deep learning are at the forefront, shaping a future where treatment decisions are tailored to the unique characteristics of each patient.
While challenges persist, the ongoing refinement of predictive models and collaborative efforts within the medical community holds the promise of even greater advancements. The journey towards precision medicine in the treatment of NF-PNETs is well underway, and the Tsukuba team’s work stands as a beacon of progress in this transformative journey.